A Novel Method for Ransomware Family Detection on Deep Learning-based Models Using Adversarial Examples
2023 IEEE 5th Eurasia Conference on IOT, Communication and Engineering (ECICE)(2023)
摘要
The rapidly growing number of ransomware variants in recent years has led to the development of detectors based on deep learning against malware threat detection. However, hackers have introduced adversarial examples (AEs) to deceive deep learning detection models, resulting in a significant reduction in classification accuracy. These perturbed signals are deliberately chosen adversarial attack signals, but machine learning models learn feature signals and make erroneous classification judgments. By combining Cuckoo sandbox analysis with four types of sequential databased deep neural networks, we created a mechanism for ransomware detection and classification. By capturing behavioral features of ransomware, filtering, and employing an LSTM model in the learning process, the optimal parameters of the models (hyperparameter) were analyzed to detect different types of ransomware families and their variants. During the model validation phase, adversarial training on Carlini and Wagner (CW)) attacks involving AEs were employed for deep learning models on attack sequence data from multiple ransomware families. Experimental results demonstrated that employing adversarial training enhanced the robustness of deep learning detection models and showed the capability of this ransomware detection model to counter adversarial examples and handle possible zero-day attacks.
更多查看译文
关键词
ransomware,adversarial attack,adversarial examples,zero-day attacks,LSTM
AI 理解论文
溯源树
样例
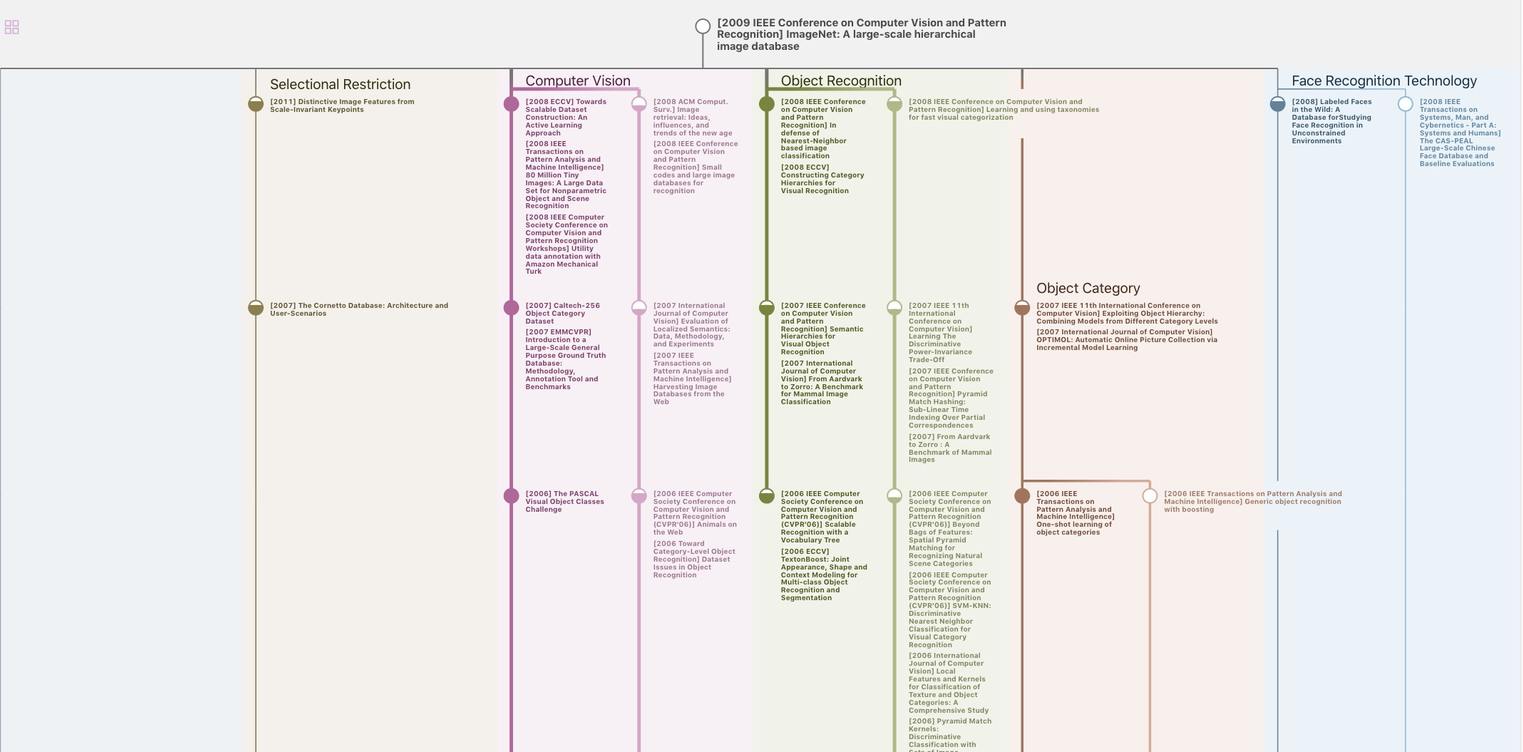
生成溯源树,研究论文发展脉络
Chat Paper
正在生成论文摘要