Learning Scene Graph for Better Cross-Domain Image Captioning
PATTERN RECOGNITION AND COMPUTER VISION, PRCV 2023, PT III(2024)
摘要
The current image captioning (IC) methods achieve good results within a single domain primarily due to training on a large amount of annotated data. However, the performance of single-domain image captioning methods suffers when extended to new domains. To address this, we propose a cross-domain image captioning framework, called SGCDIC, which achieves cross-domain generalization of image captioning models by simultaneously optimizing two coupled tasks, i.e., image captioning and text-to-image synthesis (TIS). Specifically, we propose a scene-graph-based approach SGAT for image captioning tasks. The image synthesis task employs a GAN variant (DFGAN) to synthesize plausible images based on the generated text descriptions by SGAT. We compare the generated images with the real images to enhance the image captioning performance in new domains. We conduct extensive experiments to evaluate the performance of SGCDIC by using the MSCOCO as the source domain data, and using Flickr30k and Oxford-102 as the new domain data. Sufficient comparative experiments and ablation studies demonstrate that SGCDIC achieves substantially better performance than the strong competitors for the cross-domain image captioning task.
更多查看译文
关键词
Image Captioning,Scene Graph,Text-to-Image Synthesis,Dual Learning
AI 理解论文
溯源树
样例
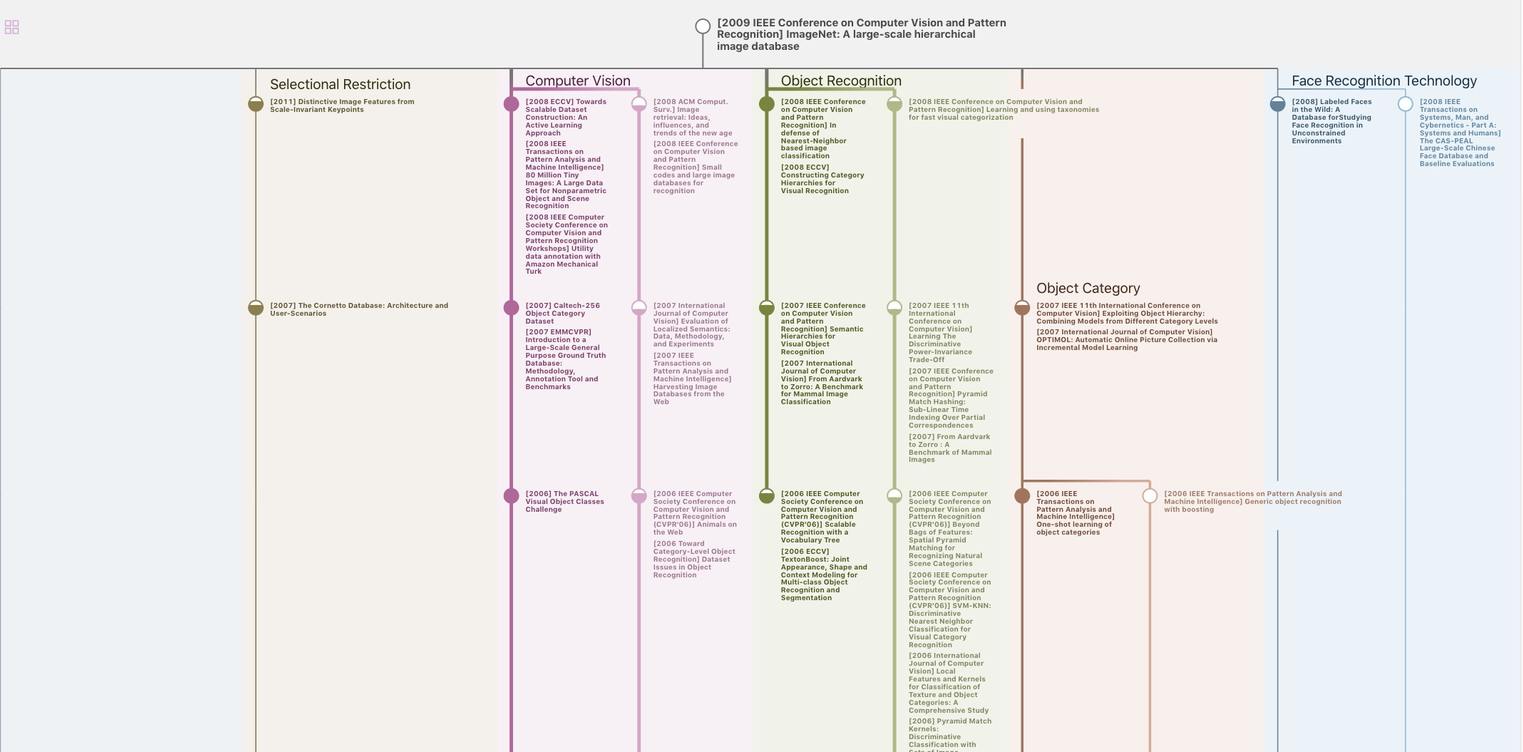
生成溯源树,研究论文发展脉络
Chat Paper
正在生成论文摘要