Leveraging Frequency Domain Learning in 3D Vessel Segmentation
2023 IEEE International Conference on Bioinformatics and Biomedicine (BIBM)(2024)
摘要
Coronary microvascular disease constitutes a substantial risk to human health. Employing computer-aided analysis and diagnostic systems, medical professionals can intervene early in disease progression, with 3D vessel segmentation serving as a crucial component. Nevertheless, conventional U-Net architectures tend to yield incoherent and imprecise segmentation outcomes, particularly for small vessel structures. While models with attention mechanisms, such as Transformers and large convolutional kernels, demonstrate superior performance, their extensive computational demands during training and inference lead to increased time complexity. In this study, we leverage Fourier domain learning as a substitute for multi-scale convolutional kernels in 3D hierarchical segmentation models, which can reduce computational expenses while preserving global receptive fields within the network. Furthermore, a zero-parameter frequency domain fusion method is designed to improve the skip connections in U-Net architecture. Experimental results on a public dataset and an in-house dataset indicate that our novel Fourier transformation-based network achieves remarkable dice performance (84.37\% on ASACA500 and 80.32\% on ImageCAS) in tubular vessel segmentation tasks and substantially reduces computational requirements without compromising global receptive fields.
更多查看译文
关键词
coronary segmentation,discrete fourier transform,global receptive field
AI 理解论文
溯源树
样例
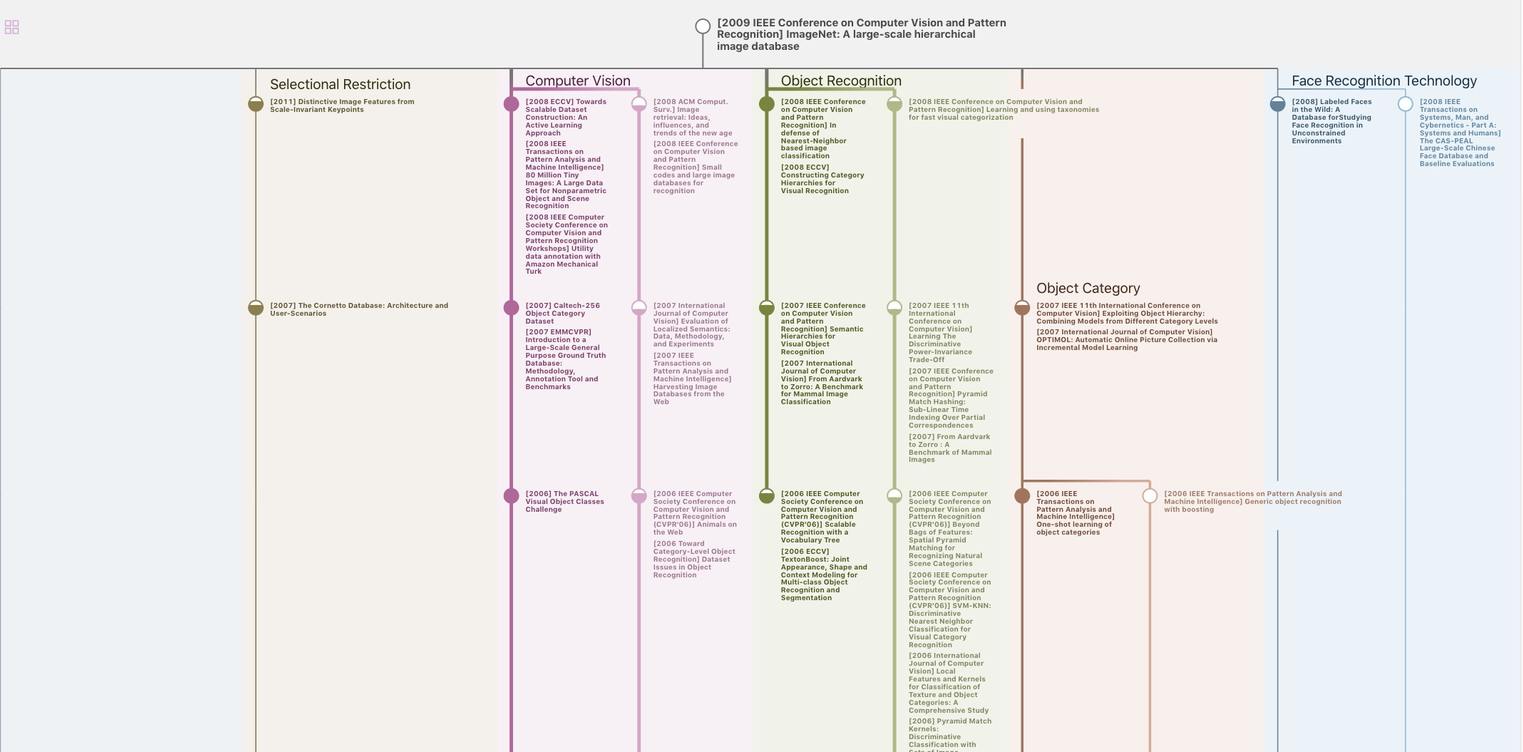
生成溯源树,研究论文发展脉络
Chat Paper
正在生成论文摘要