Universal unsupervised cross-domain 3D shape retrieval
Multimedia Systems(2024)
摘要
Most existing cross-domain 3D shape retrieval (CD3DSR) methods have assumed the setting of a fixed kind of query set (source domain), and all the annotated query data follow the same distribution. However, in practical scenarios, the labelled query sets are typically collected from multiple sources. In such scenarios, single-source CD3DSR methods may fail because of the domain shift across different sources, and universal CD3DSR methods are needed. In this paper, we propose a novel universal unsupervised domain adaptation network (U ^2 DAN). It mainly consists of two modules: cross-domain statistics alignment (CDSA) and source-domain feature adaptation (SDFA). First, we use 2D CNN to encode the query and 3D shape from the gallery (target domain) to obtain visual features. To mix up the features between each source and target domain pair, we introduce the margin disparity discrepancy (MDD) model to enforce the domain alignment in an adversarial way. Since the domain shifts also exist across different sources, which may result in performance degradation, we introduce two kinds of discriminators, source-domain discriminator, and cycle cross-domain discriminator to reduce source domain bias. Further, considering there are no available 3D datasets for evaluation, we constructed two novel datasets, MS3DOR-1 for universal cross-dataset 3D shape retrieval (3D-to-3D) and MS3DOR-2 for universal cross-modal 3D shape retrieval (2D-to-3D). Extensive comparisons on two datasets can verify the effectiveness of U ^2 DAN against the state-of-art methods.
更多查看译文
关键词
3D shape retrieval,Cross-domain retrieval,Multi-view representation learning,Multi-source domain adaptation
AI 理解论文
溯源树
样例
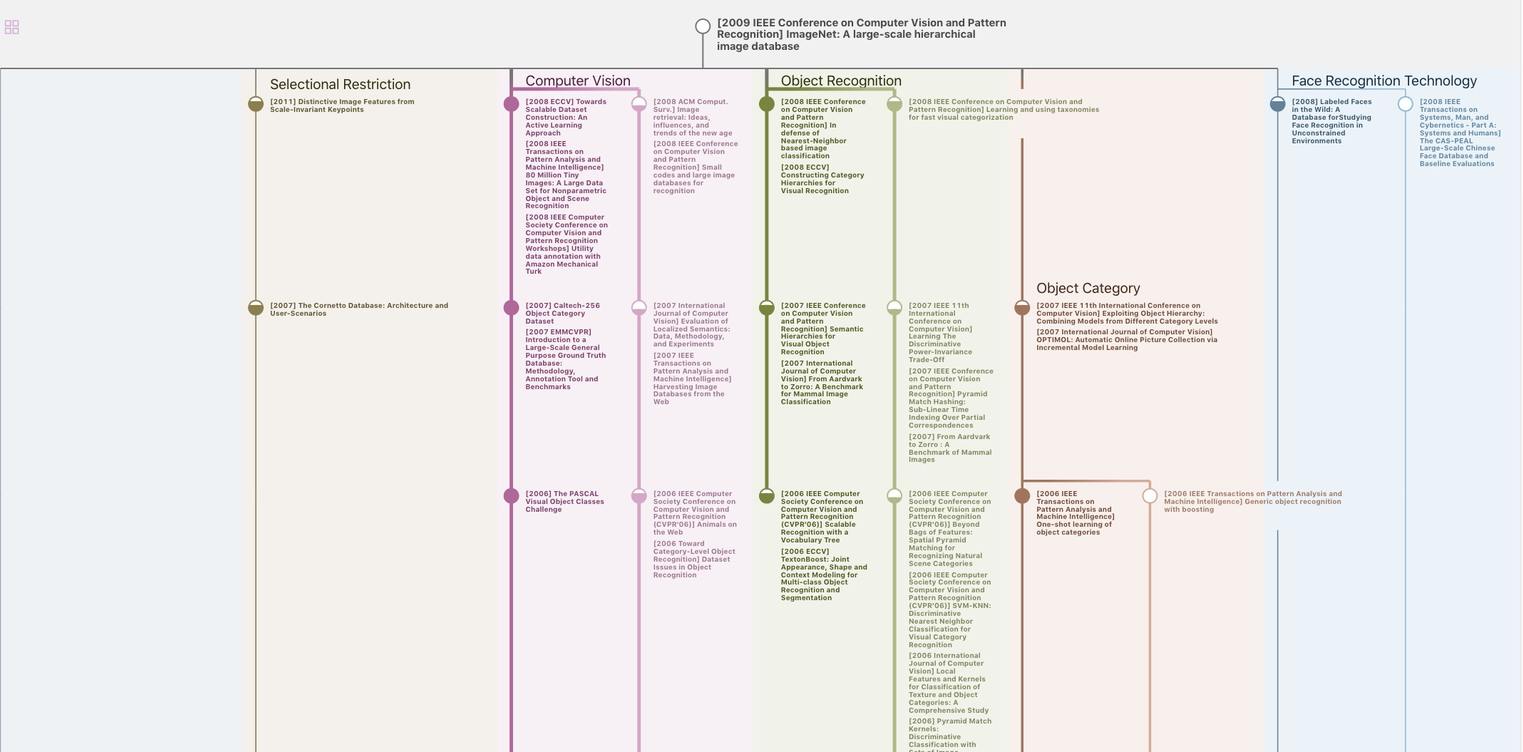
生成溯源树,研究论文发展脉络
Chat Paper
正在生成论文摘要