Feature Disentanglement and Adaptive Fusion for Improving Multi-modal Tracking
PATTERN RECOGNITION AND COMPUTER VISION, PRCV 2023, PT XII(2024)
摘要
Multi-modal tracking has increasingly gained attention due to its superior accuracy and robustness in complex scenarios. The primary challenges in this field lie in effectively extracting and fusing multi-modal data that inherently contain gaps. To address the above issues, we propose a novel regularized single-stream multi-modal tracking framework, drawing inspiration from the perspective of disentanglement. Specifically, taking into account the similarities and differences intrinsic in multi-modal data, we design a modality-specific weights sharing feature extraction module to extract well-disentangled multi-modal features. To emphasize feature-level specificity across different modal features, we propose a cross-modal deformable attention mechanism for the adaptive integration of multi-modal features with efficiency. Through extensive experiments on three multi-modal tracking benchmarks, including RGB+Thermal infrared and RGB+Depth, we demonstrate that our method significantly outperforms existing multi-modal tracking algorithms. Code is available at https://github.com/ccccwb/Multimodal-Detection-and-Tracking-UAV.
更多查看译文
关键词
Visual object tracking,Multi-modal tracking,Multi-modal Fusion,Cross-modal vision transformer
AI 理解论文
溯源树
样例
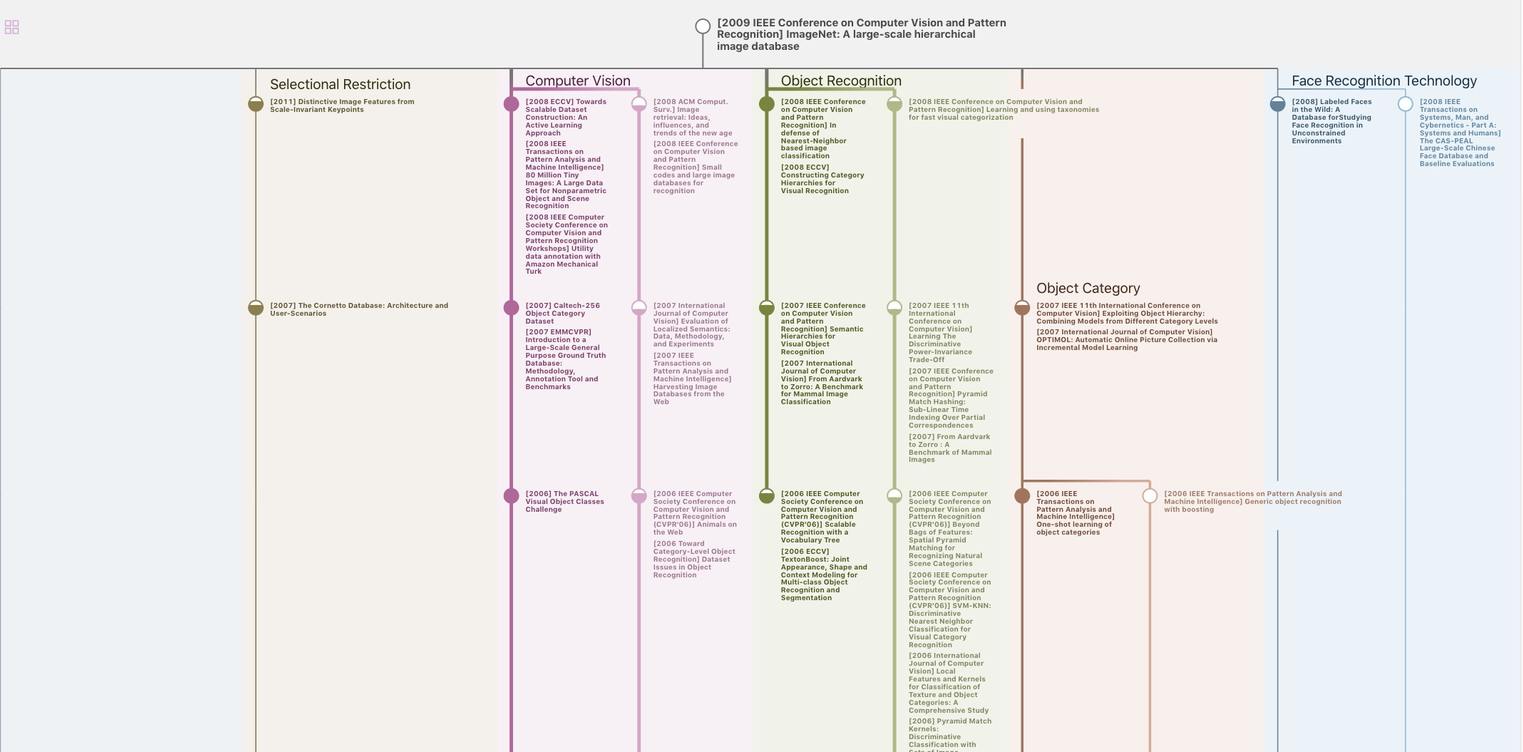
生成溯源树,研究论文发展脉络
Chat Paper
正在生成论文摘要