EmoTalker: Emotionally Editable Talking Face Generation via Diffusion Model
CoRR(2024)
摘要
In recent years, the field of talking faces generation has attracted
considerable attention, with certain methods adept at generating virtual faces
that convincingly imitate human expressions. However, existing methods face
challenges related to limited generalization, particularly when dealing with
challenging identities. Furthermore, methods for editing expressions are often
confined to a singular emotion, failing to adapt to intricate emotions. To
overcome these challenges, this paper proposes EmoTalker, an emotionally
editable portraits animation approach based on the diffusion model. EmoTalker
modifies the denoising process to ensure preservation of the original
portrait's identity during inference. To enhance emotion comprehension from
text input, Emotion Intensity Block is introduced to analyze fine-grained
emotions and strengths derived from prompts. Additionally, a crafted dataset is
harnessed to enhance emotion comprehension within prompts. Experiments show the
effectiveness of EmoTalker in generating high-quality, emotionally customizable
facial expressions.
更多查看译文
关键词
multimodal,talking face generation,diffusion model,intricate emotion
AI 理解论文
溯源树
样例
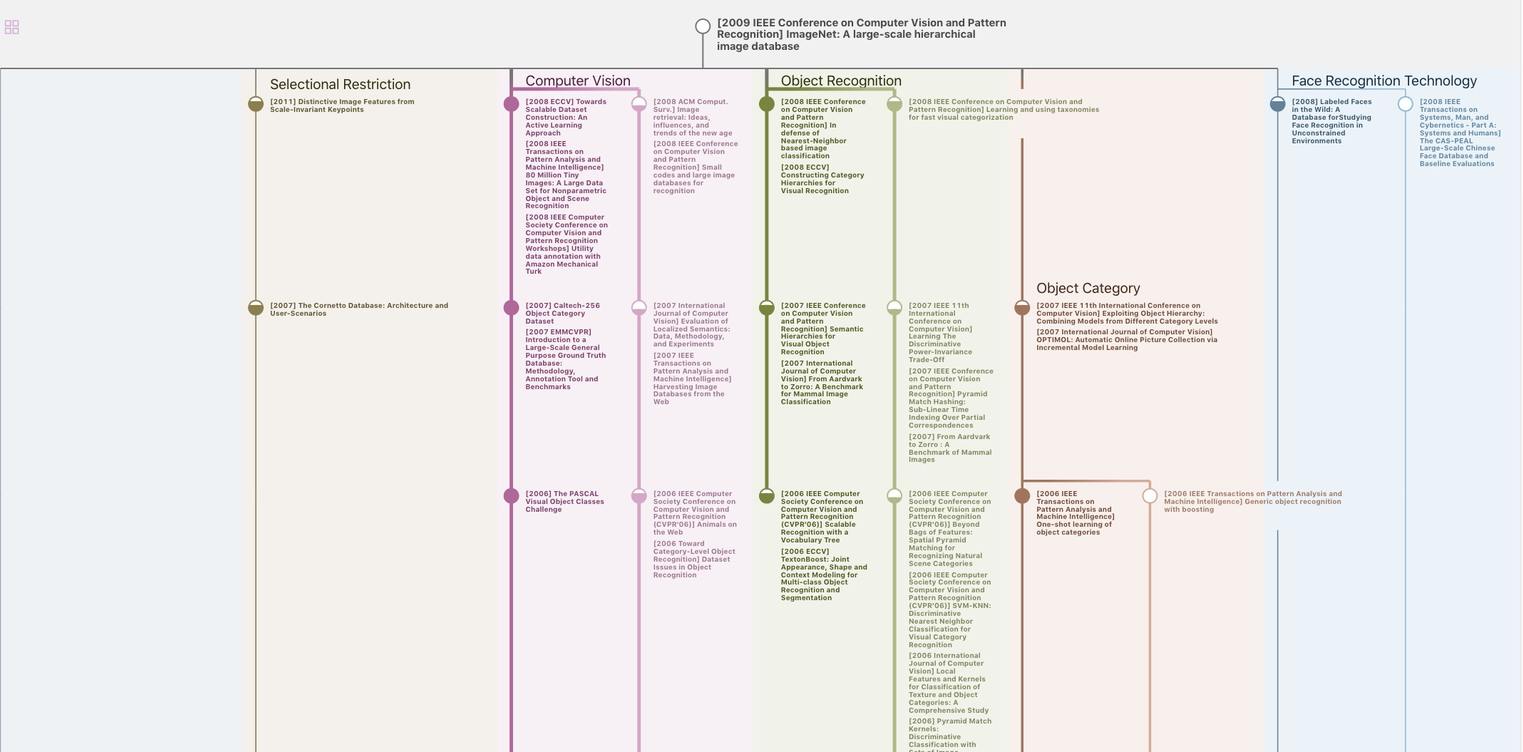
生成溯源树,研究论文发展脉络
Chat Paper
正在生成论文摘要