Quantum Advantage Actor-Critic for Reinforcement Learning
CoRR(2024)
摘要
Quantum computing offers efficient encapsulation of high-dimensional states.In this work, we propose a novel quantum reinforcement learning approach thatcombines the Advantage Actor-Critic algorithm with variational quantum circuitsby substituting parts of the classical components. This approach addressesreinforcement learning's scalability concerns while maintaining highperformance. We empirically test multiple quantum Advantage Actor-Criticconfigurations with the well known Cart Pole environment to evaluate ourapproach in control tasks with continuous state spaces. Our results indicatethat the hybrid strategy of using either a quantum actor or quantum critic withclassical post-processing yields a substantial performance increase compared topure classical and pure quantum variants with similar parameter counts. Theyfurther reveal the limits of current quantum approaches due to the hardwareconstraints of noisy intermediate-scale quantum computers, suggesting furtherresearch to scale hybrid approaches for larger and more complex control tasks.
更多查看译文
AI 理解论文
溯源树
样例
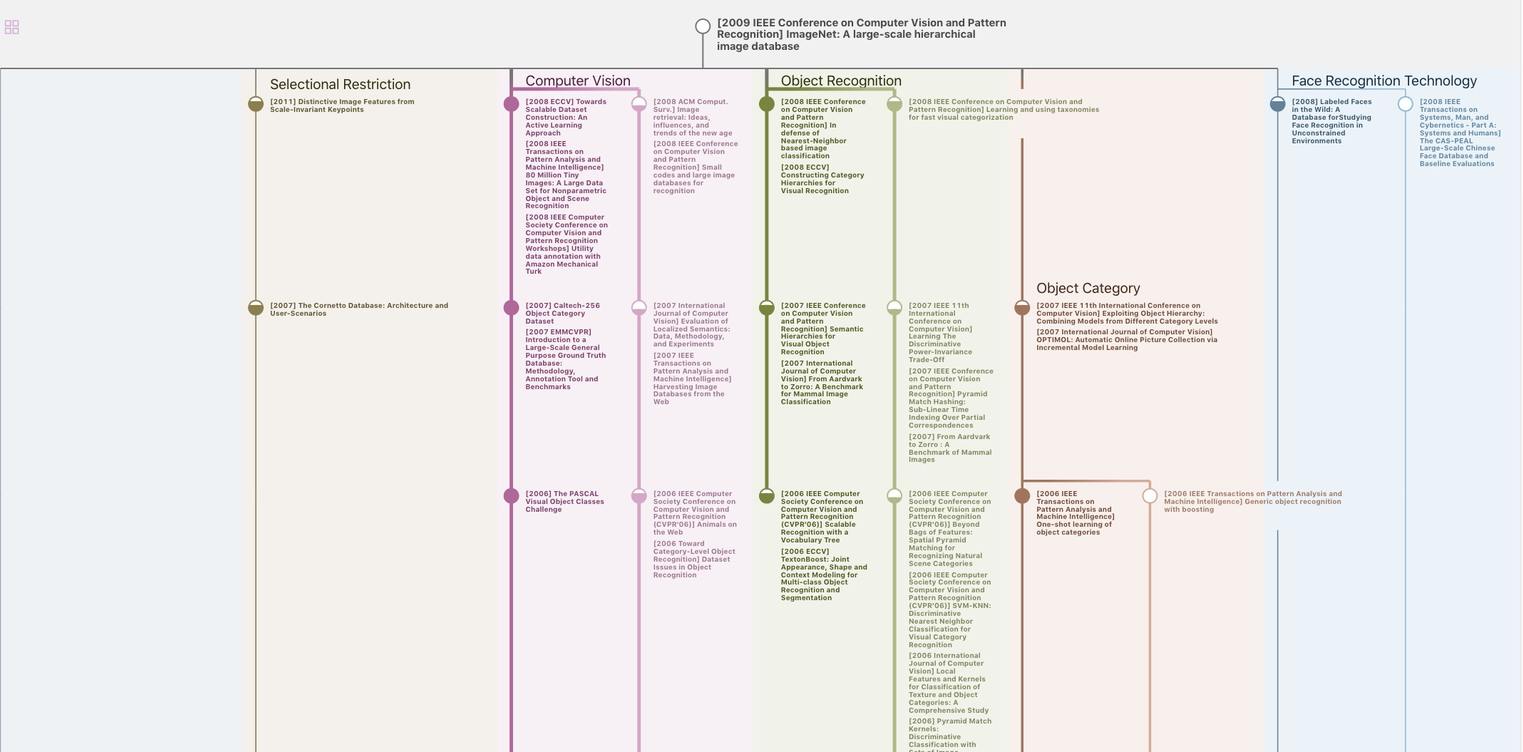
生成溯源树,研究论文发展脉络
Chat Paper
正在生成论文摘要