SemanticCrop: Boosting Contrastive Learning via Semantic-Cropped Views
PATTERN RECOGNITION AND COMPUTER VISION, PRCV 2023, PT VI(2024)
摘要
Siamese-structure-based contrastive learning has shown excellent performance in learning visual representations due to its ability to minimize the distance between positive pairs and increase the distance between negative pairs. Existing works mostly employ RandomCrop or ContrastiveCrop to obtain positive pairs of an image. However, RandomCrop causes the cropped views to contain many useless backgrounds, while ContrastiveCrop produces positive pairs that are too similar. In this paper, we propose a novel SemanticCrop to yield cropped views containing as much semantic information as possible. Specifically, SemanticCrop first computes a heatmap of an image. Then, an empirical threshold is tuned to box out a semantic region whose heatmap values are over this threshold. Finally, we design a center-suppressed probabilistic sampling to avoid excessive similarity between positive pairs, making the cropped view contain more parts of an object. As a plug-and-play module, the MoCo, SimCLR, SimSiam, and BYOL models equipped with our SemanticCrop module achieve an accuracy improvement from 0.5% to 2.34% on the CIFAR10, CIFAR100, IN-200, and IN-1K datasets. The code is available at https://github.com/GZHU-DVL/SemanticCrop.
更多查看译文
关键词
Contrastive learning,Self-supervised learning,Data agumentation
AI 理解论文
溯源树
样例
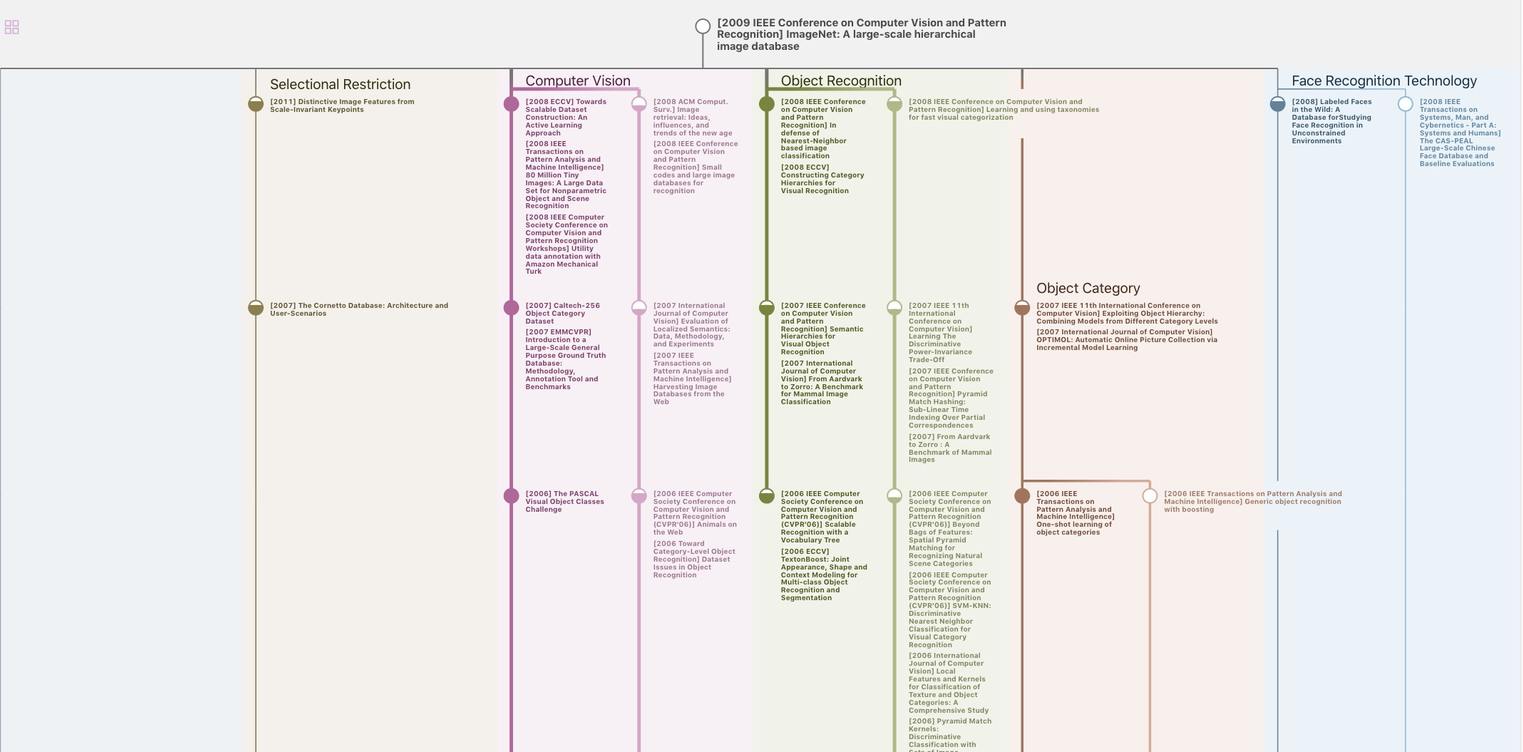
生成溯源树,研究论文发展脉络
Chat Paper
正在生成论文摘要