DSTGCS: an intelligent dynamic spatial–temporal graph convolutional system for traffic flow prediction in ITS
Soft Computing(2024)
摘要
Accurate traffic prediction is indispensable for relieving traffic congestion and people’s daily trips. Nevertheless, accurate traffic flow prediction is still challenging due to the traffic network’s complex and dynamic spatial and temporal dependencies. Most existing methods usually ignore the dynamicity of spatial dependencies or have limitations, as using the self-attention mechanism for capturing dynamic spatial dependencies is computation forbidden in large networks. In addition, there are both short- and long-range dynamic temporal dependencies, which are not well captured. To overcome these limitations, we propose an intelligent dynamic spatial and temporal graph convolutional system for traffic flow prediction. First, we propose a dynamic spatial block to capture the complex and dynamic spatial dependencies, which is computation-friendly. Next, we propose a dynamic temporal block to capture the complex and dynamic temporal dependencies, which well balances the short- and long-range dynamic temporal dependencies. We validate and analyze the performance of the proposed method through extensive experiments on two traffic datasets. Analysis of results demonstrates that our proposed model has better prediction performance than the state-of-art baselines. Compared with the best contrast methods, the proposed method improves by 2.28% and 8.01% in terms of the mean absolute error on PEMS04 and PEMS08 datasets.
更多查看译文
关键词
Graph convolutional network,Dynamic spatial–temporal modeling,Attention mechanism,Traffic flow prediction
AI 理解论文
溯源树
样例
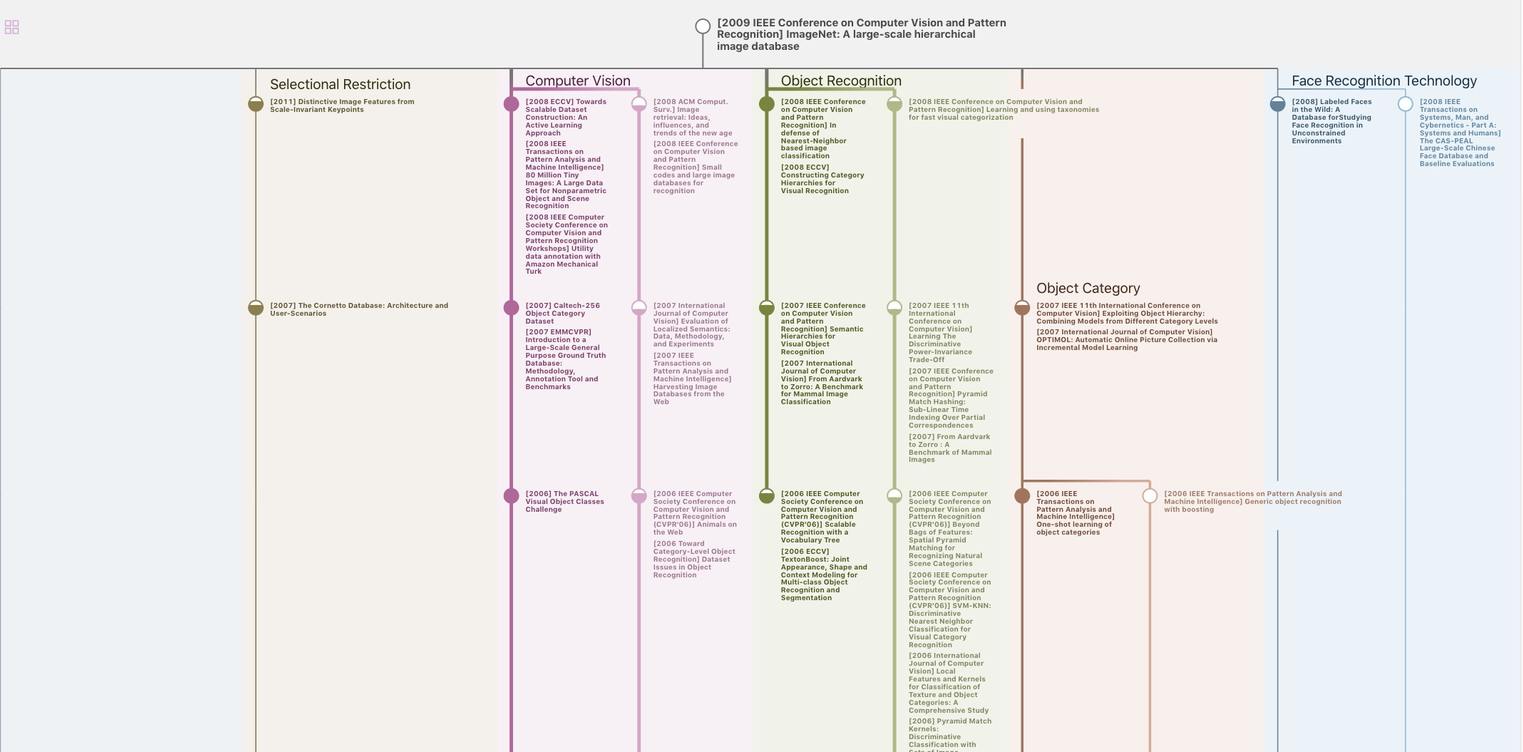
生成溯源树,研究论文发展脉络
Chat Paper
正在生成论文摘要