Learning Domain-Invariant Representations from Text for Domain Generalization
PATTERN RECOGNITION AND COMPUTER VISION, PRCV 2023, PT VIII(2024)
Abstract
Domain generalization (DG) aims to transfer the knowledge learned in the source domain to the unseen target domain. Most DG methods focus on studying how to learn domain-invariant representations that remain invariant across different domains. For humans, we tend to use the same word or text to describe images from different domains but of the same category. Therefore, text can be considered a natural domain-invariant representation. Inspired by this, this paper studies how to introduce text representations into domain generalization tasks. Specifically, the text representations generated by CLIP text encoder are used to guide the image representation learning of the visual model. To alleviate domain bias and weak discriminability caused by CLIP representations, a joint loss is proposed by combining the text representation regularization loss with standard image-level supervised loss. The proposed method is simple yet efficient, and can achieve competitive performance compared with the existing state-of-the-art methods on five standard DG datasets.
MoreTranslated text
Key words
Domain Generalization,Transfer learning,CLIP
AI Read Science
Must-Reading Tree
Example
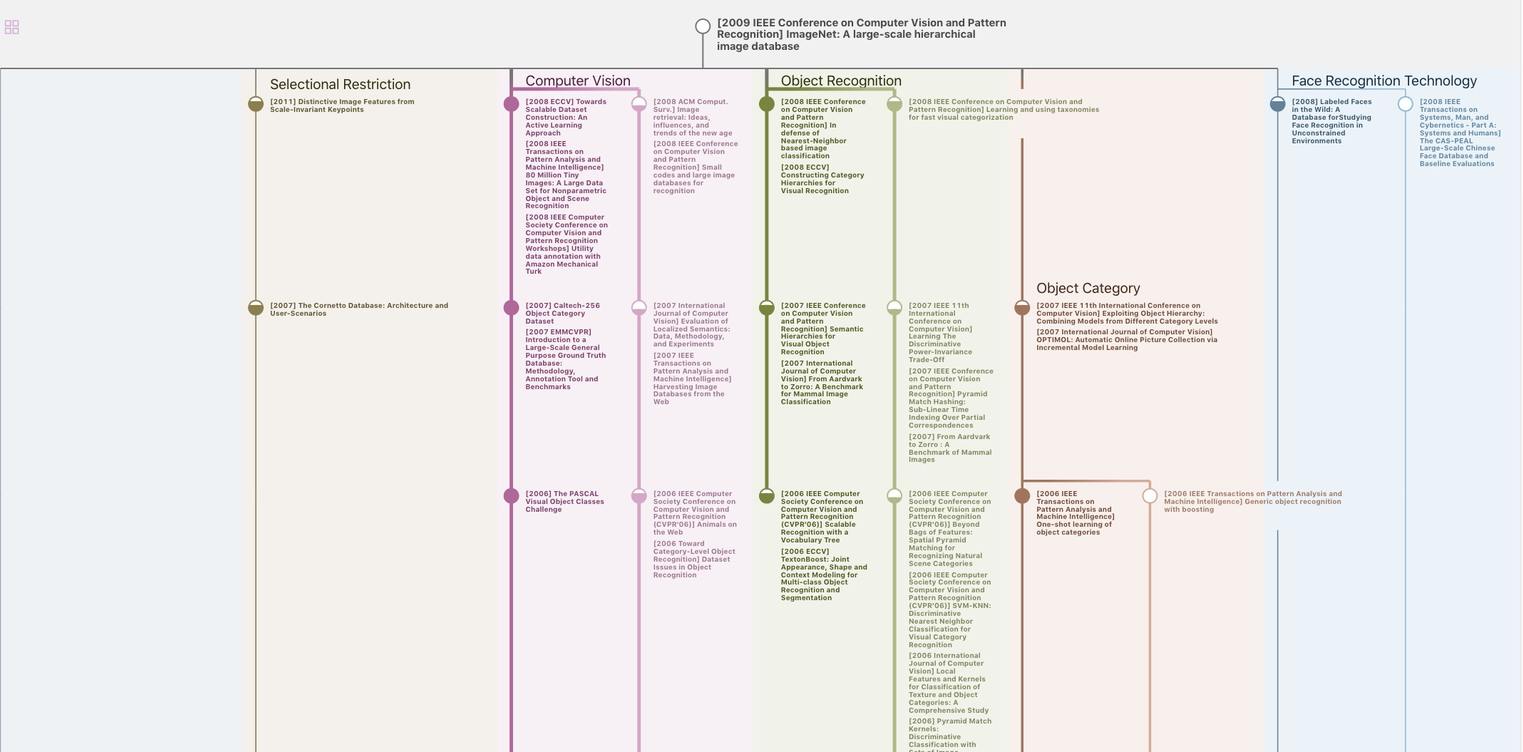
Generate MRT to find the research sequence of this paper
Chat Paper
Summary is being generated by the instructions you defined