RECO: Rotation Equivariant COnvolutional Neural Network for Human Trajectory Forecasting
PATTERN RECOGNITION AND COMPUTER VISION, PRCV 2023, PT III(2024)
摘要
Pedestrian trajectory prediction is a crucial task for various applications, especially for autonomous vehicles that need to ensure safe navigation. To perform this task, it is essential to understand the dynamics of pedestrian motion and account for the uncertainty and multi-modality of human behaviors. However, existing methods often produce inconsistent and unrealistic predictions due to their limited ability to handle different orientations of pedestrians. In this paper, we propose a novel approach that leverages the Euclidean group C4 to enhance convolutional neural networks with rotation equivariance. This property enables the networks to learn features that are invariant to rotations, thus maintaining consistent output under various orientations. We present the Rotation Equivariant COnvolutional Neural Network (RECO), a model specifically designed for pedestrian trajectory prediction using rotation equivariant convolutions. We evaluate our model on challenging realworld human trajectory forecasting datasets and show that it achieves competitive performance compared to state-of-the-art methods.
更多查看译文
关键词
Human Trajectory Forecasting,Multimodality,Uncertainty,Rotation Equivariant Convolution
AI 理解论文
溯源树
样例
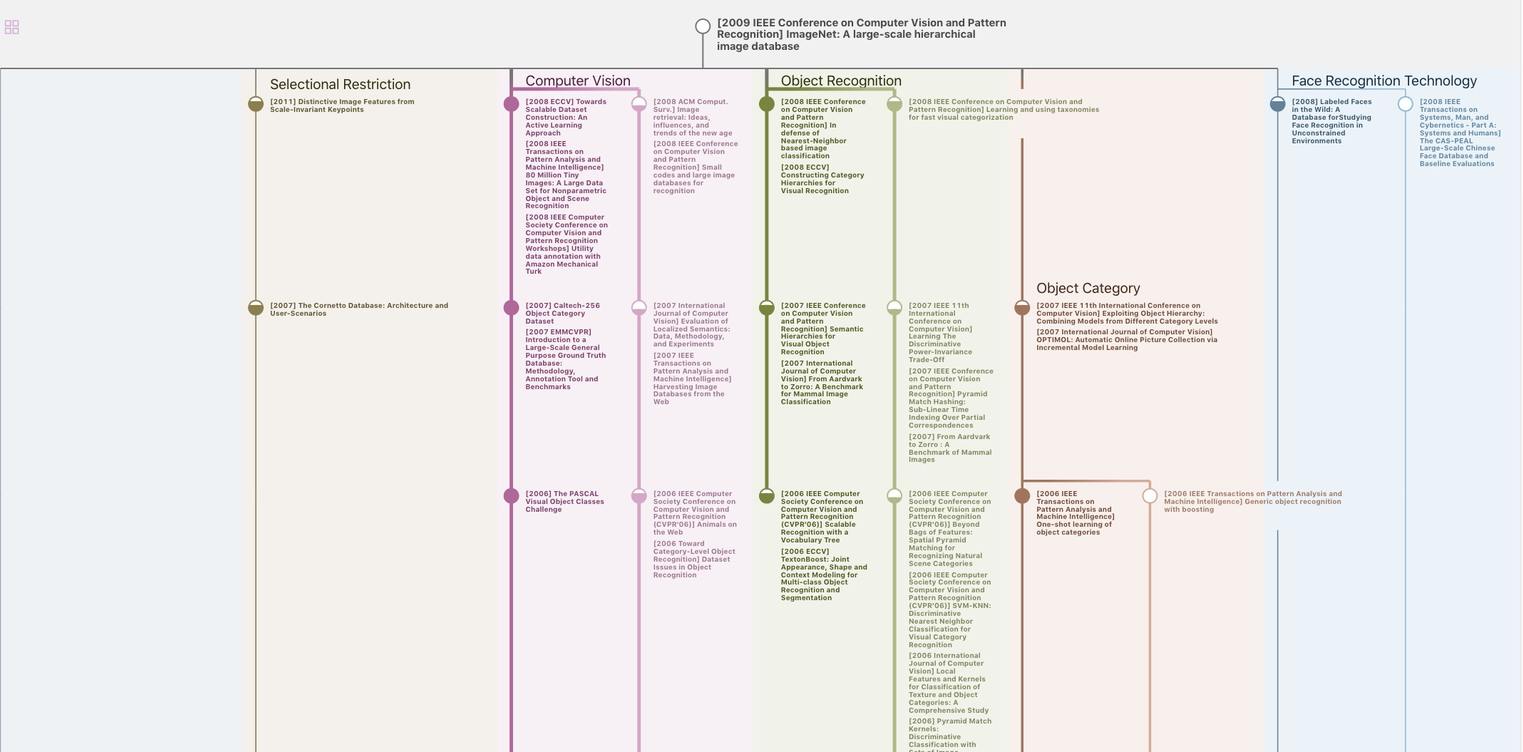
生成溯源树,研究论文发展脉络
Chat Paper
正在生成论文摘要