Graph Neural Network Encoder for Layout Planning and Scheduling in Line-less Mobile Assembly Systems
Procedia CIRP(2023)
摘要
Facing the trade-off between the customer's needs and the shift towards sustainable production pressure challenges companies to make their production systems more flexible. Line-less mobile assembly systems (LMAS) implement the needed adaptivity through flexible job routes, multi-purpose resources, and flexible transportation systems. A completely reactive layout through (re-)arrangement of stations with mobile resources allows for reconfigurations without interrupting production. Deep reinforcement learning algorithms are needed to dynamically solve the integrated layout optimization and scheduling problem in LMAS. Within LMAS, stations can be added to the assembly system, or the number of jobs and technologies in the system can vary. Thus, reinforcement learning control agents must produce actions for problem instances of varying sizes. Therefore, an encoder is needed to enable the scheduling policy to handle the differently sized system state descriptions. This paper presents an encoder for LMAS using a Graph Neural Network (GNN). The developed algorithm is trained using a Discrete Event Simulation and validated based on several use cases. As a result, the developed encoder allows for system state representation over all use cases.
更多查看译文
关键词
Production Control,Production scheduling,Graph Neural Network,Layout optimization,Flexible assembly
AI 理解论文
溯源树
样例
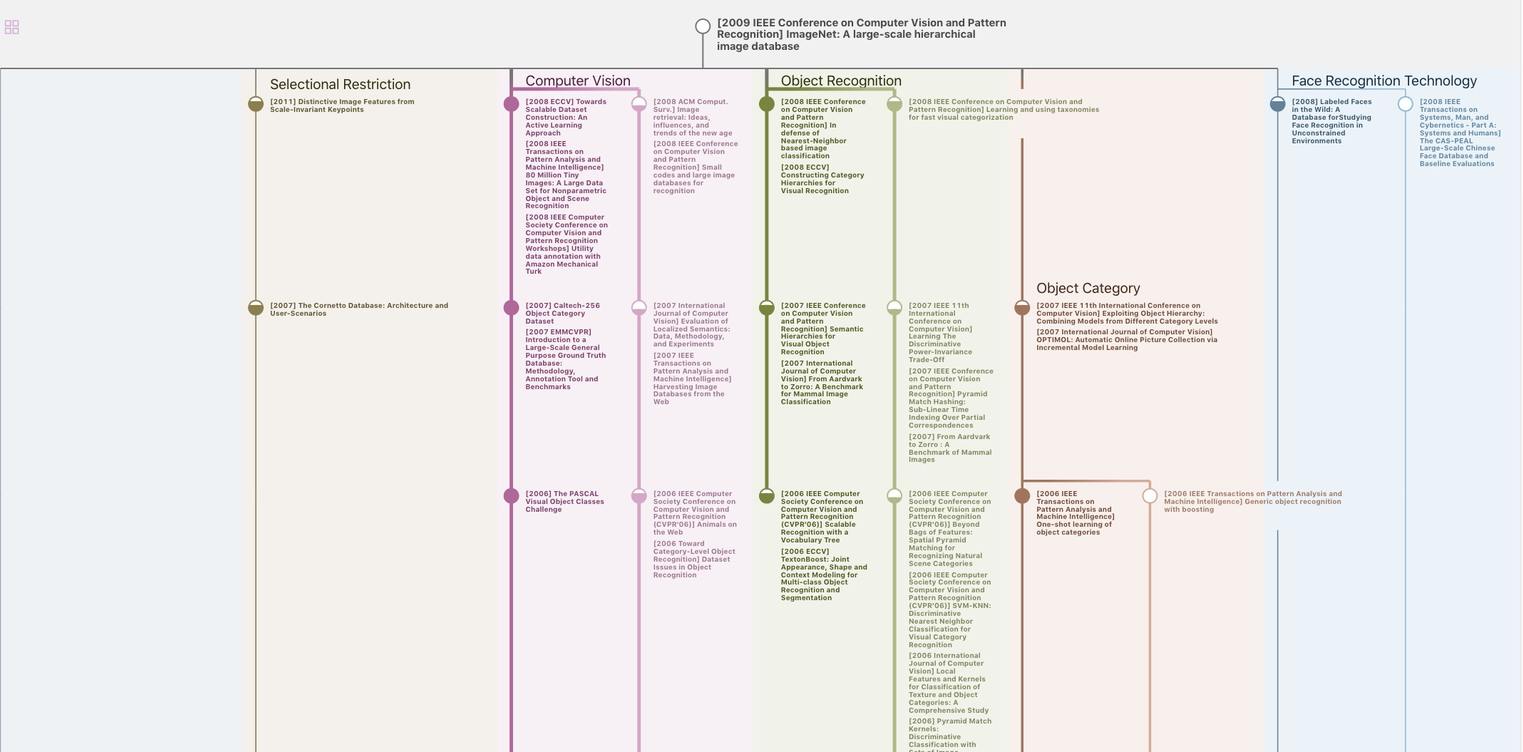
生成溯源树,研究论文发展脉络
Chat Paper
正在生成论文摘要