HADGSM: A Unified Nonconvex Framework for Hyperspectral Anomaly Detection
IEEE TRANSACTIONS ON GEOSCIENCE AND REMOTE SENSING(2024)
摘要
Hyperspectral anomaly detection aims at distinguishing targets of interest from the background without prior knowledge. Although low-rank representation (LRR)-based methods have been broadly applied in anomaly detection tasks, how to approximate the penalties in LRR-based methods more precisely is still a problem that needs to be further investigated. To this end, this article designs a unified nonconvex framework called hyperspectral anomaly detection via generalized shrinkage mappings (HADGSMs) to better approximate the LRR-based methods. The core of the proposed framework is to design new nonconvex penalties to approximate the group sparsity, $l_{0}$ gradient, and low-rankness penalties in the LRR-based anomaly detection models, which can be efficiently minimized by means of generalized shrinkage mappings (GSMs). Then, an efficient alternating direction method of multipliers (ADMM) is developed to handle the proposed model. Experiments conducted on several real hyperspectral datasets demonstrate the superiority and effectiveness of the proposed framework in enhancing detection performance with respect to state-of-the-art methods.
更多查看译文
关键词
Hyperspectral imaging,Anomaly detection,Task analysis,Image reconstruction,Tensors,Dictionaries,Detectors,generalized shrinkage mappings (GSMs),hyperspectral image,low-rank representation (LRR),total variation (TV)
AI 理解论文
溯源树
样例
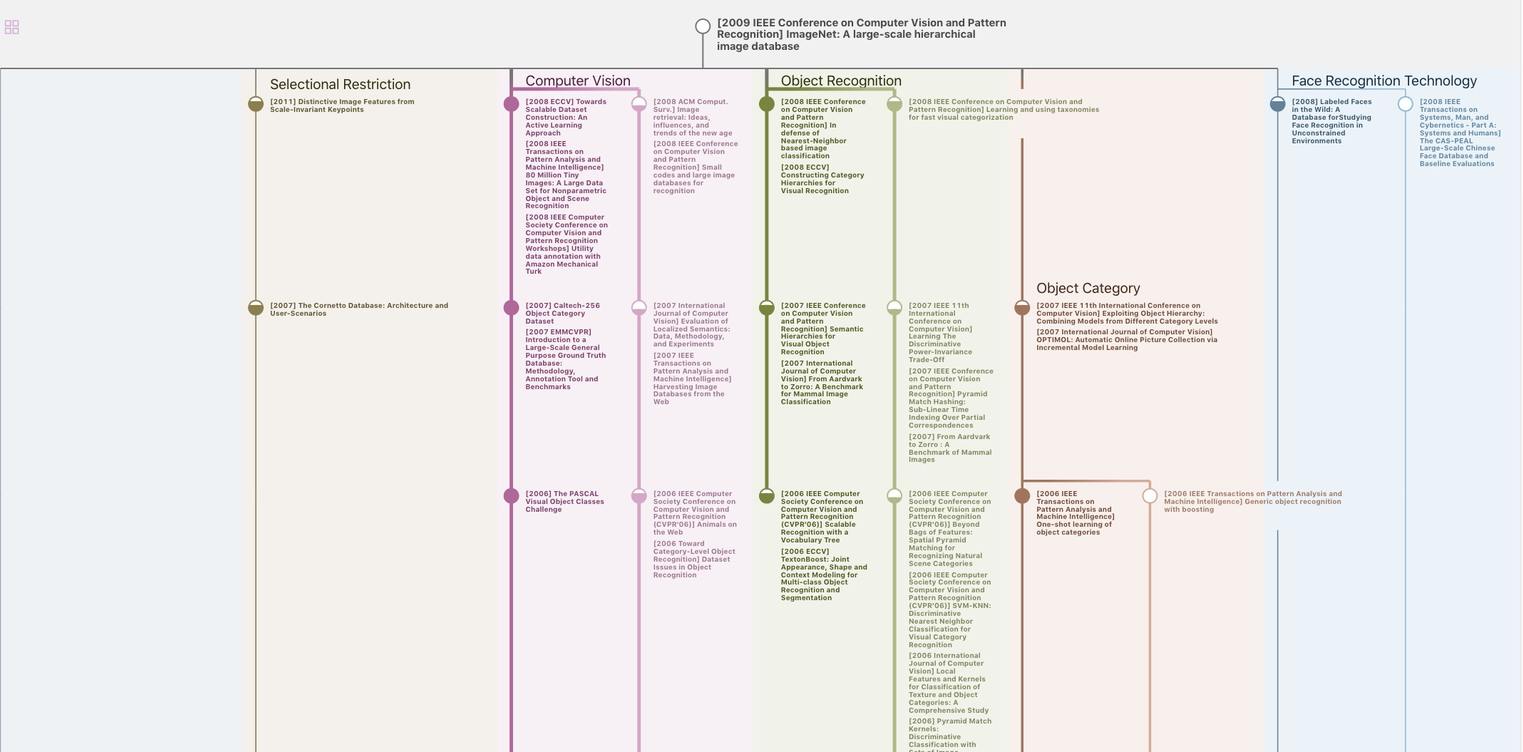
生成溯源树,研究论文发展脉络
Chat Paper
正在生成论文摘要