A high-resolution map of soil organic carbon in cropland of Southern China
CATENA(2024)
摘要
An accurate and fine map of soil organic carbon (SOC) plays a vital role in understanding the global carbon cycle and achieving soil carbon sequestration potential. Although global and national maps of SOC are already available with various spatial resolutions, limited sample size, and relative coarse resolution hinder its accuracy and application in local regions. Here, we collected 13,424 soil samples and information of 45 environmental covariates from cropland in Jiangxi Province, Southern China during 2012 and 2013. Then, the optimal covariates were selected by a recursive feature elimination algorithm for mapping SOC. After that, we deployed the random forest (RF) to produce a fine map (30 m) of SOC in the cropland of Jiangxi Province and 100 times bootstrapping was performed to calculate the prediction uncertainty. Finally, we determined the impacts of various covariates on SOC variability using RF and partial least squares structural equation modeling. Our results showed that compared with the predictive model without soil management information, introducing soil management information improved the predictability of SOC with an increase in R-2 by 7.35 % (0.73 vs 0.68) and decrease in RMSE by 7.03 % (2.91 vs 3.13 g kg(-1)). Our results well estimated the uncertainty of the predicted result with a PICP of 0.91 for a 90 % prediction interval. Soil properties and soil management activities make the largest contribution for modelling SOC. Specifically, the total nitrogen content, straw return amount, total potassium content, and multi-resolution valley bottom flatness were found as the most important factors for mapping SOC in the cropland of our study area. Overall, this study deepened our knowledge of the variation of SOC and also emphasizes that incorporating soil management information could help us to achieve more accurate predictions of SOC.
更多查看译文
关键词
Digital soil mapping,Soil organic carbon,Random Forest,Variable selection,Cropland,Controlling factors
AI 理解论文
溯源树
样例
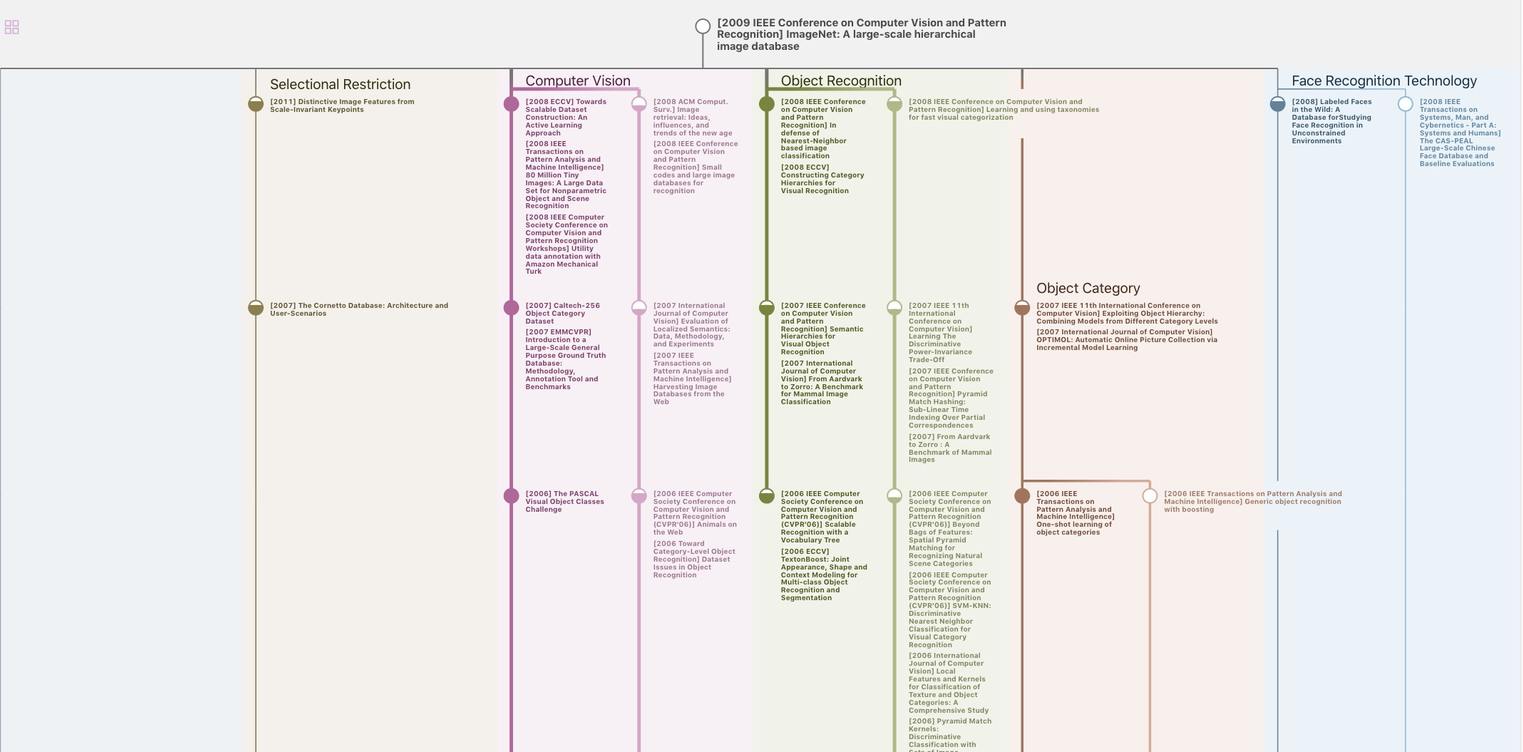
生成溯源树,研究论文发展脉络
Chat Paper
正在生成论文摘要