RefineHD: Accurate and Efficient Single-Pass Adaptive Learning Using Hyperdimensional Computing.
2023 IEEE International Conference on Rebooting Computing (ICRC)(2023)
摘要
Hyperdimensional computing (HDC) is a computing framework that has gained significant attention due to its high efficiency and rapid training and inference of machine learning algorithms [1]. With its fast learning and inference capabilities, HDC shows excellent potential for IoT/Embedded systems. However, while HDC allows for fast single-pass learning [2], it suffers from weak classification accuracy, resulting from model saturation caused by excessive noise due to the addition of similar patterns. In this paper, we propose an adaptive learning method that surpasses accuracy and robustness compared to the state-of-the-art adaptive HDC model, while maintaining the same efficiency during both training and testing phases. Our method addresses the issue of saturation by selectively adding correctly classified samples only when their similarity to the existing patterns sufficiently differs from the class. Moreover, we achieve a robust model against noise and hardware failures, thanks to its HDC holographic properties. Through this approach, we achieve a remarkable average accuracy improvement of +2.8% across 126 datasets (with a maximum improvement of +26%). Furthermore, we observe a remarkable +6.6% improvement over the HDC baseline (with a maximum improvement of +67%), all while retaining the same inference efficiency.
更多查看译文
AI 理解论文
溯源树
样例
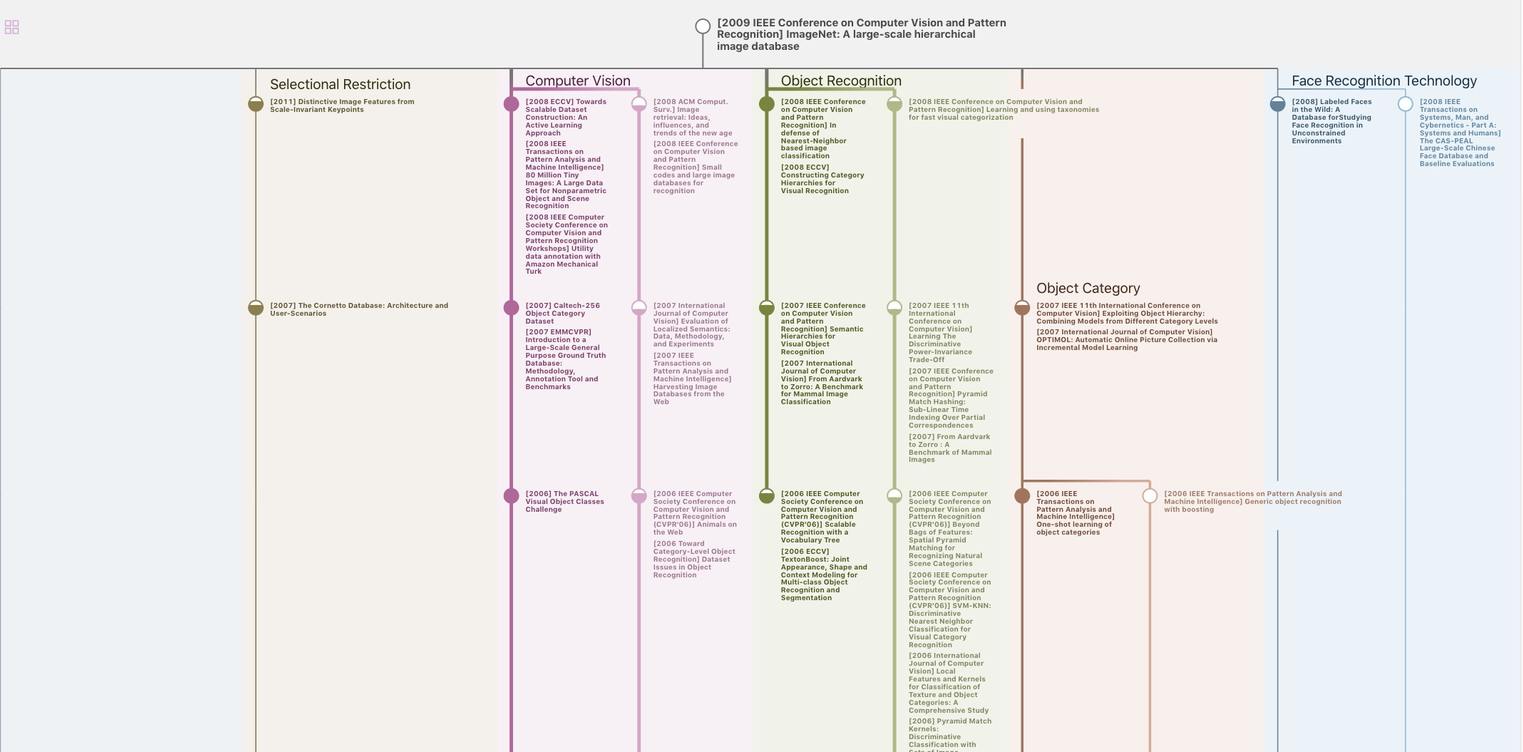
生成溯源树,研究论文发展脉络
Chat Paper
正在生成论文摘要