Bridging the Preference Gap Between Retrievers and LLMs
Annual Meeting of the Association for Computational Linguistics(2024)
University of Illinois at Chicago | University of Michigan
Abstract
Large Language Models (LLMs) have demonstrated superior results across a widerange of tasks, while retrieval has long been established as an effective meansof obtaining task-relevant information for humans. Retrieval-augmentedGeneration (RAG) are known for their effectiveness in knowledge-intensive tasksby locating relevant information and placing it within the context window ofthe LLM. However, the relationship between retrievers and LLMs is stillunder-investigated. Most existing work treats the retriever and the LLM asindependent components and leaves a gap between retrieving human-friendlyinformation and assembling a LLM-friendly context. In this work, we examine anovel bridge model, validate the ranking and selection assumptions inretrievers in the context of RAG, and propose a training framework that chainstogether supervised and reinforcement learning to learn a bridge model.Empirical results demonstrate the effectiveness of our method in bothquestion-answering and personalized generation tasks.
MoreTranslated text
Key words
Reinforcement Learning,Information Retrieval,Natural Language Generation,Language Modeling
PDF
View via Publisher
AI Read Science
Must-Reading Tree
Example
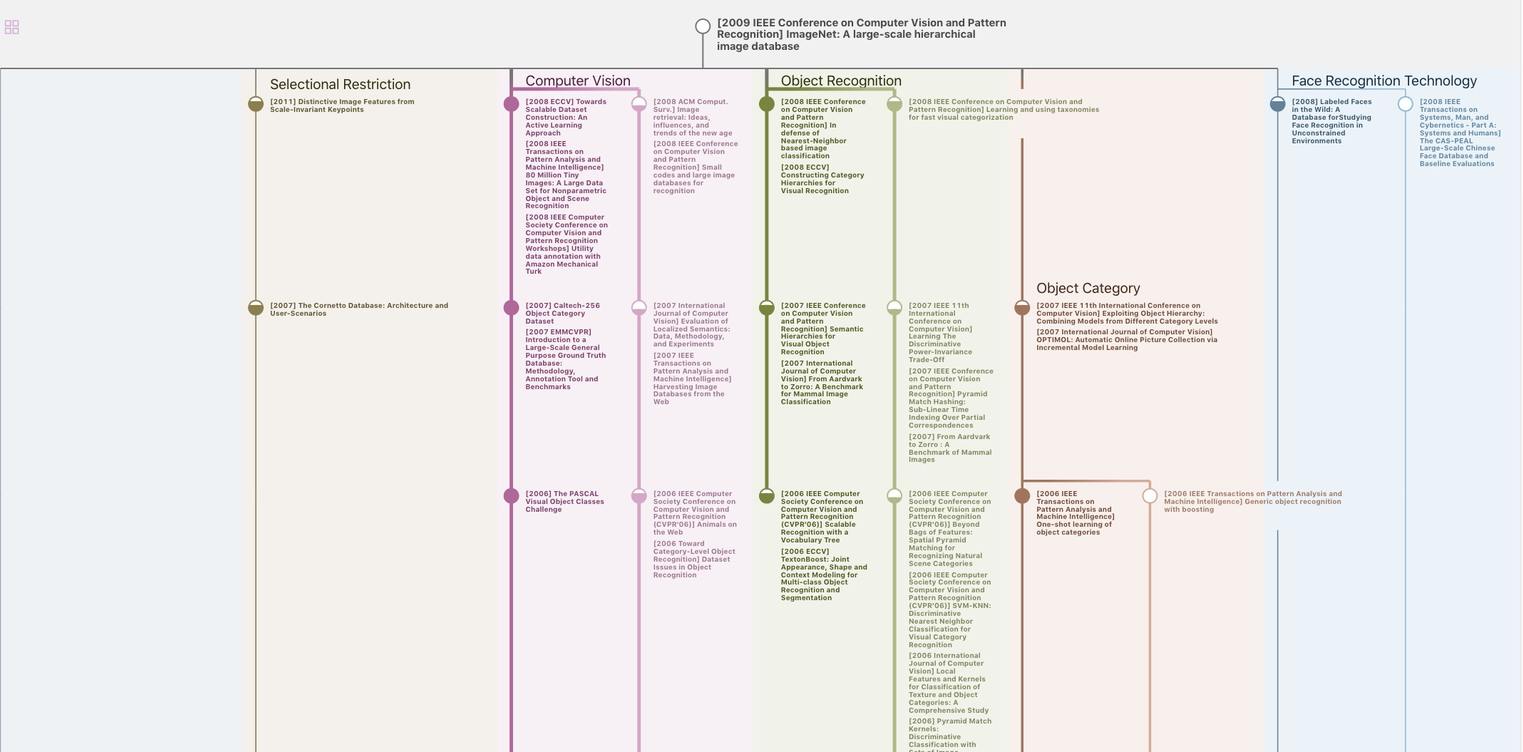
Generate MRT to find the research sequence of this paper
Related Papers
2019
被引用1696 | 浏览
2021
被引用313 | 浏览
Data Disclaimer
The page data are from open Internet sources, cooperative publishers and automatic analysis results through AI technology. We do not make any commitments and guarantees for the validity, accuracy, correctness, reliability, completeness and timeliness of the page data. If you have any questions, please contact us by email: report@aminer.cn
Chat Paper
去 AI 文献库 对话