Unsupervised Image-to-Image Translation with Style Consistency.
Lecture notes in computer science(2023)
摘要
Unsupervised Image-to-Image Translation (UNIT) has gained significant attention due to its strong ability of data augmentation. UNIT aims to generate a visually pleasing image by synthesizing an image's content with another's style. However, current methods cannot ensure that the style of the generated image matches that of the input style image well. To overcome this issue, we present a new two-stage framework, called Unsupervised Image-to-Image Translation with Style Consistency (SC-UNIT), for improving the style consistency between the image of the style domain and the generated image. The key idea of SC-UNIT is to build a style consistency module to prevent the deviation of the learned style from the input one. Specifically, in the first stage, SC-UNIT trains a content encoder to extract the multiple-layer content features wherein the last-layer's feature can represent the abstract domain-shared content. In the second stage, we train a generator to integrate the content features with the style feature to generate a new image. During the generation process, dynamic skip connections and multiple-layer content features are used to build multiple-level content correspondences. Furthermore, we design a style reconstruction loss to make the style of the generated image consistent with that of the input style image. Numerous experimental results show that our SC-UNIT outperforms state-of-the-art methods in image quality, style diversity, and style consistency, even for domains with significant visual differences. The code is available at https://github.com/GZHU-DVL/SC-UNIT.
更多查看译文
关键词
Unsupervised Image-to-Image Translation,Prior Distillation,Generative Adversarial Network,Style Consistency
AI 理解论文
溯源树
样例
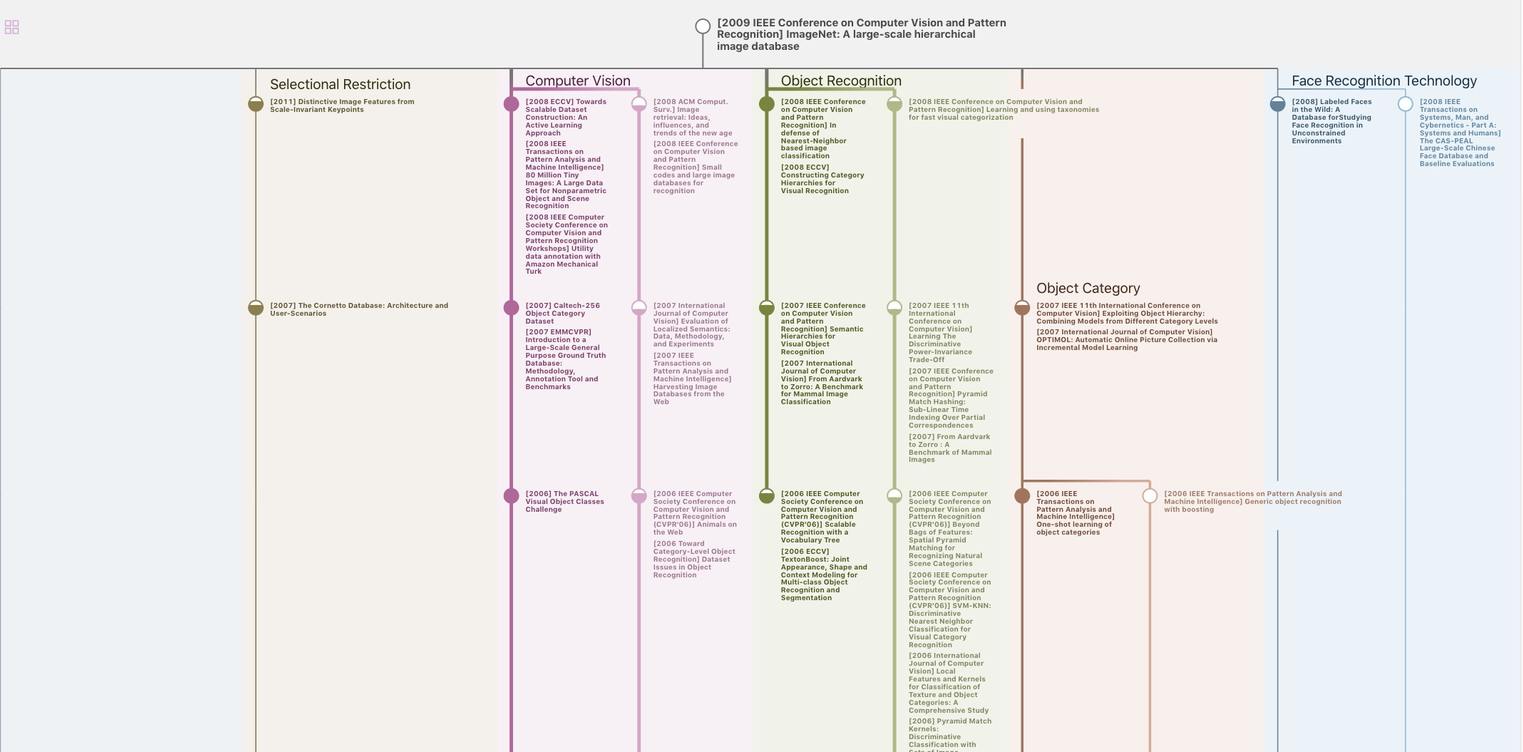
生成溯源树,研究论文发展脉络
Chat Paper
正在生成论文摘要