Consistency Guided Multiview Hypergraph Embedding Learning with Multiatlas-Based Functional Connectivity Networks Using Resting-State fMRI
PATTERN RECOGNITION AND COMPUTER VISION, PRCV 2023, PT IX(2024)
摘要
Recently, resting-state functional connectivity network (FCN) analysis via graph convolutional networks (GCNs) has greatly boosted diagnostic performance of brain diseases in a manner that can refine FCN embeddings by treating FCN as irregular graph-structured data. In this paper, we propose a Consistency Guided Multiview HyperGraph Embedding Learning (CG-MHGEL) framework to integrate FCNs based on multiple brain atlases in multisite studies. First, we model brain network as a hypergraph and develop a multiview hypergraph convolutional network (HGCN) to extract a multiatlas-based FCN embedding for each subject. Here, we employ HGCN rather than GCN to capture more complex information in brain networks, due to the fact that a hypergraph can characterize higher-order relations among multiple vertexes than a widely used graph. Moreover, in order to preserve between-subject associations to promote optimal FCN embeddings, we impose a class-consistency regularization in the embedding space to minimize intra-class dissimilarities while maximizing inter-class dissimilarities for subjects, as well as a site-consistency regularization to further penalize the dissimilarities between intra-class but inter-site subjects. The learned multiatlas-based FCN embeddings are finally fed into fully connected layers followed by the soft-max classifier for brain disease diagnosis. Experimental results on the ABIDE demonstrate the effectiveness of our method for autism spectrum disorder (ASD) identification. Furthermore, the detected ASD-relevant brain regions can be easily traced back with biological interpretability.
更多查看译文
关键词
Autism,embedding,functional connectivity,graph convolution networks,hypergraph
AI 理解论文
溯源树
样例
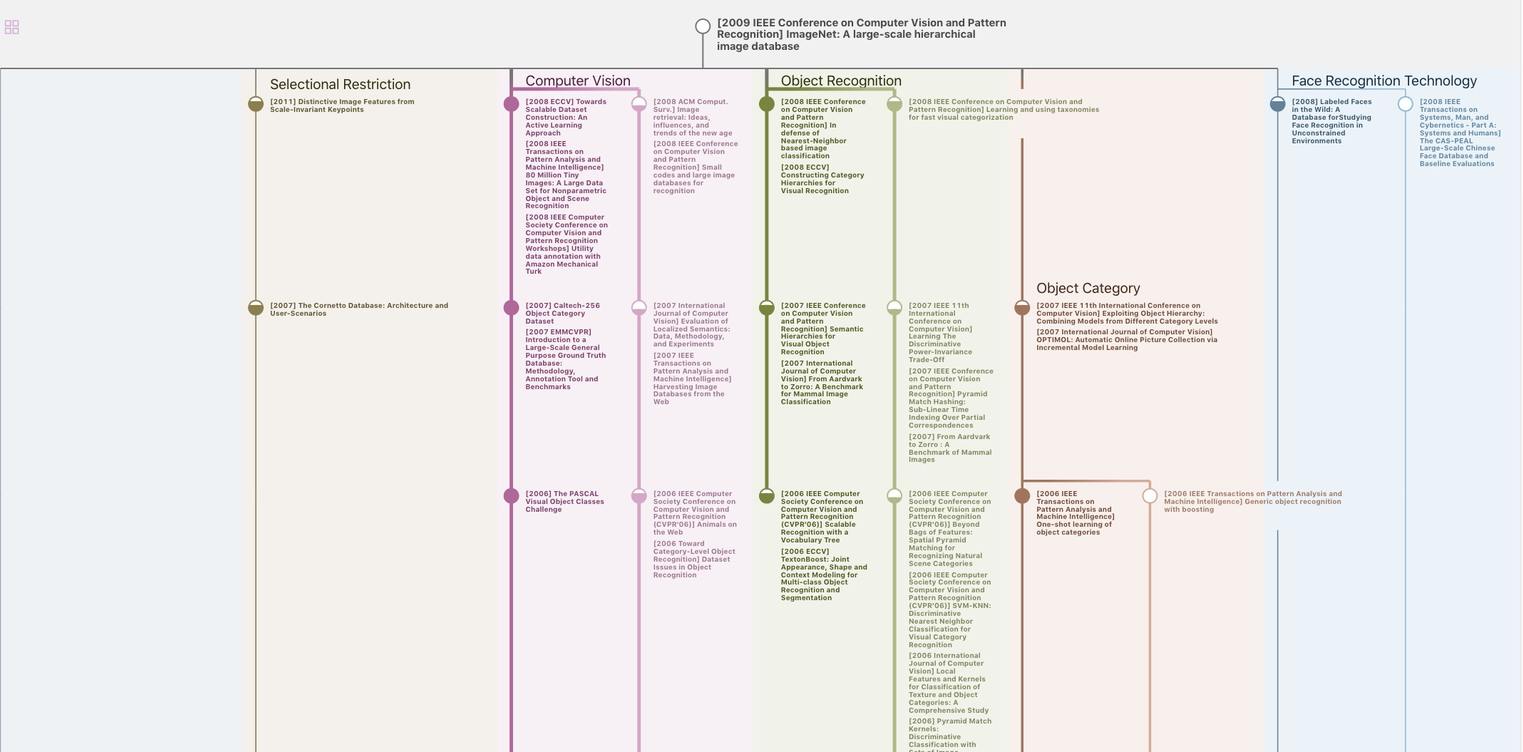
生成溯源树,研究论文发展脉络
Chat Paper
正在生成论文摘要