Where to Focus: Central Attention-Based Face Forgery Detection
PATTERN RECOGNITION AND COMPUTER VISION, PRCV 2023, PT V(2024)
摘要
Face forgery detection in compressed images is an active area of research. However, previous frequency-based methods are subject to two limitations. One aspect to consider is that they apply the same weight to different frequency bands. Moreover, they exhibit an equal treatment of regions that contain distinct semantic information. To address these limitations above, we propose the Central Attention Network (CAN), a multi-modal architecture comprising two bright components: Adaptive Frequency Embedding (AFE) and Central Attention (CA) block. The AFE module adaptively embeds practical frequency information to enhance forged traces and minimize the impact of redundant interference. Moreover, the CA block can achieve fine-grained trace observation by concentrating on facial regions where indications of forgery frequently manifest. CAN is efficient in extracting forgery traces and robust to noise. It effectively reduces the unnecessary focus of our model on irrelevant factors. Extensive experiments on multiple datasets validate the advantages of CAN over existing state-of-the-art methods.
更多查看译文
关键词
Face Forgery Detection,Multi-level Frequency Fusion,Attention Mechanism
AI 理解论文
溯源树
样例
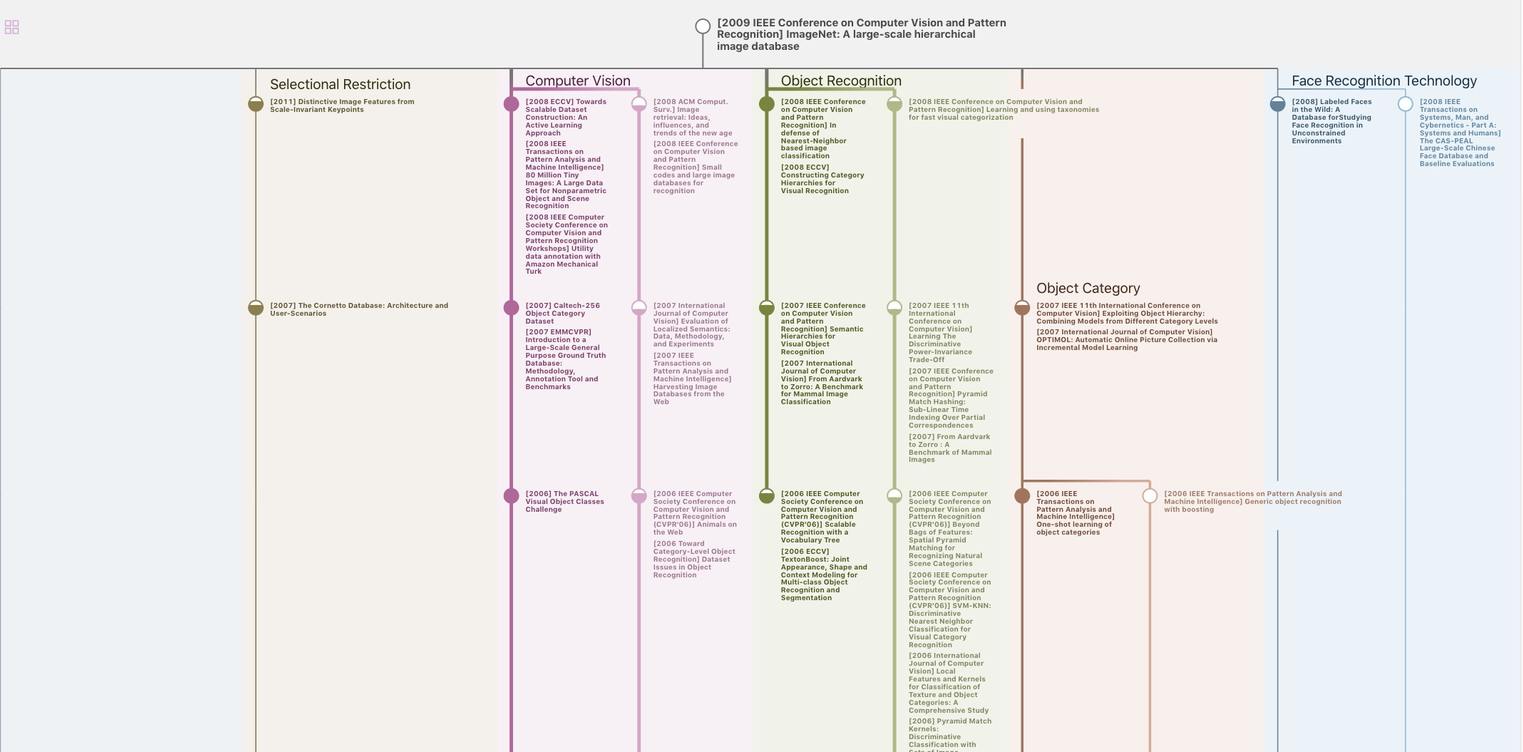
生成溯源树,研究论文发展脉络
Chat Paper
正在生成论文摘要