CorrFormer: Context-aware tracking with cross-correlation and transformer
COMPUTERS & ELECTRICAL ENGINEERING(2024)
摘要
The fusion of the template and search region features plays a significant role in deep learningbased trackers. In Siamese -based trackers, different cross -correlation operations are commonly used to fuse features, which cannot obtain global connections. On the other hand, transformerbased trackers use attention mechanism to fuse features, which cannot suppress the interference of distractors in the background. Furthermore, existing trackers use regression and classification heads with the same structure, which leads to lack a deeper understanding of these two different tasks. To address these problems, we firstly propose a feature enhancement -fusion network (FEFN) based on cross -correlation and transformer, with two Encoders that employ self -attention and a Decoder that removes cross -attention to adapt to the tracking task. Using the FEFN to combine the advantages of Siamese -based and transformer -based trackers, our tracker establishes global connections while effectively suppressing the distractors. We also propose a novel decoupled head, designing a spatial sensitive classification head and a global information sensitive regression head, which helps the context -aware tracker locate the target more accurately. Our proposed tracker obtains 0.710 of AO, 0.814 of SR0.5 and 0.657 of SR0.75 on the GOT -10k test set, and achieves real-time requirement at 36.99FPS.
更多查看译文
关键词
Object tracking,Cross-correlation,Transformer,Decoupled head
AI 理解论文
溯源树
样例
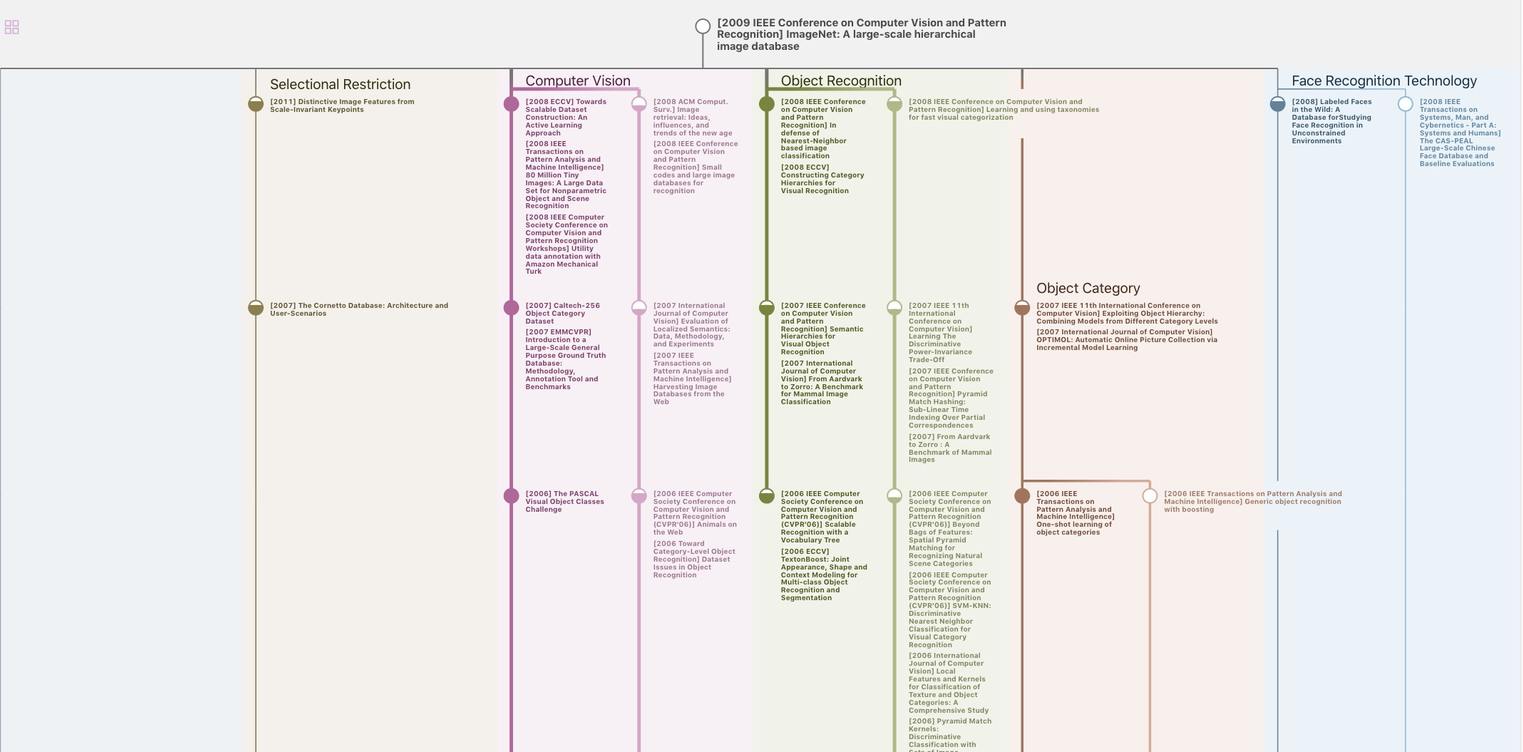
生成溯源树,研究论文发展脉络
Chat Paper
正在生成论文摘要