Adversarial perturbation denoising utilizing common characteristics in deep feature space
Applied Intelligence(2024)
摘要
Recent studies have shown that deep neural networks (DNNs) are vulnerable to adversarial examples (AEs). Denoising based on the input pre-processing is one of the defenses against adversarial attacks. However, it is hard to remove multiple adversarial perturbations, especially in the presence of evolving attacks. To address this challenge, we attempt to extract the commonality of adversarial perturbations. Due to the imperceptibility of adversarial perturbations in the input space, we conduct the extraction in the deep feature space where the perturbations become more apparent. Through the obtained common characteristics, we craft common adversarial examples (CAEs) to train the denoiser. Furthermore, to prevent image distortion while removing as much of the adversarial perturbation as possible, we propose a hybrid loss function that guides the training process at both the pixel level and the deep feature space. Our experiments show that our defense method can eliminate multiple adversarial perturbations, significantly enhancing adversarial robustness compared to previous state-of-the-art methods. Moreover, it can be plug-and-play for various classification models, which demonstrates the generalizability of our defense method.
更多查看译文
关键词
Deep neural network,Adversarial examples,Deep feature,Denoiser,Adversarial robustness
AI 理解论文
溯源树
样例
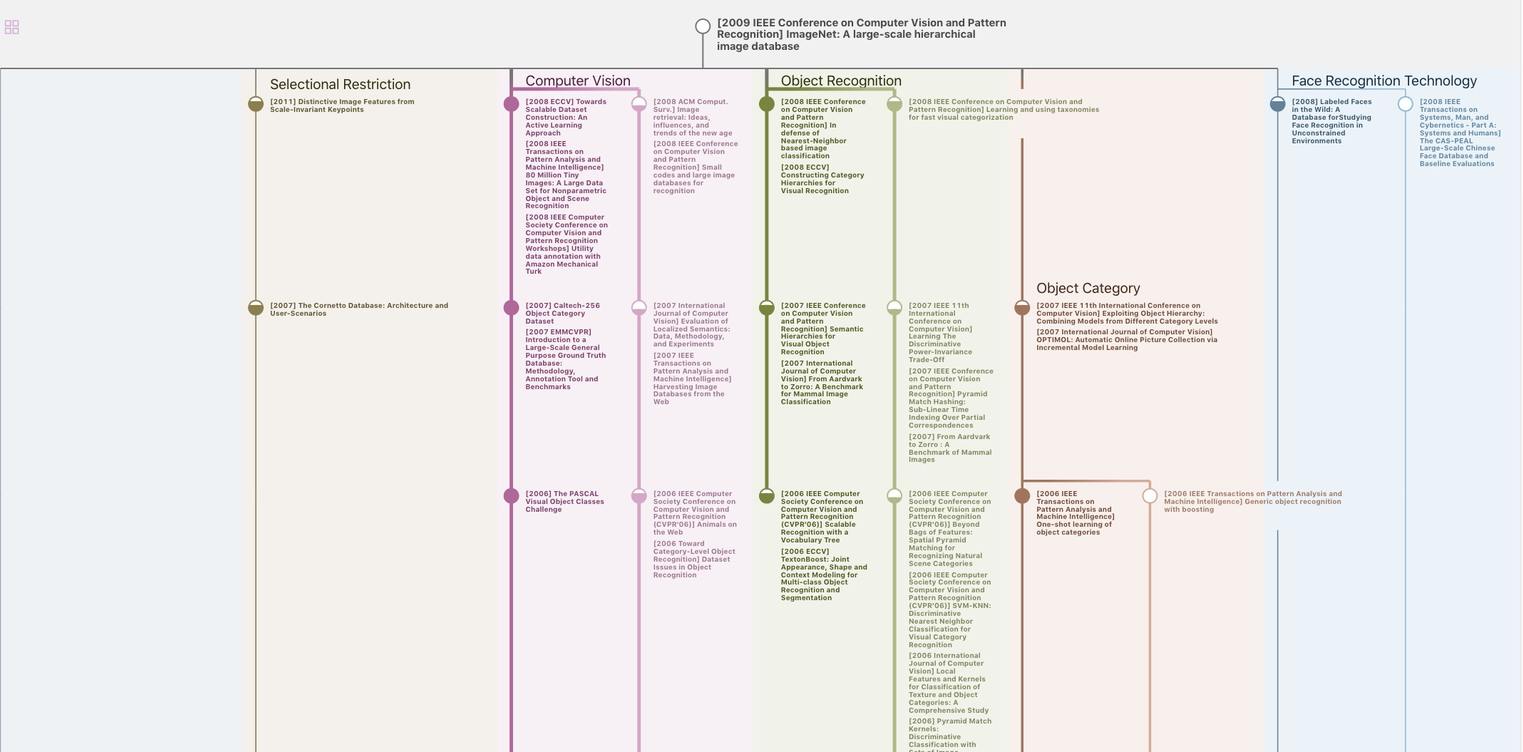
生成溯源树,研究论文发展脉络
Chat Paper
正在生成论文摘要