A novel stock trading utilizing long short term memory prediction and evolutionary operating-weights strategy
EXPERT SYSTEMS WITH APPLICATIONS(2024)
摘要
In the realm of quantitative investing, predicting stock prices and developing effective trading strategies are paramount. The inherent randomness of the stock market, however, limits the precision of stock price predictions, thus undermining investment performance when these predictions are the sole basis for strategy. Nonetheless, forecasting future prices can offer invaluable insights for strategic models. When predictions are paired with well-designed strategies, they can augment investment returns. This paper proposes a novel approach to enhance investment returns by integrating Long Short Term Memory (LSTM) predictions with the Evolutionary Operating-weights (EOW) algorithmic strategy. The proposed method employs a multi-layer LSTM to forecast future stock prices, incorporating the predictions with real market data, subsequently deriving an operational strategy using the EOW algorithm. The results of this research indicate that this proposed methodology outperforms existing approaches.
更多查看译文
关键词
Evolutionary operating-weights,Long short term memory,Evolutionary algorithms,Reinforcement learning,Quantitative investment
AI 理解论文
溯源树
样例
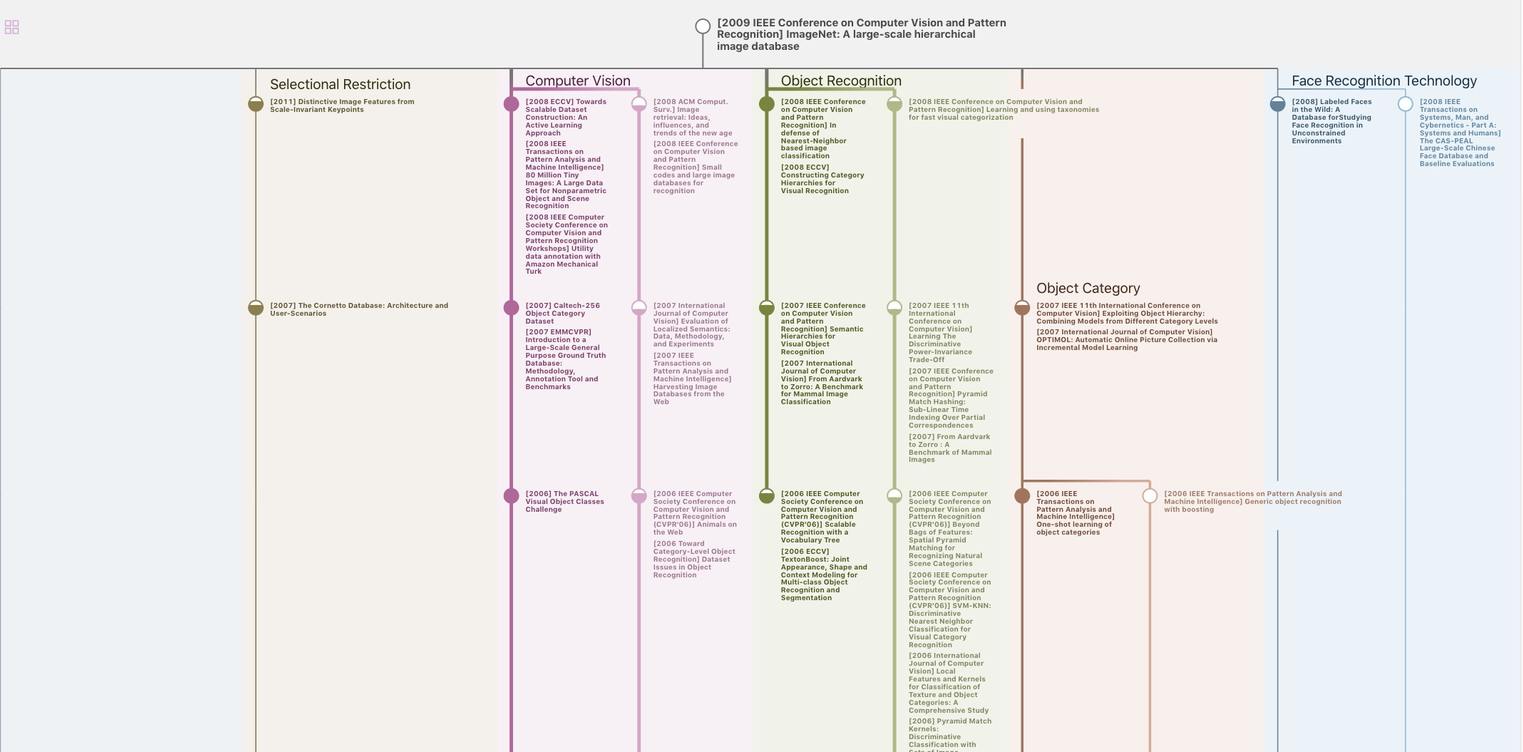
生成溯源树,研究论文发展脉络
Chat Paper
正在生成论文摘要