WAE-TLDN: self-supervised fusion for multimodal medical images via a weighted autoencoder and a tensor low-rank decomposition network
Applied Intelligence(2024)
摘要
Multimodal medical image fusion (MMIF) integrates the advantages of multiple source images to assist clinical diagnosis. Existing image fusion methods need help to distinguish the importance between features and often define features to be retained subjectively, which leads to global structure loss and limits the performance of fusion. To overcome these restrictions, we propose a novel self-supervised tensor low-rank decomposition fusion network that can effectively extract global information from high-rank to low-rank conversion processes. Specifically, the compensation of textural features is performed by employing a self-supervised auxiliary task, and the whole network is dynamically fine-tuned according to a hybrid loss. In our model, an enhanced weights (EW) estimation method based on the global luminance contrast is developed, and a structure tensor loss with constraints is introduced to improve the robustness of the fusion results. Moreover, extensive experiments on six types of multimodal medical images show that visual and qualitative results are superior to competitors, validating the effectiveness of our methods.
更多查看译文
关键词
Multimodal medical image fusion,Weighted autoencoder,Tensor low-rank decomposition,Feature enhancement network,Structure tensor loss
AI 理解论文
溯源树
样例
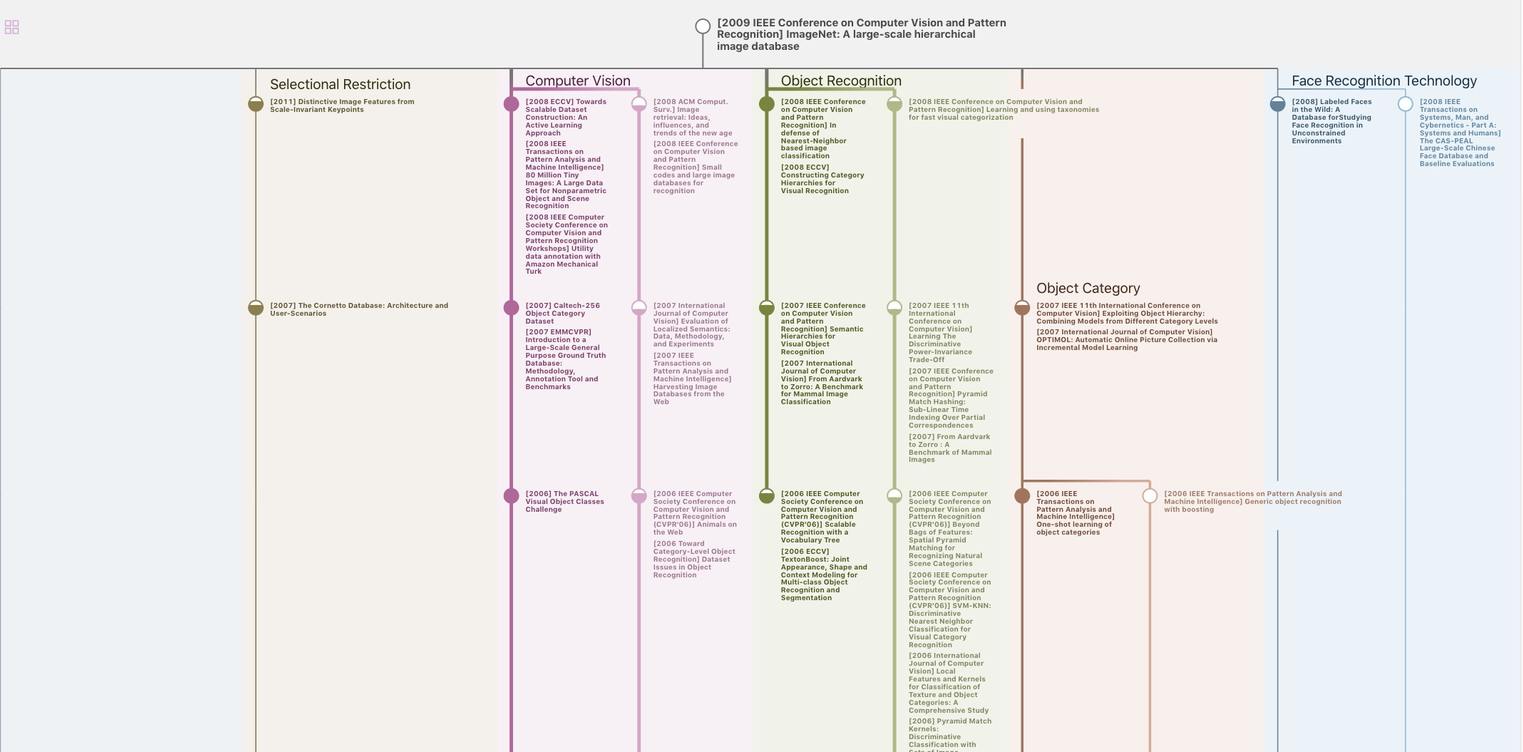
生成溯源树,研究论文发展脉络
Chat Paper
正在生成论文摘要