TSTD:A Cross-modal Two Stages Network with New Trans-decoder for Point Cloud Semantic Segmentation
PATTERN RECOGNITION AND COMPUTER VISION, PRCV 2023, PT VIII(2024)
摘要
In recent years, exploring integrated heterogeneous features architecture has become one of the hot spots in 3D point cloud understanding. However, the efficacy of end-to-end training in enhancing the precision of multi-view fusion for point cloud segmentation and its flexibility remain limited. Furthermore, it is worth highlighting that prior studies have consistently employed encoder-decoder architectures, predominantly emphasizing the refinement of encoder designs, while relatively neglecting the significance of decoders which ultimately leads to increased computing costs. In this study, we present our novel TSTD model, which exhibits remarkable efficacy and efficiency, addressing the constraints encountered in prior research. Diverging from existing approaches that exclusively employ either geometry or RGB data for semantic segmentation, our proposed methodology incorporates both modalities within a unified, two-stage network architecture. This integrative approach enables the effective fusion of heterogeneous data features, leading to notable enhancements in semantic segmentation outcomes. Moreover, we have devised an innovative and efficient decoder utilizing a lightweight transformer module. This novel design further enhances the decoding process, resulting in improved performance and effectiveness. The performance of our model, TSTD, demonstrates strong results with an mIoU of 72.5% on the ScanNet v2 validation set and 67.6% on the test set. Notably, TSTD outperforms the current leading cross-modal point cloud semantic segmentation method CMX by a significant margin of 6.3% mIoU. It also reduces Flops by 31.4% compared to Point Transformer. Extensive experiments confirm that TSTD achieves state-of-the-art performance in cross-model point cloud semantic segmentation.
更多查看译文
关键词
cross-modal,semantic segmentation,two-stage,decoder
AI 理解论文
溯源树
样例
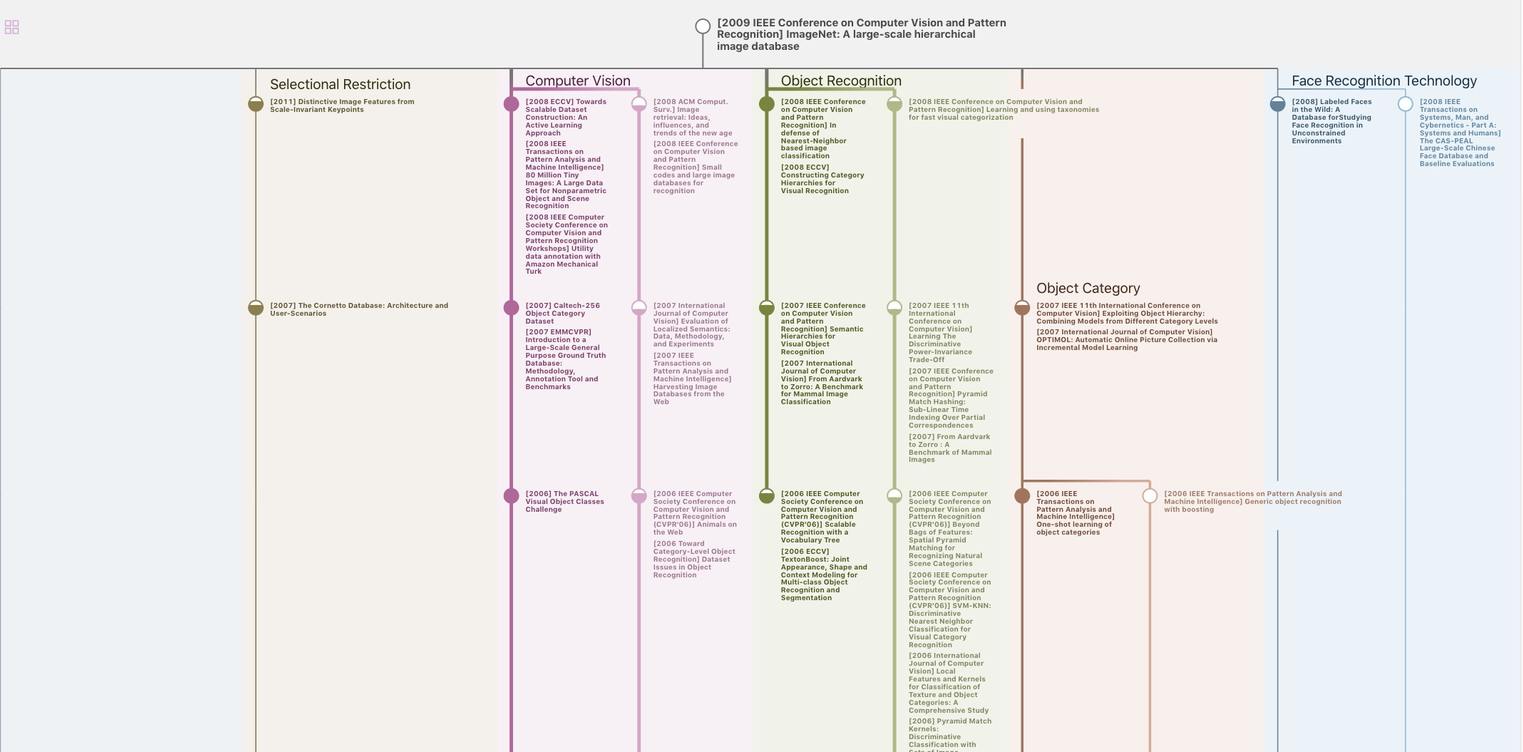
生成溯源树,研究论文发展脉络
Chat Paper
正在生成论文摘要