Intelligent Wireless Resource Management in Industrial Camera Systems: Reinforcement Learning-based AI-extension for Efficient Network Utilization
Computer communications(2024)
摘要
In this paper, we present an innovative method for replacing wired communication within an operational agile production cell with wireless communication, using a minimal number of access points. Our approach utilizes Reinforcement Learning (RL) to optimize the layout and minimize the required number of access points. We introduce an Artificial Intelligence (AI) agent that facilitates cooperative interaction between cameras and access points, optimizing camera stream utilization and radio coverage. This solution benefits from the recent standardization of The fifth generation of mobile communications (5G) Network Resource Management (NRM) features of the User Equipment (UE) attached to the cameras. To accommodate the dynamic nature of the production cell, our optimization algorithms run during cell operation, necessitating extended training times. Our contributions extend beyond the state-of-the-art by offering a Digital Twin (DT) environment equipped with physics and radio propagation capabilities, fast raytracing for radio propagation modeling, curriculumlearning -based reinforcement learning, and a Physical Twin (PT) of the radio -connected camera with selfadjusting scene setup capabilities. This comprehensive proposal combines the efficiency of 5G radio utilization with precise modeling and high -resolution simulations, while also significantly improving training speed. In the Agile Robotics for Industrial Automation Competition (ARIAC) 2020 environment, our methods showed compelling results, with curriculum learning achieving a remarkable 3x speed-up compared to noncurriculum approaches. We also demonstrated that high Quality of Service (QoS) demands can be reduced to just 2% of total time while maintaining consistent productivity Key Performance Indicators (KPIs). Furthermore, we explored the integration of these methods into Internet of Things (IoT) devices with limited performance capabilities. In conclusion, our approach offers significant benefits and savings for IoT devices, networks, edge clouds, and industry players.
更多查看译文
关键词
Digital twin,Network resource management,Wireless,Manufacturing/assembly,Artificial intelligence
AI 理解论文
溯源树
样例
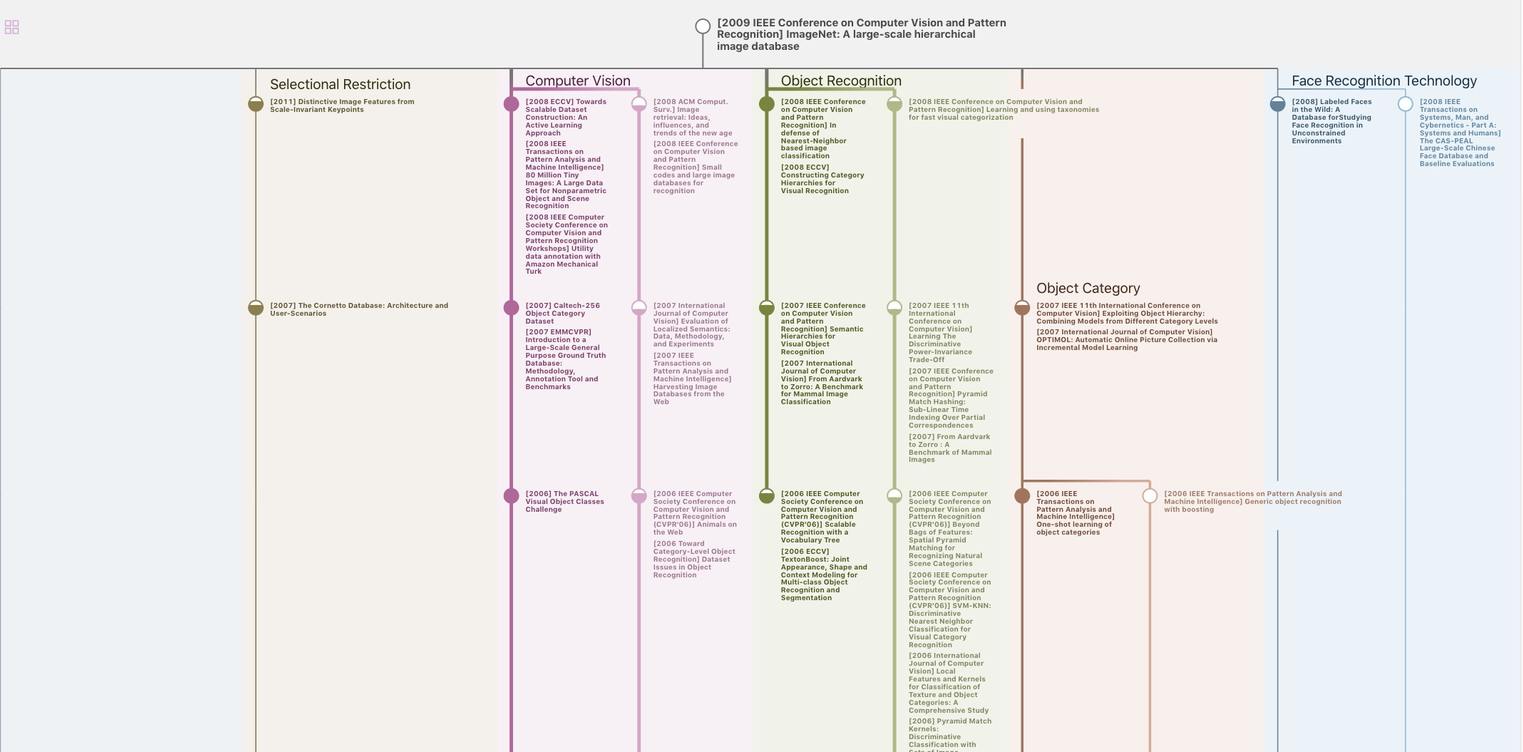
生成溯源树,研究论文发展脉络
Chat Paper
正在生成论文摘要