Robust trajectory tracking of quadrotors using adaptive radial basis function network compensation control
JOURNAL OF THE FRANKLIN INSTITUTE-ENGINEERING AND APPLIED MATHEMATICS(2024)
摘要
Radial Basis Function Neural Networks (RBFNN) methods have gained incredible efficiency and applicability in control. This paper presents a nested control strategy for robust trajectory tracking of a quadrotor using adaptive RBF compensation and NN -supervised control embedded with Integrator BackStepping (IBS). The approach addresses the robustness in the presence of modeling uncertainties, sensing noise, and bounded disturbances. The control design is derived from the decentralized inverse dynamics, using adaptive RBFNN for outer -loop disturbance approximation and compensation. In conjunction with an Inner -loop supervised control that stabilizes the quadrotor attitude, preventing initial instability during NN convergence. In addition, an adaptive Extended Kalman Filter (EKF) attenuates noisy signals. Simulation results demonstrate strong adaptability to changes in model parameters, and superior performance when compared to Proportional Integral Derivative (PID), Integrator BackStepping (IBS), and offline decentralized Multi -Layer Perceptron (MLP) algorithms, in terms of parameter convergence, disturbance compensation control, and noise attenuation.
更多查看译文
关键词
Neural networks,Radial Basis Functions,Disturbance compensation,Kalman filter,Robust adaptive control
AI 理解论文
溯源树
样例
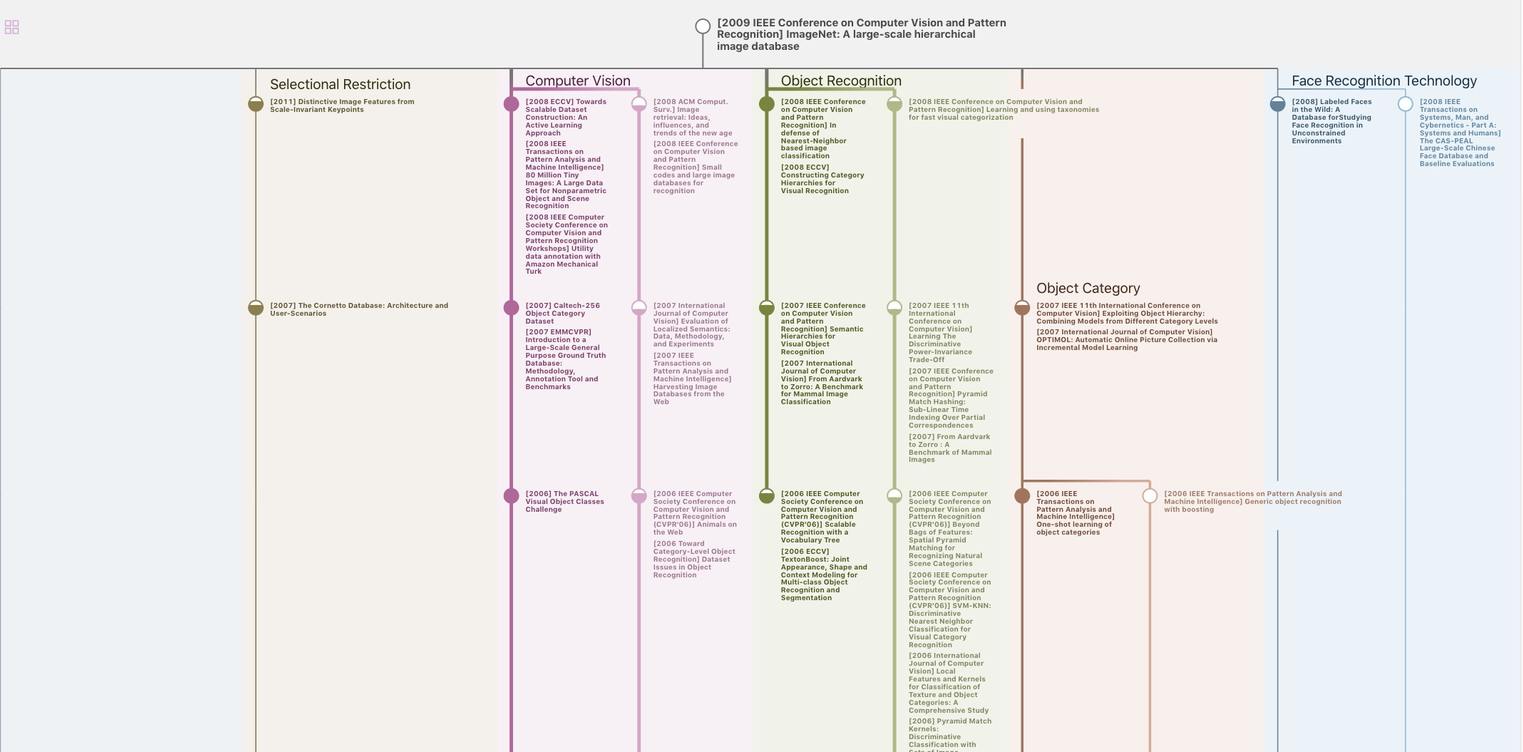
生成溯源树,研究论文发展脉络
Chat Paper
正在生成论文摘要