scikit-matter : A Suite of Generalisable Machine Learning Methods Born out of Chemistry and Materials Science [version 2; peer review: 2 approved, 1 approved with reservations]
Open Research Europe(2023)
摘要
Easy-to-use libraries such as scikit-learn have accelerated the adoption and application of machine learning (ML) workflows and data-driven methods. While many of the algorithms implemented in these libraries originated in specific scientific fields, they have gained in popularity in part because of their generalisability across multiple domains. Over the past two decades, researchers in the chemical and materials science community have put forward general-purpose machine learning methods. The deployment of these methods into workflows of other domains, however, is often burdensome due to the entanglement with domain-specific functionalities. We present the python library scikit-matter that targets domain-agnostic implementations of methods developed in the computational chemical and materials science community, following the scikit-learn API and coding guidelines to promote usability and interoperability with existing workflows.
更多查看译文
关键词
Python,feature selection,sample selection,PCovR,KPCovR,feature reconstruction,eng
AI 理解论文
溯源树
样例
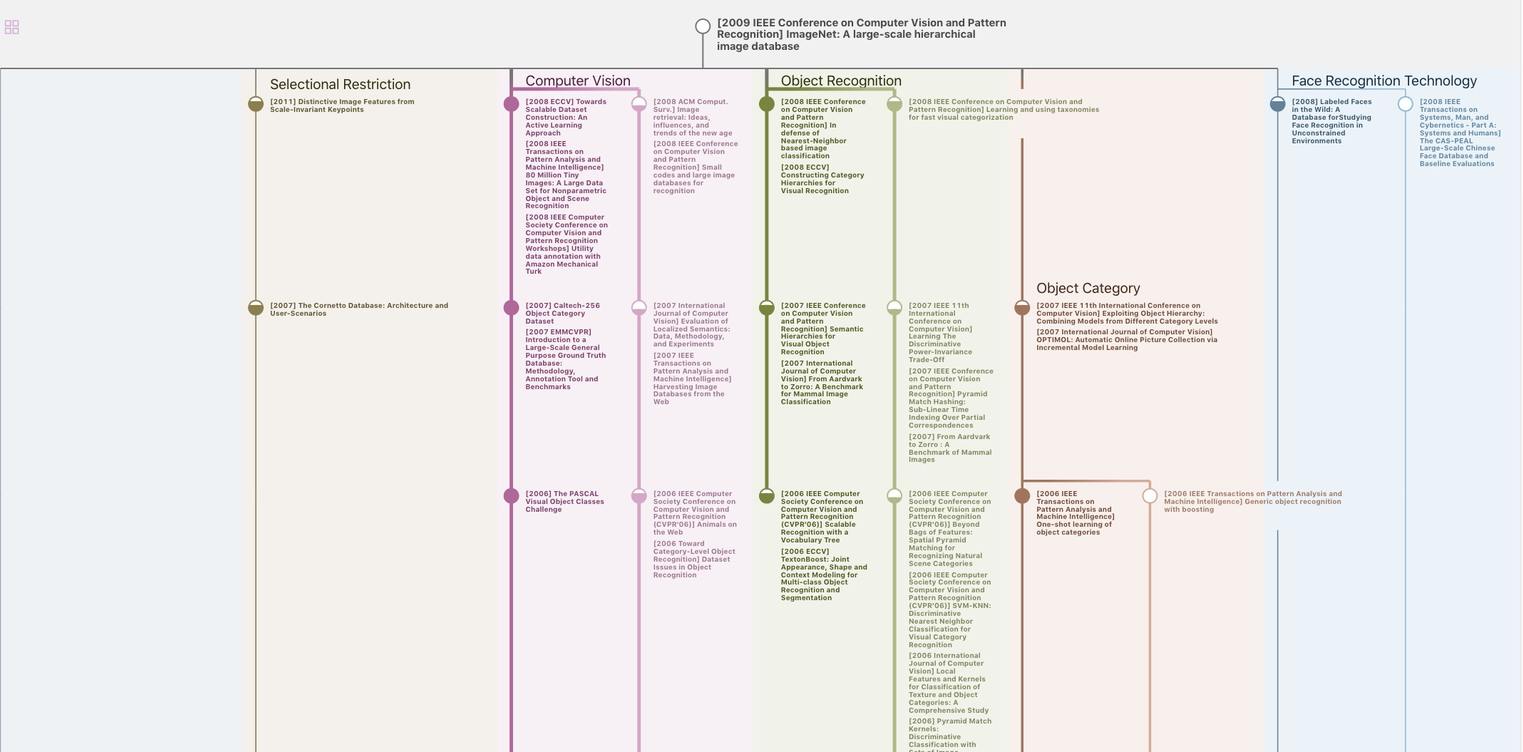
生成溯源树,研究论文发展脉络
Chat Paper
正在生成论文摘要