AHT: A Novel Aggregation Hyper-transformer for Few-Shot Object Detection
PATTERN RECOGNITION AND COMPUTER VISION, PRCV 2023, PT XII(2024)
摘要
Few-shot object detection aims to detect novel objects with few annotated examples and this task has been extensively investigated by meta-learning-based paradigm. However, most of the previous approaches suffer from: 1) Most of the previous methods only perform two-branch interaction in the detection head which lacks the interaction of low-level semantic features. 2) Traditional method is difficult to capture the fine-grained differences between categories due to fixed weights. To alleviate these issues, we proposed a simple yet effective method, named Aggregation Hyper-Transformer (AHT) framework, which can generate corresponding weights into the primary network by hypernetworks mechanism. In particular, we design a novel Dynamic Aggregation Module and a Conditional Adaptation Hypernetworks, which apply the aggregated category vectors as conditions to dynamically generates class-specific parameters. Benefiting from the above two modules, our method significantly exceeds the previous meta-learning methods and provides new insights for my community.
更多查看译文
关键词
Few-shot object detection,Hypernetwork,Meta-learning
AI 理解论文
溯源树
样例
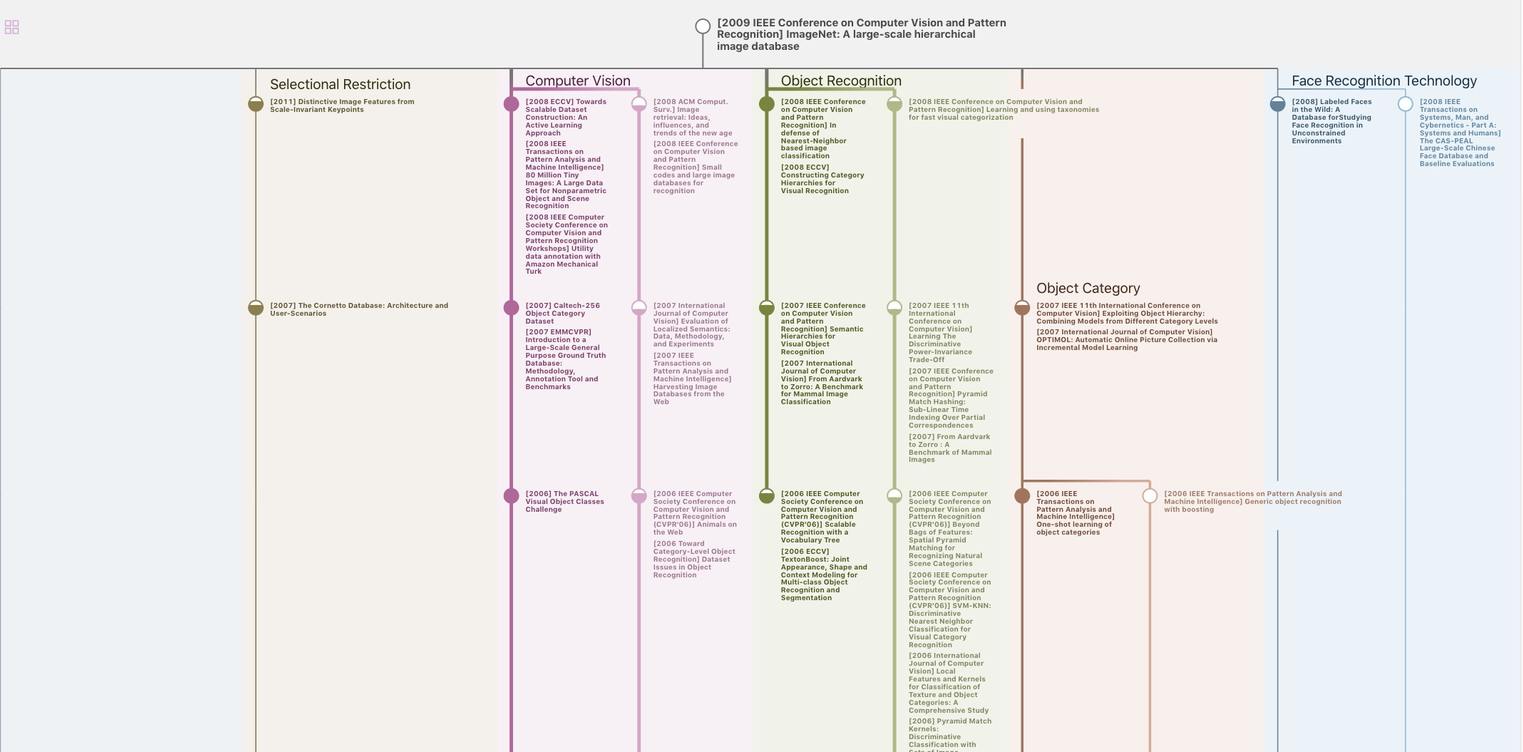
生成溯源树,研究论文发展脉络
Chat Paper
正在生成论文摘要