Exploiting Context and Attention Using Recurrent Neural Network for Sensor Time Series Prediction
ADVANCED ANALYTICS AND LEARNING ON TEMPORAL DATA, AALTD 2023(2023)
摘要
In the current era of Internet of Things, typically data from multiple sources are captured through various sensors yielding Multivariate Time Series (MTS) data. Sensor MTS prediction has several real-life applications in various domains such as healthcare, manufacturing, and agriculture. In this paper, we propose a novel Recurrent Neural Network (RNN) architecture that leverages contextual information and attention mechanism for sensor MTS prediction. We adopt the notion of primary and contextual features to distinguish between the features that are independently useful for learning irrespective of other features, and the features that are not useful in isolation. The contextual information is represented through the contextual features and when used with primary features can potentially improve the performance of the model. The proposed architecture uses the contextual features in two ways. Firstly, to weight the primary input features depending on the context, and secondly to weight the hidden states in the alignment model. The latter is used to compute the dependencies between hidden states (representations) to derive the attention vector. Further, integration of the context and attention allows visualising temporally and spatially the relevant parts of the input sequence which are influencing the prediction. To evaluate the proposed architecture, we used two benchmark datasets as they provide contextual information. The first is NASA Turbofan Engine Degradation Simulation dataset for estimating Remaining Useful Life, and the second is appliances energy prediction dataset. We compared the proposed approach with the state-of-the-art methods and observed improved prediction results, particularly with respect to the first dataset.
更多查看译文
关键词
Recurrent Neural Network,Gated Recurrent Unit,Context,Attention,Multivariate Sensor Time Series,Remaining Useful Life,Appliance energy prediction
AI 理解论文
溯源树
样例
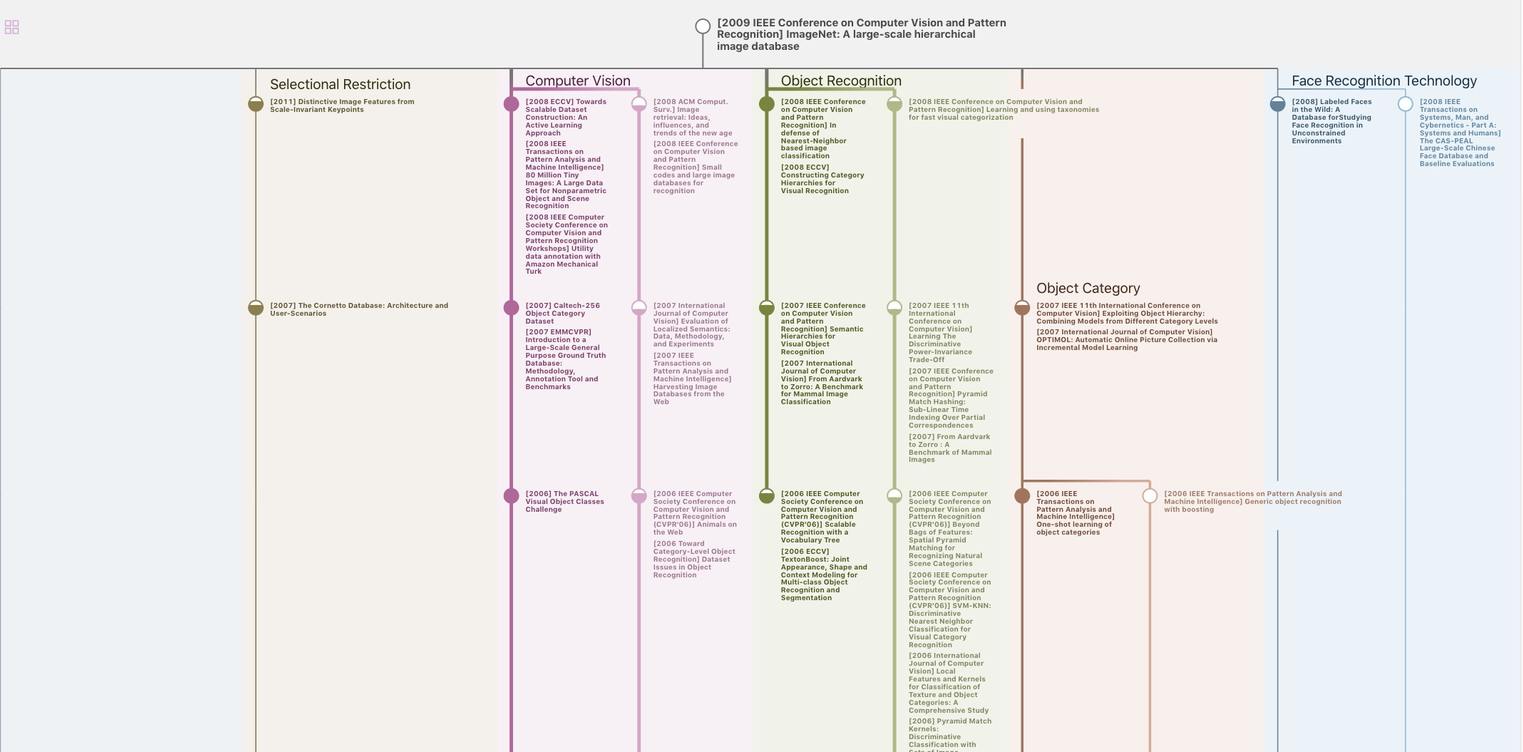
生成溯源树,研究论文发展脉络
Chat Paper
正在生成论文摘要