Gaze and Head Movement Patterns of Depressive Symptoms During Conversations with Emotional Virtual Humans
2023 11TH INTERNATIONAL CONFERENCE ON AFFECTIVE COMPUTING AND INTELLIGENT INTERACTION, ACII(2023)
摘要
Depressive symptoms involve dysfunctional social attitudes and heightened negative emotional states. Identifying biomarkers requires data collection in realistic environments that activate depression-specific phenomena. However, no previous research analysed biomarkers in combination with AI-powered conversational virtual humans (VH) for mental health assessment. This study aims to explore gaze and head movements patterns related to depressive symptoms during conversations with emotional VH. A total of 105 participants were evenly divided into a control group and a group of subjects with depressive symptoms (SDS). They completed six semi-guided conversations designed to evoke basic emotions. The VHs were developed using a cognitive-inspired framework, enabling real-time voice-based conversational interactions powered by a Large Language Model, and including emotional facial expressions and lip synchronization. They have embedded life-history, context, attitudes, emotions and motivations. Signal processing techniques were applied to obtain gaze and head movements features, and heatmaps were generated. Then, parametric and non-parametric statistical tests were applied to evaluate differences between groups. Additionally, a two-dimensional t-SNE embedding was created and combined with k-means clustering. Results indicate that SDS exhibited shorter blinks and longer saccades. The control group showed affiliative lateral head gyros and accelerations, while the SDS demonstrated stress-related back-and-forth movements. SDS also displayed the avoidance of eye contact. The exploratory multivariate statistical unsupervised learning achieved 72.3% accuracy. The present study analyse biomarkers in affective processes with multiple social contextual factors and information modalities in ecological environments, and enhances our understanding of gaze and head movements patterns in individuals with depressive symptoms, ultimately contributing to the development of more effective assessments and intervention strategies.
更多查看译文
关键词
depressive disorder,virtual human,emotion elicitation,clustering,statistical learning,large language models,mental health
AI 理解论文
溯源树
样例
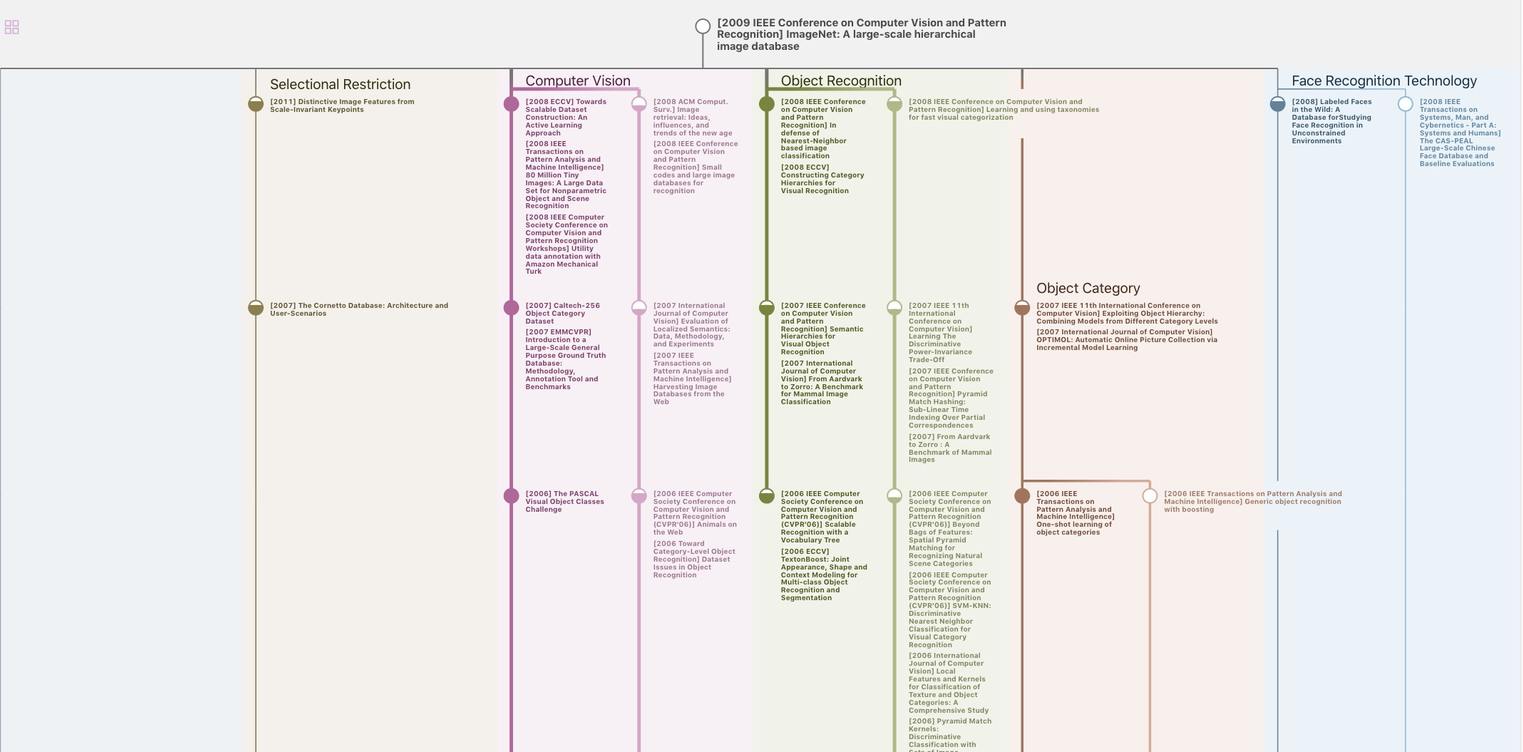
生成溯源树,研究论文发展脉络
Chat Paper
正在生成论文摘要