A Novel Surface Electromyographic Gesture Recognition Using Discrete Cosine Transform-Based Attention Network
IEEE SIGNAL PROCESSING LETTERS(2024)
摘要
This study introduces a novel frequency-based attention network (FANet) designed to acquire frequency information in surface electromyographic signals (sEMG) while mitigating potential high-frequency disturbances. The utilization of the discrete cosine transform (DCT) in dedicated attention modules, enables the conversion of sEMG features to the frequency domain and facilitating the extraction of spectral characteristics. This attention module replaces the self-attention module within the multilayer perceptron (MLP) based transformer, offering a streamlined means for internal frequency features analysis in deep learning models. In evaluation, we focus on the dominance of the "rest" gesture in available NinaPro DB4 and NinaPro DB5 datasets. Therefore we employ common metrics tailored to the class-imbalanced hand gesture classification task. Through comprehensive experiments and ablation studies, the results showed significant improvement in hand gesture recognition, with the DCT-based attention modules achieve accuracies of 78.70% and 89.64% in the NinaPro DB4 and DB5 datasets, respectively, while maintaining low computational cost with log-linear complexity.
更多查看译文
关键词
Frequency-based attention,hand gesture recognition,MLP transformer,surface electromyographic (sEMG)
AI 理解论文
溯源树
样例
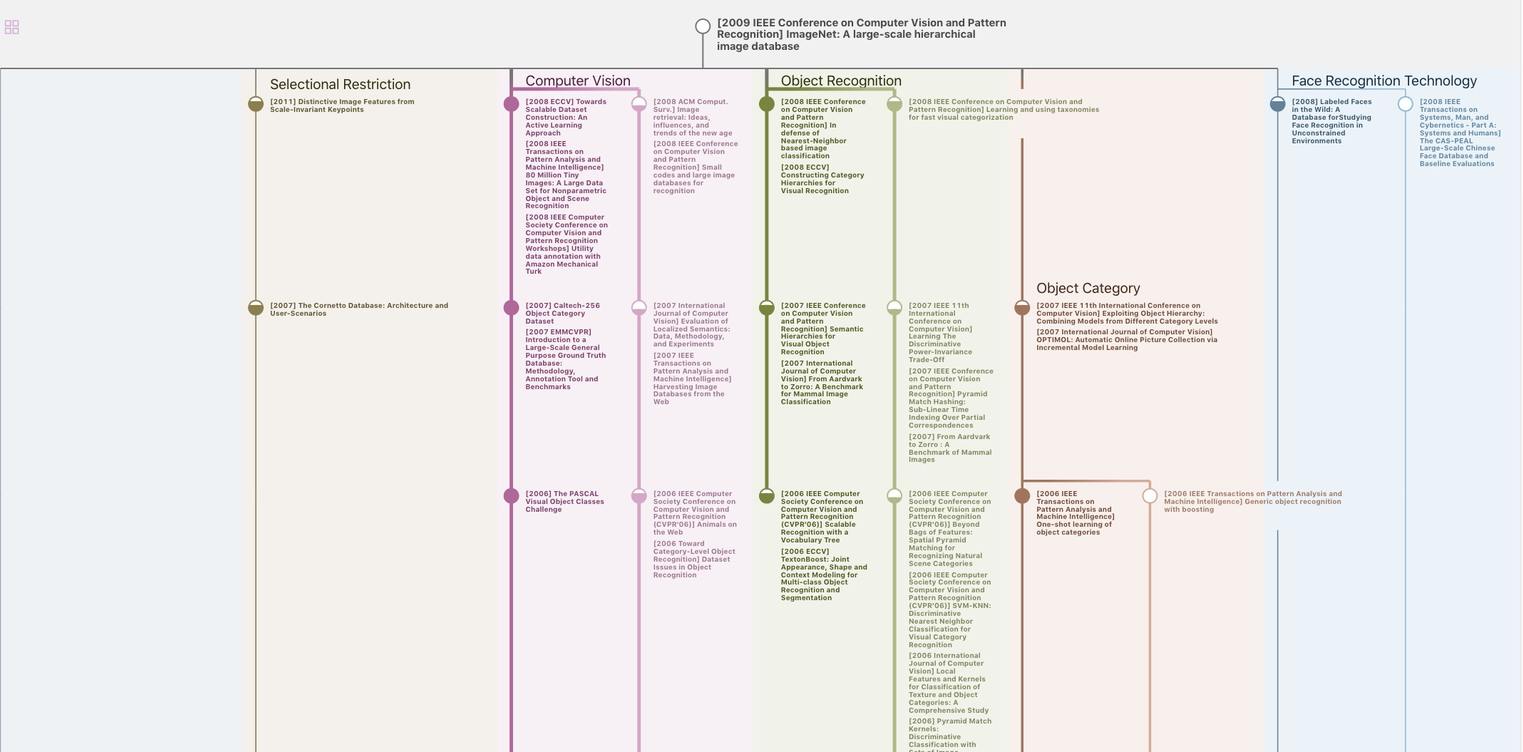
生成溯源树,研究论文发展脉络
Chat Paper
正在生成论文摘要