Screening and Designing of a Large Chemical Space of Organic Semiconductors for Photodetectors
Materials Today Communications(2024)
摘要
The computational approaches show immense potential for rapid discovery, screening, and rational designing of high-performance materials with tailored properties by providing guidance about candidate material for synthesis. Machine learning, an artificial intelligence technology-based approach, provides efficient exploration of high throughput materials as compared to expensive quantum chemical calculations. In this work, machine learning is applied to screen large chemical space of organic semiconductors for aiding the material designing for photodetectors. The key parameters such as band gap and UV/visible absorption maxima of organic semiconductors, which can strongly affect their photodetector properties are predicted using trained machine learning models. Importantly, the hist gradient boosting regression model shows good predictive capability. The Breaking Retrosynthetically Interesting Chemical Substructures (BRICS) method is used to design 10,000 monomers using RDkit. Moreover, the clustering of monomers in generated chemical space is performed. In addition, Silhouette plot and t-distributed stochastic neighbor embedding (t-SNE) are constructed for complex data analysis and visualization. Property prediction is also conducted by considering crucial parameters of different monomers including band gaps, λmax, and synthetic accessibility scores. Similarity analysis is also performed using clustering of monomers and constructing heatmap of similarity in monomers. This methodology may help experimentalists select attractive synthetic targets, which may accelerate the development of photodetectors.
更多查看译文
关键词
Photodetector,Machine learning,Chemical space,Clustering,Similarity analysis
AI 理解论文
溯源树
样例
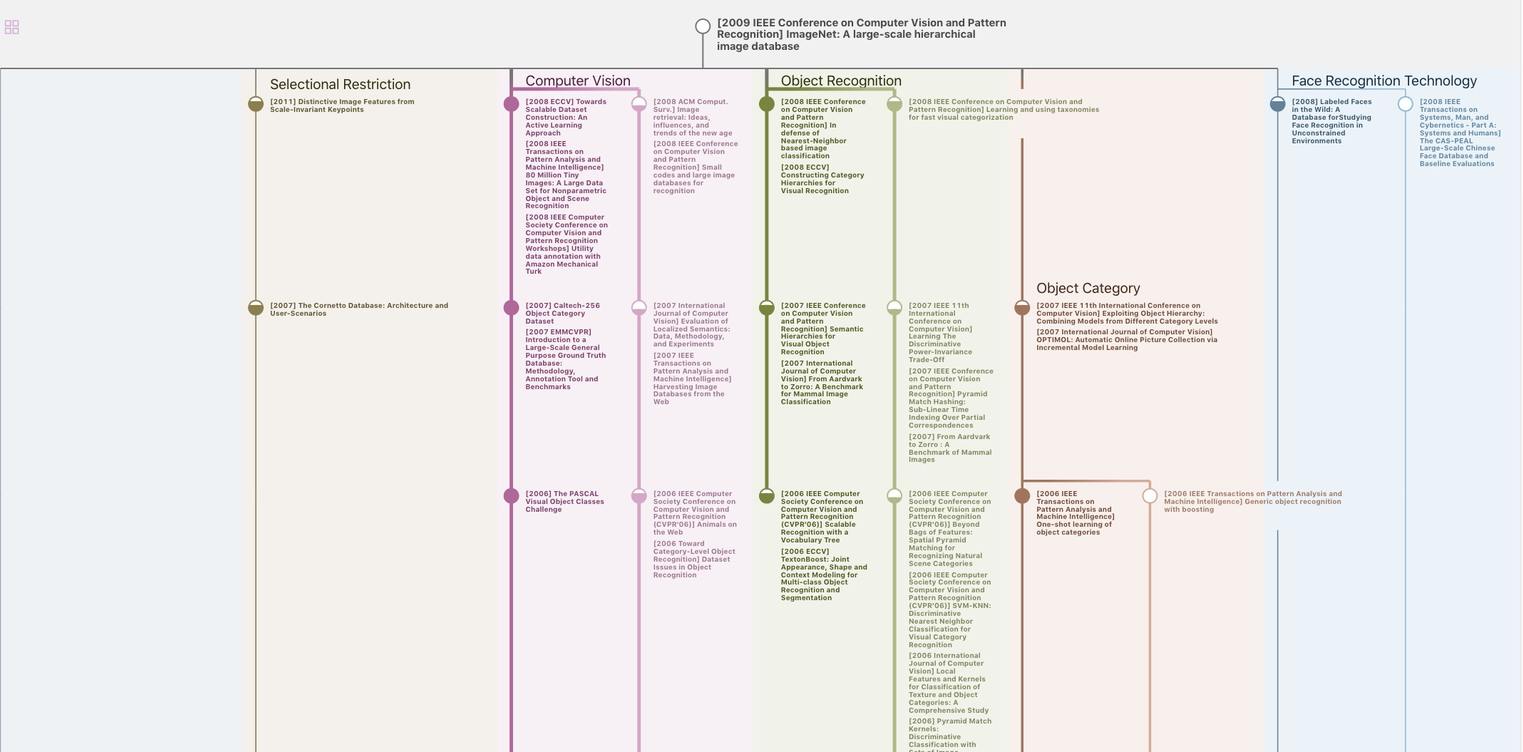
生成溯源树,研究论文发展脉络
Chat Paper
正在生成论文摘要