A Combinatorial Optimization Analysis Method for Detecting Malicious Industrial Internet Attack Behaviors
ACM TRANSACTIONS ON CYBER-PHYSICAL SYSTEMS(2024)
摘要
Industrial Internet plays an important role in key critical infrastructure sectors and is the target of different security threats and risks. There are limitations in many existing attack detection approaches, such as function redundancy, overfitting, and low efficiency. A combinatorial optimization method-Lagrange multiplier-is designed to optimize the underlying feature screening algorithm. The optimized feature combination is fused with random forest and XG-Boost selected features to improve the accuracy and efficiency of attack feature analysis. Using both the UNSW-NB15 and natural gas pipeline datasets, we evaluate the performance of the proposed method. It is observed that the influence degrees of the different features associated with the attack behavior can result in the binary classification attack detection increasing to 0.93 and the attack detection time reducing by 6.96 times. The overall accuracy of multi-classification attack detection is also observed to improve by 0.11. We also observe that nine key features of attack behavior analysis are essential to the analysis and detection of general attacks targeting the system, and by focusing on these features one could potentially improve the effectiveness and efficiency of real-time critical industrial system security. In this article, the CICDDoS2019 and CICIDS2018 datasets are used to prove the generalization. The experimental results show that the proposed method has good generalization and can be extended to the same type of industrial anomaly datasets.
更多查看译文
关键词
Industrial Internet,industrial situational security,attack behavior,feature analysis,combinatorial optimization
AI 理解论文
溯源树
样例
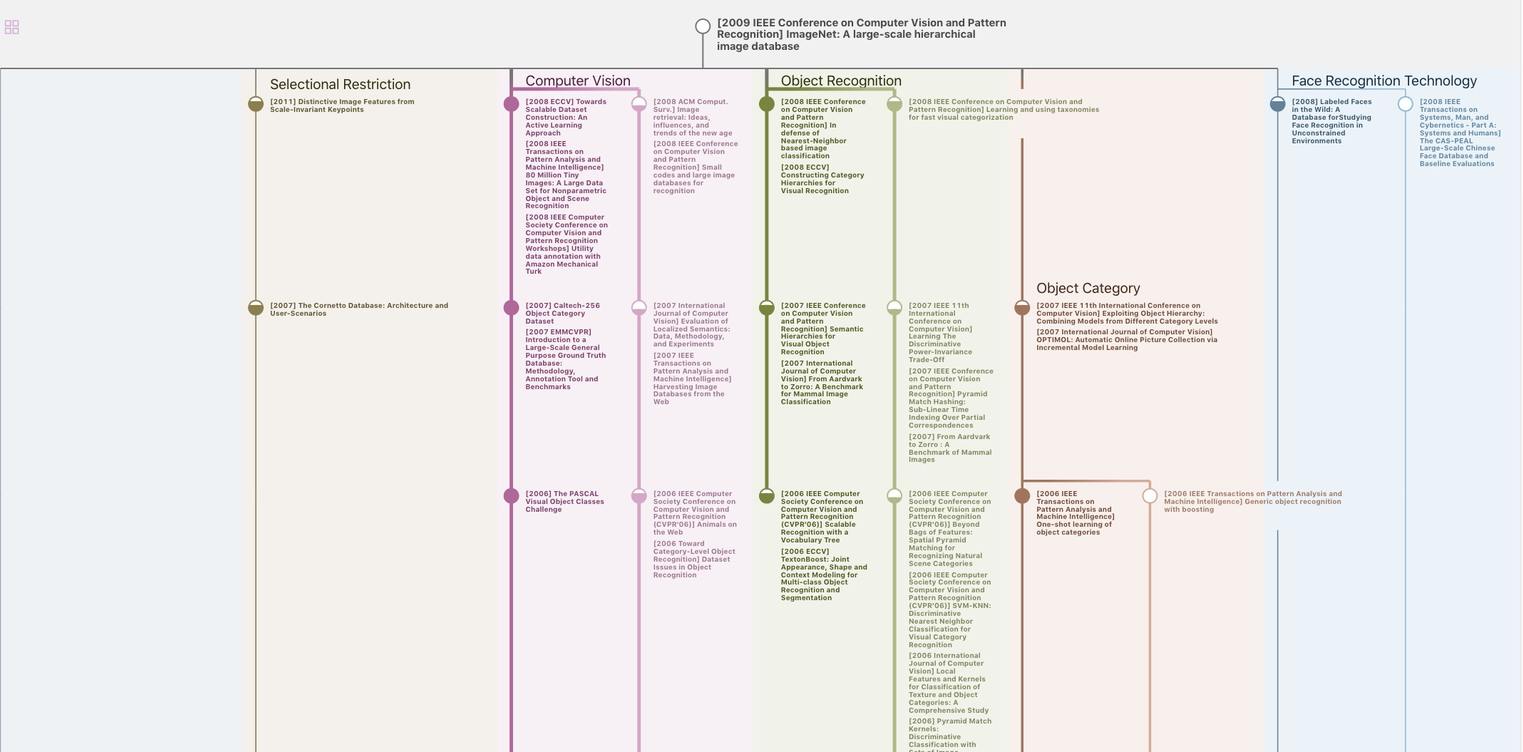
生成溯源树,研究论文发展脉络
Chat Paper
正在生成论文摘要