Pixel-Level Sonar Image JND Based on Inexact Supervised Learning
PATTERN RECOGNITION AND COMPUTER VISION, PRCV 2023, PT XI(2024)
摘要
The Just Noticeable Difference (JND) model aims to identify perceptual redundancies in images by simulating the perception of the Human Visual System (HVS). Exploring the JND of sonar images is important for the study of their visual properties and related applications. However, there is still room for improvement in performance of existing JND models designed for Natural Scene Images (NSIs), and the characteristics of sonar images are not sufficiently considered by them. On the other hand, there are significant challenges in constructing a densely labeled pixel-level JND dataset. To tackle these issues, we proposed a pixel-level JND model based on inexact supervised learning. A perceptually lossy/lossless predictor was first pre-trained on a coarsegrained picture-level JND dataset. This predictor can guide the unsupervised generator to produce an image that is perceptually lossless compared to the original image. Then we designed a loss function to ensure that the generated image is perceptually lossless and maximally different from the original image. Experimental results show that our model outperforms current models.
更多查看译文
关键词
Just Noticeable Difference (JND),Inexact Supervised Learning,Sonar Images
AI 理解论文
溯源树
样例
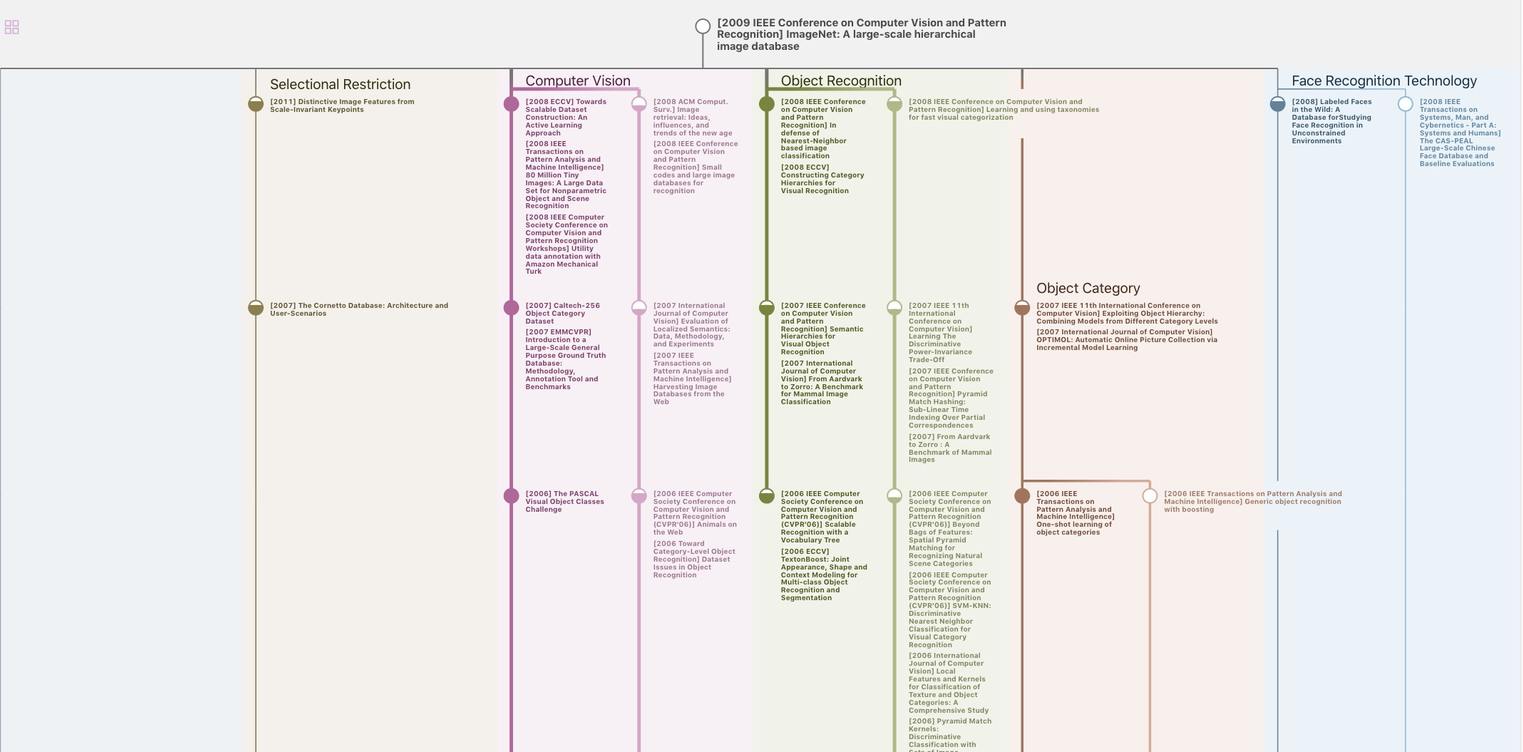
生成溯源树,研究论文发展脉络
Chat Paper
正在生成论文摘要