OrbitDANN: A Mechanism-Informed Transfer Learning Method for Automatic Fault Diagnosis of Turbomachinery
IEEE SENSORS JOURNAL(2024)
摘要
The traditional feature-based fault diagnosis methods for turbomachinery largely rely on manual extraction and selection of features. However, this introduces additional uncertainties into the modeling process. Furthermore, practical applications often suffer from insufficient sensor data for specific fault modes, resulting in inaccurate diagnosis results. This article presents a mechanism-informed adversarial transfer learning approach integrated with domain-adversarial neural network (DANN) for turbomachinery fault diagnosis. It aims to enhance the diagnosis accuracy even when only a small amount of sensor data is available. This approach seamlessly integrates physics-embedded axis orbits corresponding to various fault modes in different turbomachines with the DANN model, so-called orbit-based DANN (OrbitDANN). We have developed a generalized procedure to implement this proposed method for automatic fault diagnosis in various turbomachines. Orbit images are generated from both simulated signals and measured sensor data to illustrate the accuracy and feasibility of the proposed model. Parametric sensitivity analysis is conducted to establish an optimal model for fault diagnosis. In a comparative study with feature-based back-propagation and convolutional neural network (CNN) models using a limited amount of real-world data, we demonstrate the advantages of the proposed model and procedure. This study offers a promising approach to constructing a general artificial intelligence (AI) model to support predictive maintenance of turbomachinery, even when only a small amount of actual sensor data is available.
更多查看译文
关键词
Orbits,Fault diagnosis,Sensors,Feature extraction,Vibrations,Rotors,Transfer learning,Orbit images,domain-adversarial neural network (DANN),adversarial transfer learning,fault diagnosis,turbomachine
AI 理解论文
溯源树
样例
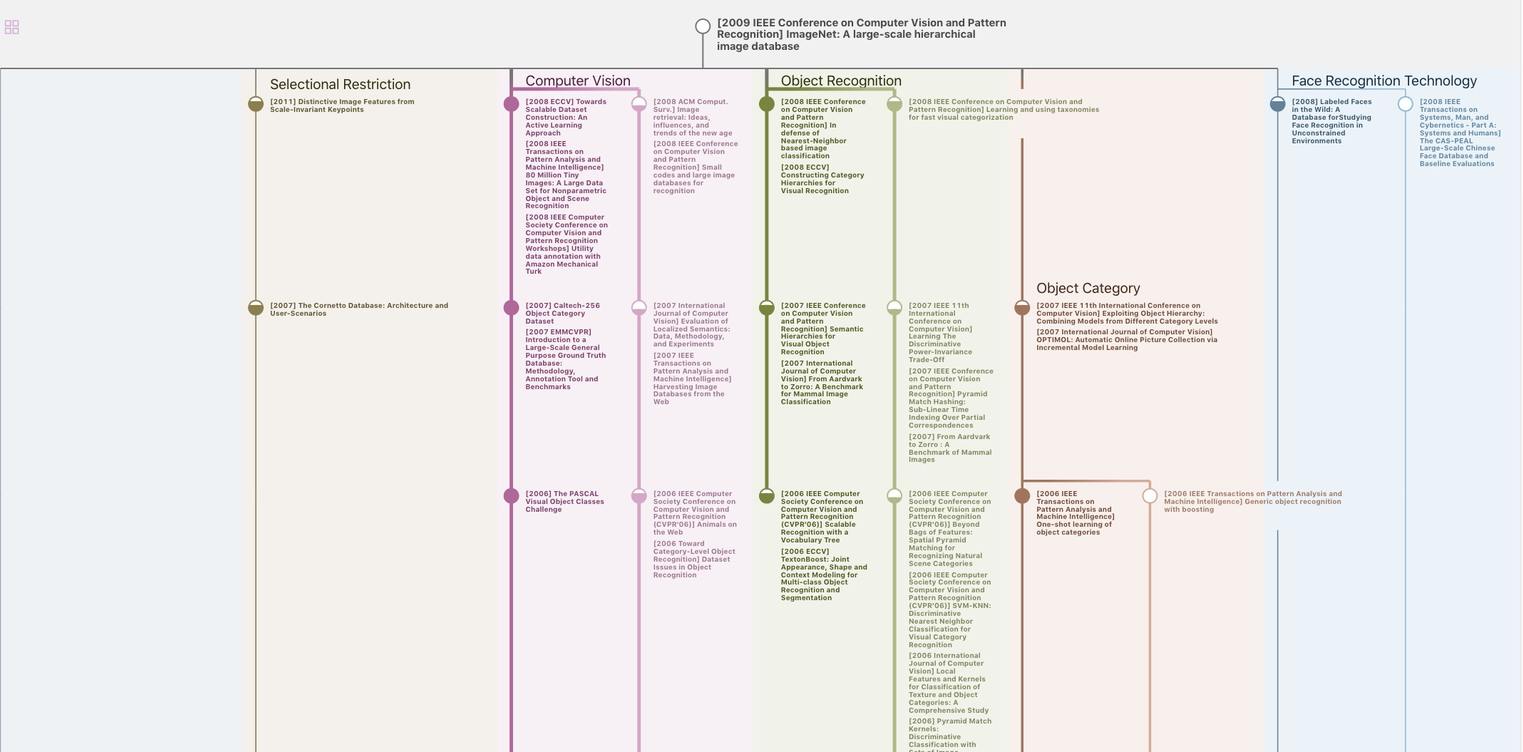
生成溯源树,研究论文发展脉络
Chat Paper
正在生成论文摘要