Explainable Predictive Maintenance: A Survey of Current Methods, Challenges and Opportunities
CoRR(2024)
摘要
Predictive maintenance is a well studied collection of techniques that aims
to prolong the life of a mechanical system by using artificial intelligence and
machine learning to predict the optimal time to perform maintenance. The
methods allow maintainers of systems and hardware to reduce financial and time
costs of upkeep. As these methods are adopted for more serious and potentially
life-threatening applications, the human operators need trust the predictive
system. This attracts the field of Explainable AI (XAI) to introduce
explainability and interpretability into the predictive system. XAI brings
methods to the field of predictive maintenance that can amplify trust in the
users while maintaining well-performing systems. This survey on explainable
predictive maintenance (XPM) discusses and presents the current methods of XAI
as applied to predictive maintenance while following the Preferred Reporting
Items for Systematic Reviews and Meta-Analyses (PRISMA) 2020 guidelines. We
categorize the different XPM methods into groups that follow the XAI
literature. Additionally, we include current challenges and a discussion on
future research directions in XPM.
更多查看译文
关键词
eXplainable Artificial Intelligence,XAI,Predictive Maintenance,Industry 4.0,Industry 5.0,Interpretable Machine Learning,PRISMA
AI 理解论文
溯源树
样例
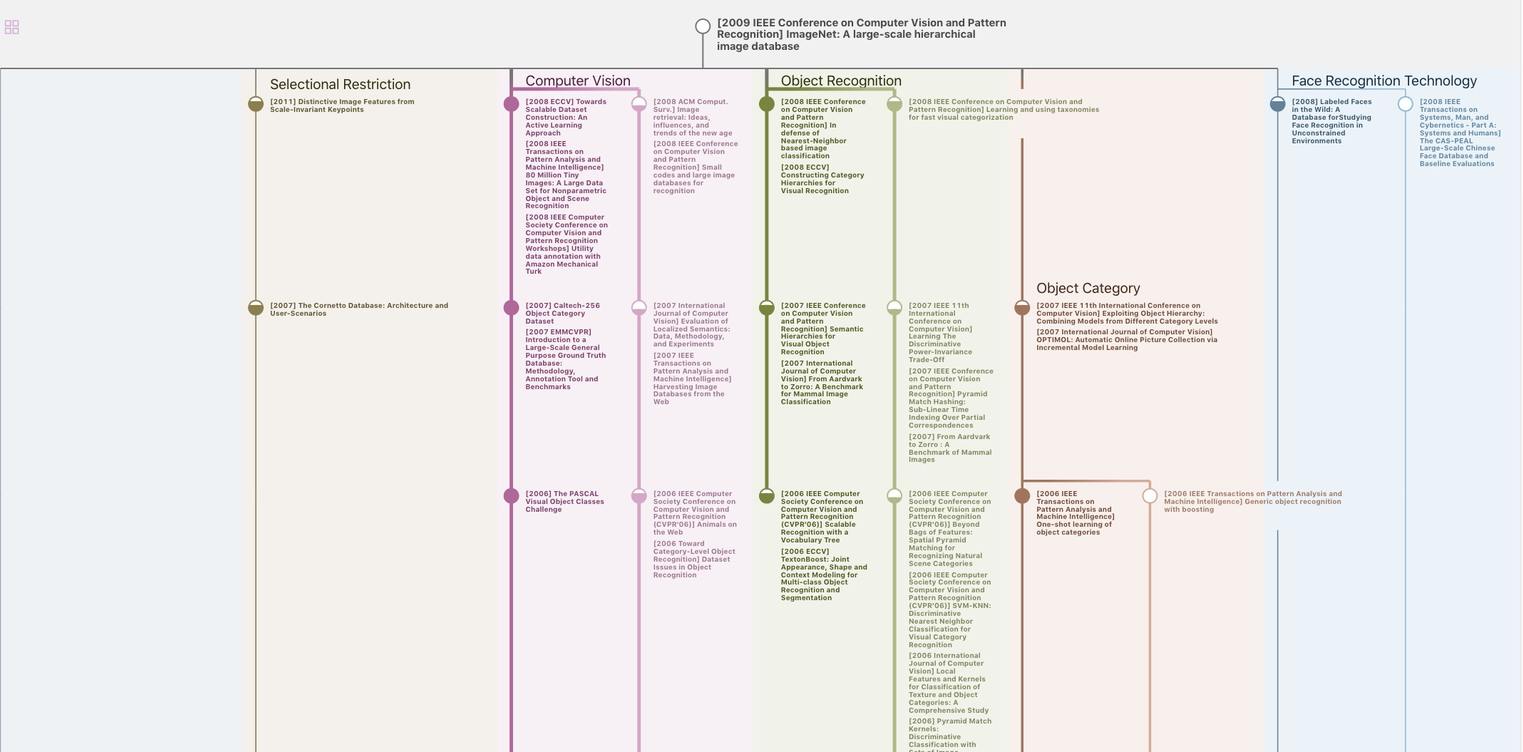
生成溯源树,研究论文发展脉络
Chat Paper
正在生成论文摘要