One for All: Toward Unified Foundation Models for Earth Vision
IGARSS(2024)
Technical University of Munich Chair of Data Science in Earth Observation
Abstract
Foundation models characterized by extensive parameters and trained onlarge-scale datasets have demonstrated remarkable efficacy across variousdownstream tasks for remote sensing data. Current remote sensing foundationmodels typically specialize in a single modality or a specific spatialresolution range, limiting their versatility for downstream datasets. Whilethere have been attempts to develop multi-modal remote sensing foundationmodels, they typically employ separate vision encoders for each modality orspatial resolution, necessitating a switch in backbones contingent upon theinput data. To address this issue, we introduce a simple yet effective method,termed OFA-Net (One-For-All Network): employing a single, shared Transformerbackbone for multiple data modalities with different spatial resolutions. Usingthe masked image modeling mechanism, we pre-train a single Transformer backboneon a curated multi-modal dataset with this simple design. Then the backbonemodel can be used in different downstream tasks, thus forging a path towards aunified foundation backbone model in Earth vision. The proposed method isevaluated on 12 distinct downstream tasks and demonstrates promisingperformance.
MoreTranslated text
Key words
Foundation models,remote sensing,Earth observation,self-supervised learning
PDF
View via Publisher
AI Read Science
Must-Reading Tree
Example
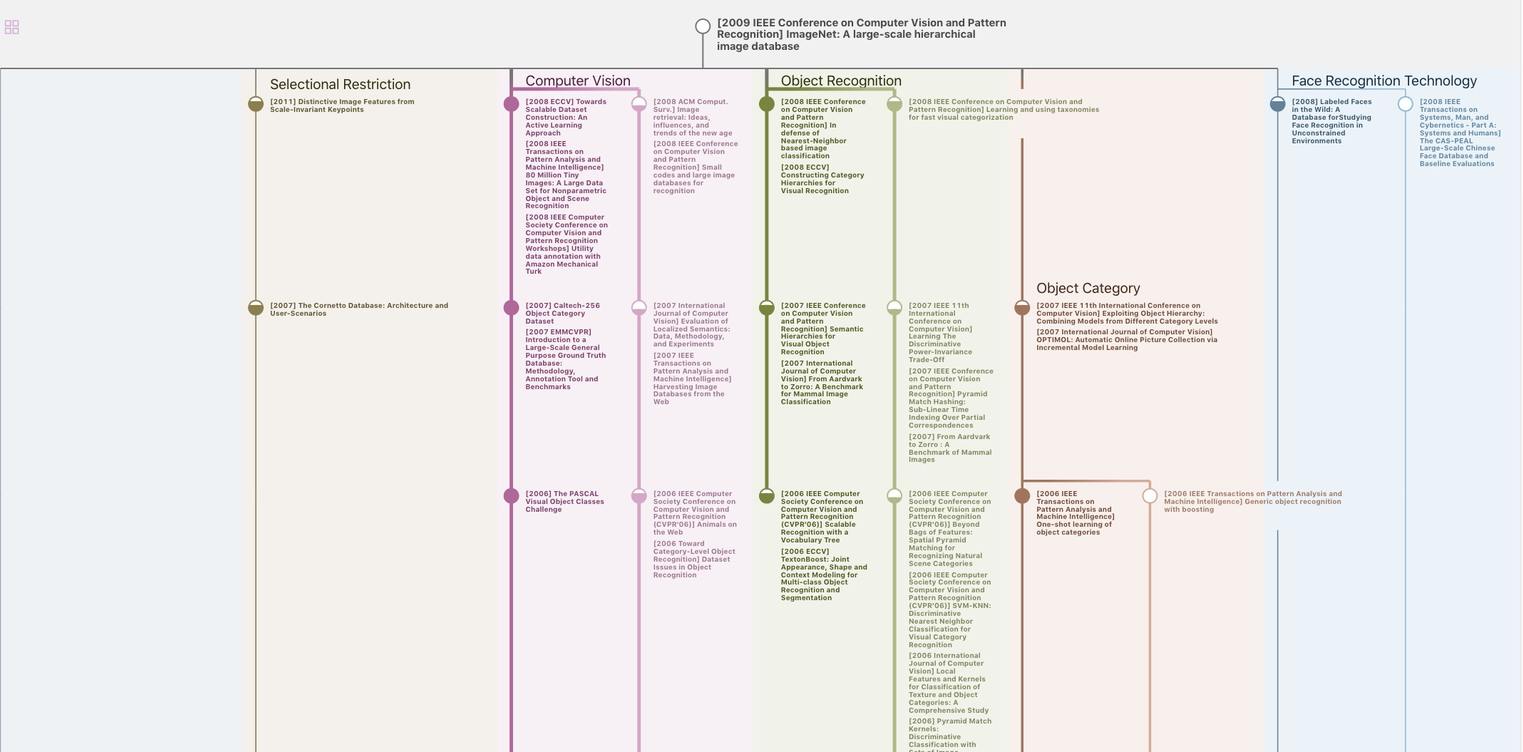
Generate MRT to find the research sequence of this paper
Related Papers
2022
被引用26 | 浏览
2023
被引用4 | 浏览
Data Disclaimer
The page data are from open Internet sources, cooperative publishers and automatic analysis results through AI technology. We do not make any commitments and guarantees for the validity, accuracy, correctness, reliability, completeness and timeliness of the page data. If you have any questions, please contact us by email: report@aminer.cn
Chat Paper
去 AI 文献库 对话