Noise-robust voice conversion using adversarial training with multi-feature decoupling
ENGINEERING APPLICATIONS OF ARTIFICIAL INTELLIGENCE(2024)
摘要
Most existing voice conversion methods focus primarily on separating speech content from speaker information while overlooking the decoupling of pitch information. Additionally, the quality of converted speech significantly degrades when the speech of the target speaker is contaminated by noises. To address these issues, this paper proposes a noise-robust voice conversion model with multi-feature decoupling based on adversarial training. The proposed framework utilizes three distinct encoders to encode speech content, speaker identity, and pitch information independently, which aims to enhance the performance of decoupling by minimizing their mutual information and reduce the correlations between feature vectors. Moreover, a gradient reversal layer and a noise decoupling discriminator are incorporated into the framework, which extracts noise-resistant speaker representations and content representations through adversarial training to facilitate the synthesis of highquality speech. In order to optimize the learning process, a training strategy is developed which involves alternating between clean and noisy data during the training of the encoder. This strategy effectively guides and expedites the convergence of the model. Experimental results demonstrate that compared to the state-of-the-art baselines of noise-robust voice conversion, the proposed model achieves improvements around 0.31 and 0.39 in terms of speech naturalness and speaker similarity evaluation metrics, respectively.
更多查看译文
关键词
Voice conversion,Noise -robustness,Adversarial training,Multi -feature decoupling,Encoder -decoder,Gradient reversal layer
AI 理解论文
溯源树
样例
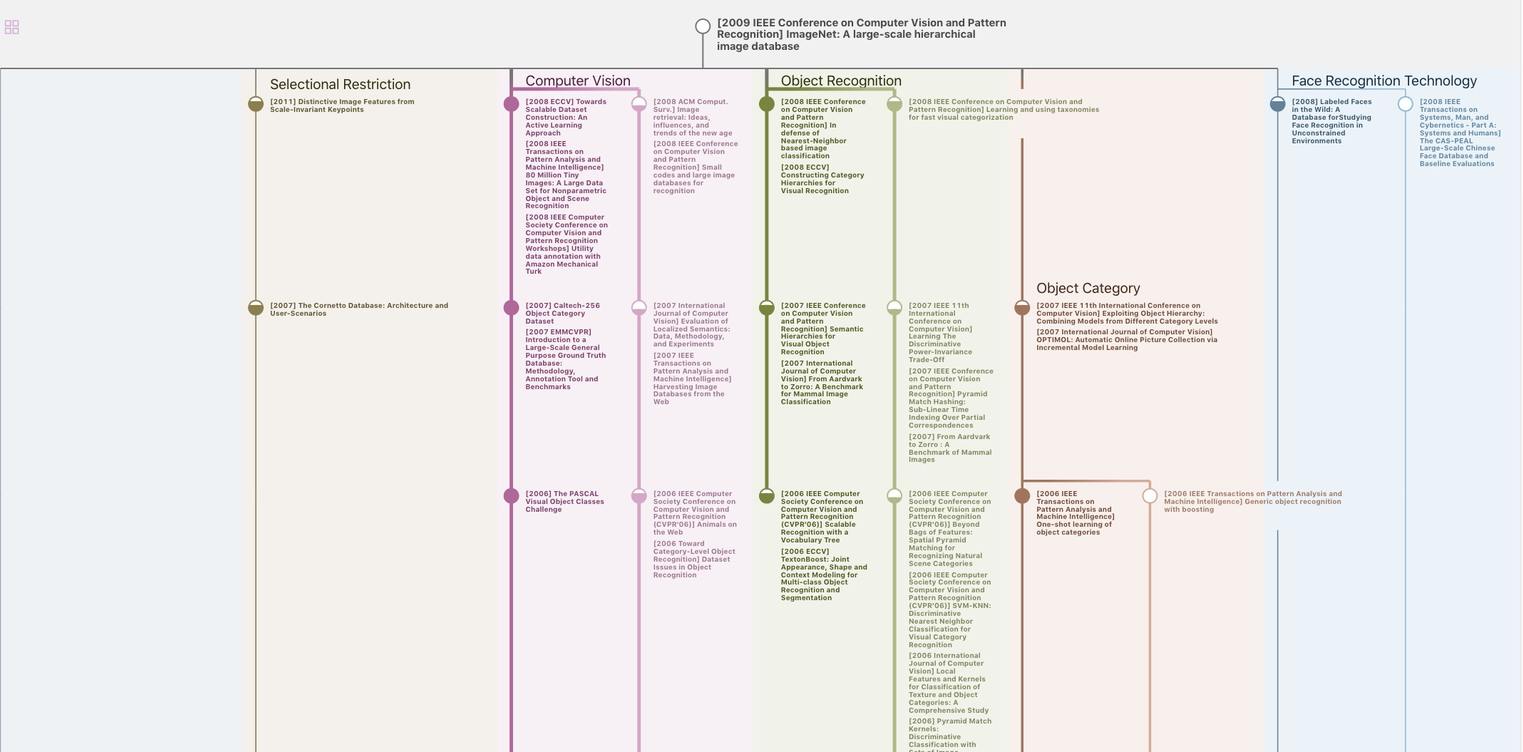
生成溯源树,研究论文发展脉络
Chat Paper
正在生成论文摘要