Learning Explainable and Better Performing Representations of POMDP Strategies
CoRR(2024)
摘要
Strategies for partially observable Markov decision processes (POMDP)
typically require memory. One way to represent this memory is via automata. We
present a method to learn an automaton representation of a strategy using the
L*-algorithm. Compared to the tabular representation of a strategy, the
resulting automaton is dramatically smaller and thus also more explainable.
Moreover, in the learning process, our heuristics may even improve the
strategy's performance. In contrast to approaches that synthesize an automaton
directly from the POMDP thereby solving it, our approach is incomparably more
scalable.
更多查看译文
AI 理解论文
溯源树
样例
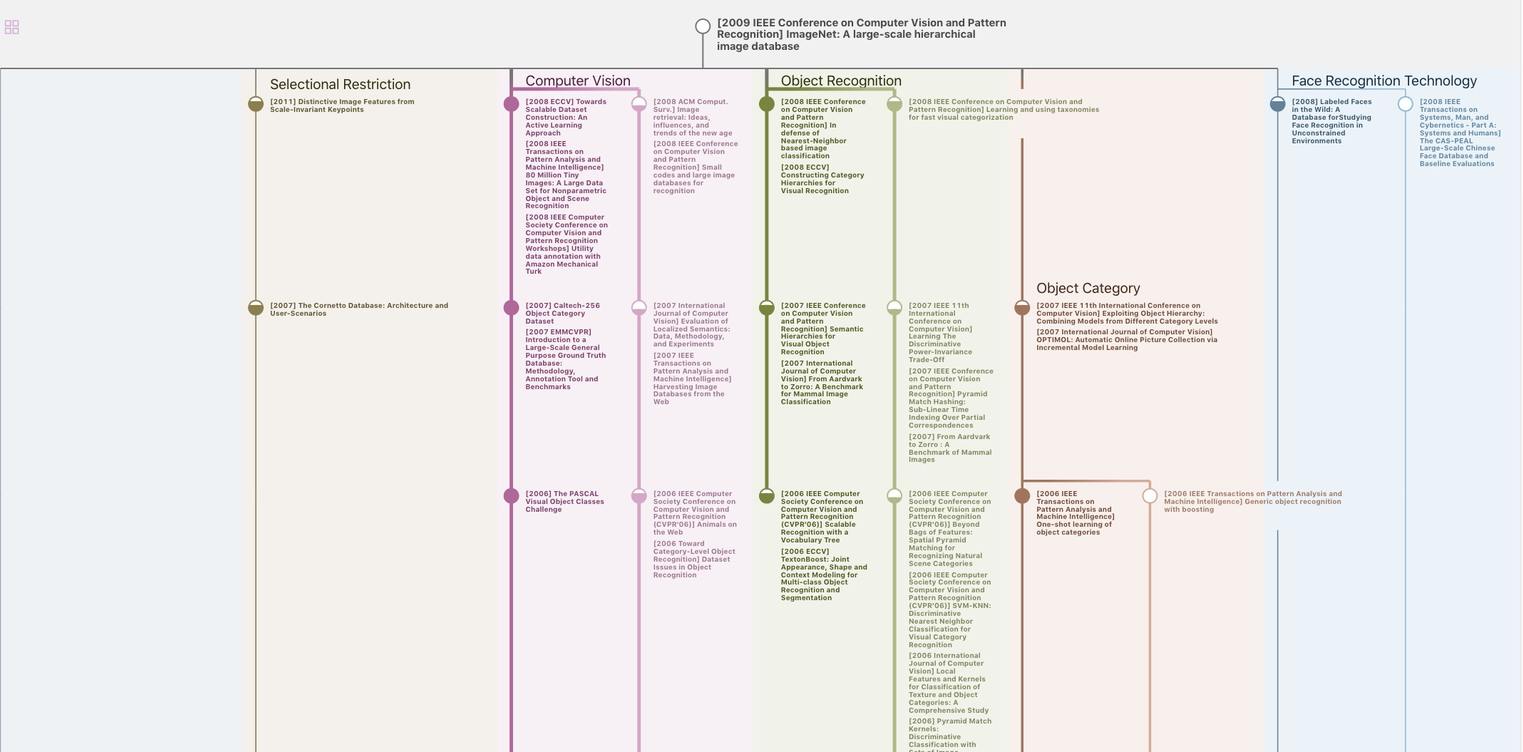
生成溯源树,研究论文发展脉络
Chat Paper
正在生成论文摘要