Predicting Loneliness from Subject Self-Report
2023 11TH INTERNATIONAL CONFERENCE ON AFFECTIVE COMPUTING AND INTELLIGENT INTERACTION, ACII(2023)
摘要
In this work, we propose to predict four items from the UCLA loneliness scale using subject self-report scores. Using subjective self-reporting, over 14 days, for positive and negative affect, and depression and anxiety we evaluate both subject dependent (personalized) and subject independent experimental design. We evaluate four approaches for prediction, namely random forest, support vector machine, k-nearest neighbor, and logistic regression. We find that the features (self-report) are relatively stable across all four approaches. Along with each individual self-report feature, we also evaluate the fusion of all features where they are concatenated into one feature vector. Through our experimental design, we show that UCLA loneliness items can be predicted, and that the fusion of features (positive and negative affect, and depression and anxiety) is the most encouraging way to do this prediction. We also show that the number of days, used for prediction, has a noticeable impact on the results and that personalization helps with prediction.
更多查看译文
关键词
loneliness,affect,machine learning
AI 理解论文
溯源树
样例
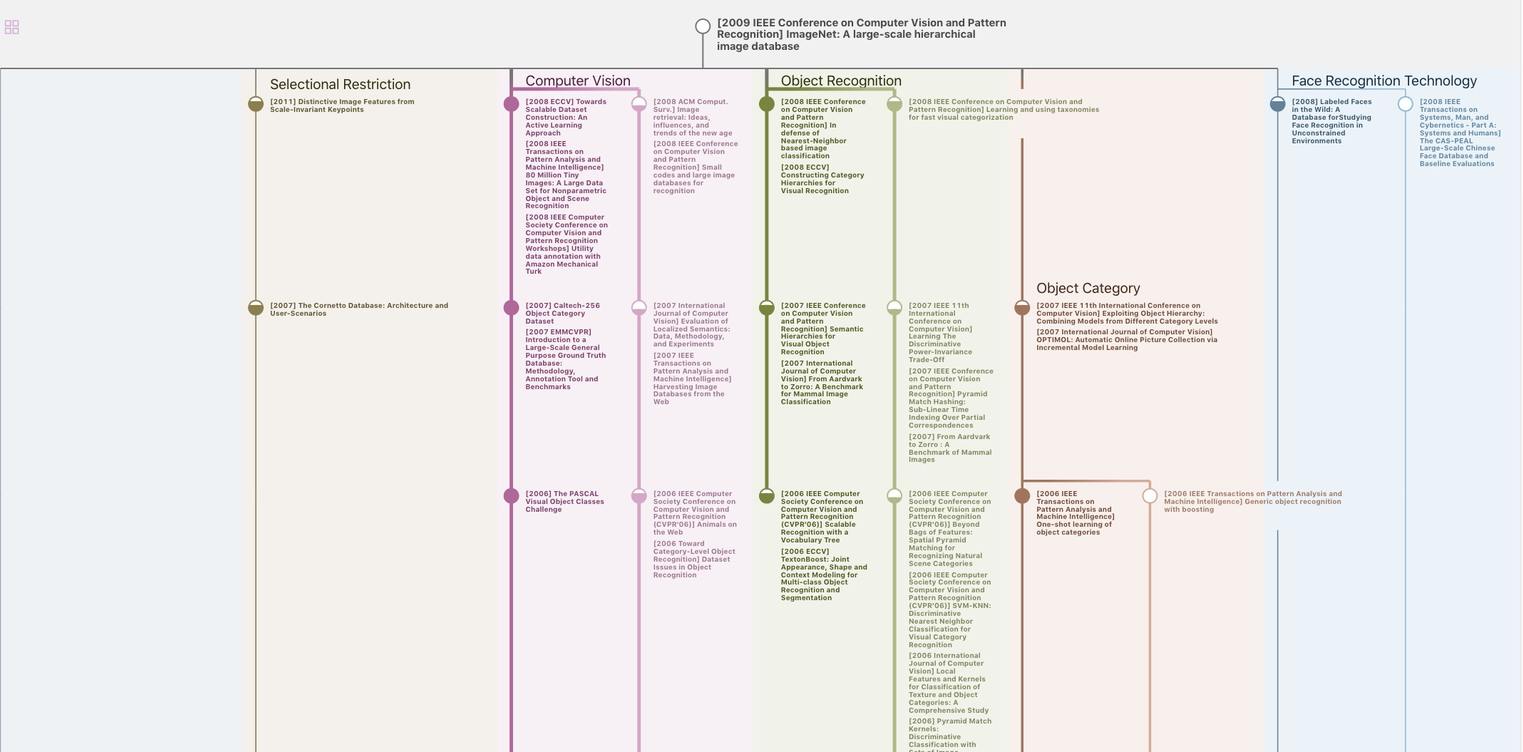
生成溯源树,研究论文发展脉络
Chat Paper
正在生成论文摘要