Spatial-Temporal Masked Autoencoder for Multi-Device Wearable Human Activity Recognition.
Proc. ACM Interact. Mob. Wearable Ubiquitous Technol.(2023)
摘要
The widespread adoption of wearable devices has led to a surge in the development of multi-device wearable human activity recognition (WHAR) systems. Nevertheless, the performance of traditional supervised learning-based methods to WHAR is limited by the challenge of collecting ample annotated wearable data. To overcome this limitation, self-supervised learning (SSL) has emerged as a promising solution by first training a competent feature extractor on a substantial quantity of unlabeled data, followed by refining a minimal classifier with a small amount of labeled data. Despite the promise of SSL in WHAR, the majority of studies have not considered missing device scenarios in multi-device WHAR. To bridge this gap, we propose a multi-device SSL WHAR method termed Spatial-Temporal Masked Autoencoder (STMAE). STMAE captures discriminative activity representations by utilizing the asymmetrical encoder-decoder structure and two-stage spatial-temporal masking strategy, which can exploit the spatial-temporal correlations in multi-device data to improve the performance of SSL WHAR, especially on missing device scenarios. Experiments on four real-world datasets demonstrate the efficacy of STMAE in various practical scenarios.
更多查看译文
关键词
annotation scarcity,human activity recognition,self-supervised learning,wearable sensors
AI 理解论文
溯源树
样例
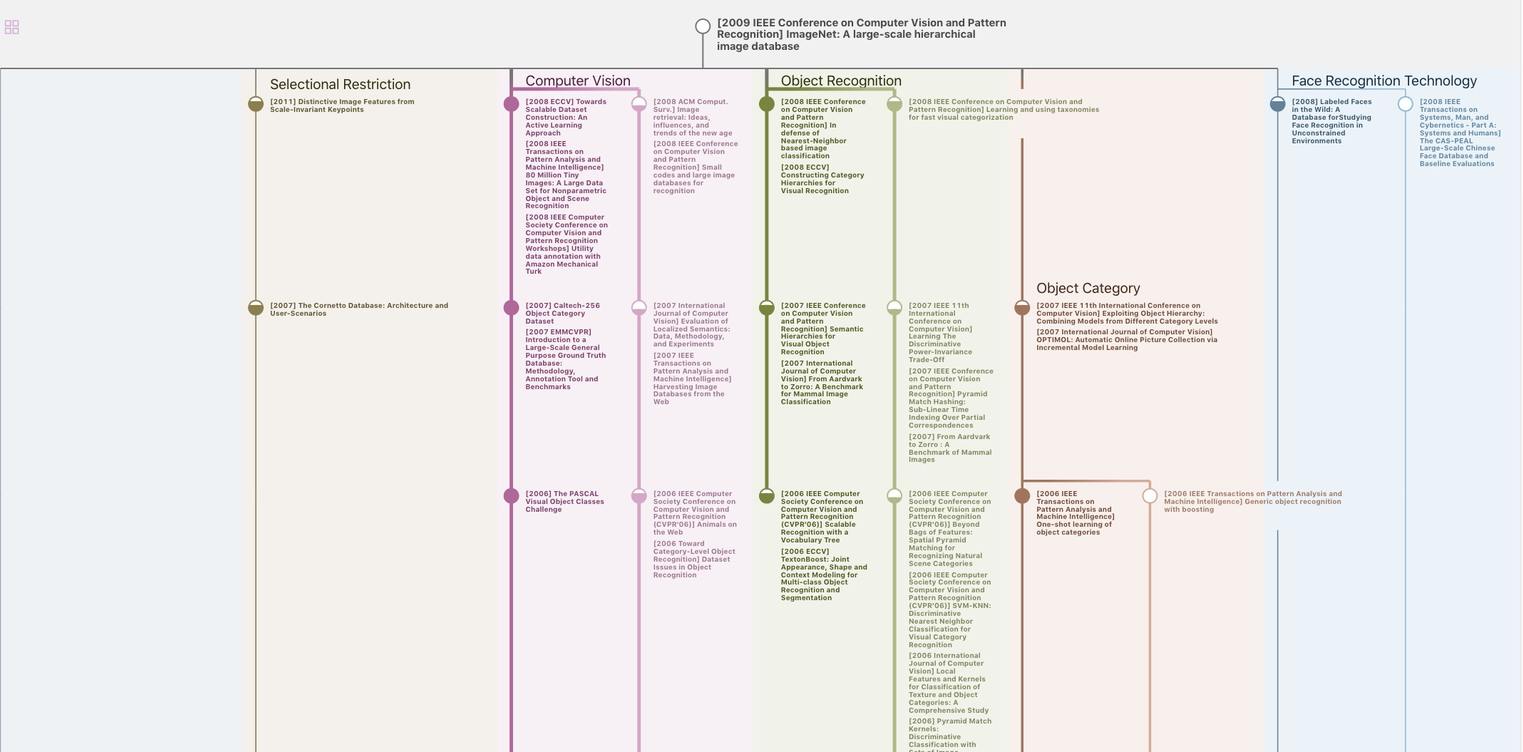
生成溯源树,研究论文发展脉络
Chat Paper
正在生成论文摘要