Quality-Aware CLIP for Blind Image Quality Assessment
PATTERN RECOGNITION AND COMPUTER VISION, PRCV 2023, PT VI(2024)
摘要
Blind Image Quality Assessment (BIQA) aims to simulate human perception of image quality without reference images. Pretrained visual-linguistic models, like CLIP, have shown excellent performance in various visual tasks and have been successfully applied in BIQA. However, existing CLIP-based approaches typically employ a coarse classification method, dividing images into two or five quality levels based on CLIP's text-image comparison ability. In this work, we propose a novel approach for BIQA that introduces a fine-grained quality-level stratification strategy. This strategy enables a more precise assessment of image quality across a wider range of levels. Additionally, we present a two-stage training model called Quality-Aware CLIP (QA-CLIP). In the first stage, we leverage a set of learnable text tokens to optimize the text description and fully utilize the representation capabilities of CLIP's text encoder. In the second stage, we further optimize the image encoder and quality-aware block to capture features that are highly relevant to perceived quality. Experimental results demonstrate that QA-CLIP achieves comparable performance with state-of-the-art methods on various synthetic and real datasets. Notably, in CSIQ, TID2013, and KADID datasets, QA-CLIP outperforms the state-of-the-art by 1.2%, 4.7%, and 4.8% respectively in terms of Spearman Rank Correlation Coefficient (SRCC).
更多查看译文
关键词
Blind Image Quality Assessment,CLIP,Quality-Aware,Learnable Text Tokens
AI 理解论文
溯源树
样例
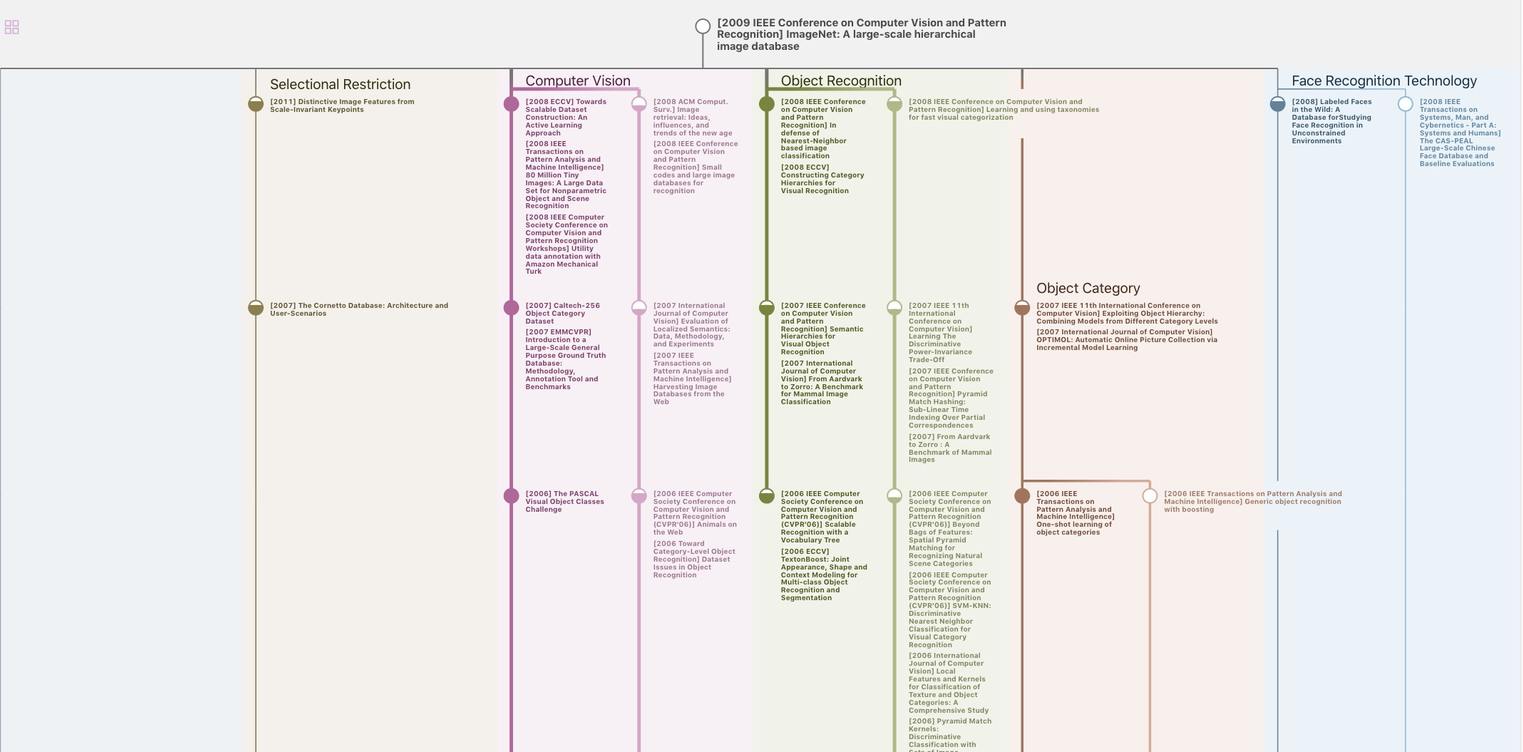
生成溯源树,研究论文发展脉络
Chat Paper
正在生成论文摘要