Enhancing Model Robustness Against Adversarial Attacks with an Anti-adversarial Module
PATTERN RECOGNITION AND COMPUTER VISION, PRCV 2023, PT IX(2024)
摘要
Due to the rapid development of artificial intelligence technologies, such as deep neural networks in recent years, the subsequent emergence of adversarial samples poses a great threat to the security of deep neural network models. In order to defend threats brought by adversarial attacks, the recent mainstream method is to use adversarial training methods to add adversarial samples into model's training process. Although such a type of method can defend against adversarial attacks, it requires increased computing resources and time, and reduces the accuracy of the original samples. Adversarial defense method that conduct anti-adversity in the inference stage. Inspired by adversarial example generation methods, we propose a defense against adversarial in the inference phase of the model. The adversarial sample generation is to add disturbances in the direction of maximizing the loss function after obtaining the sample gradient. Therefore, we add a perturbation in the opposite direction of the adversarial example generated before the sample is fed into the network. The main advantages of our method are that the method requires less computing resources and time; and our method can effectively improve the robust accuracy of the model against adversarial attacks. As a summary, the research content in this paper can stabilize adversarial training, alleviate the high resource consumption of adversarial training, and improve the overall robust performance of the model, which is of great significance to adversarial defense.
更多查看译文
关键词
Deep neural network,Adversarial training,Adversarial attack,Adversarial defense
AI 理解论文
溯源树
样例
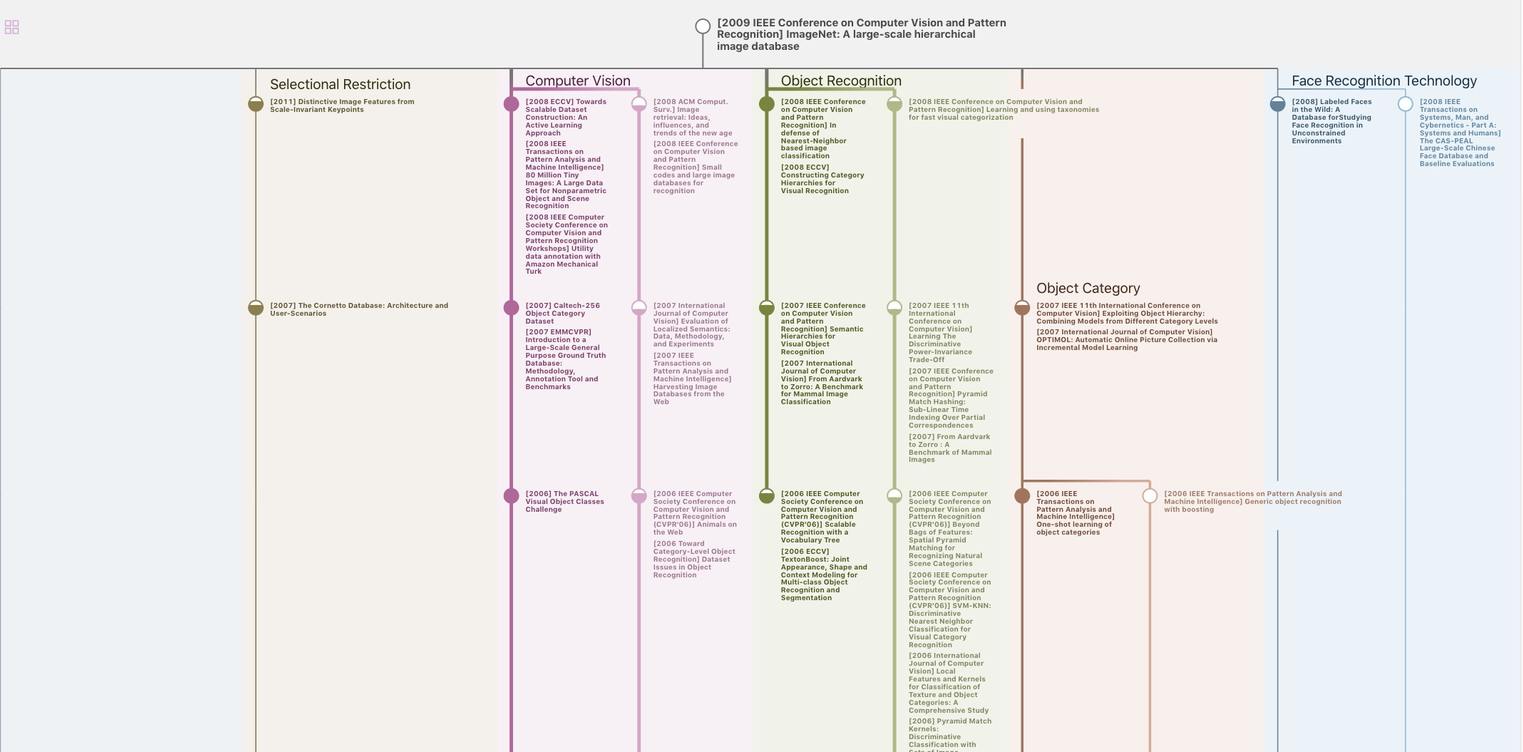
生成溯源树,研究论文发展脉络
Chat Paper
正在生成论文摘要