Statistical inference for pairwise comparison models
arxiv(2024)
摘要
Pairwise comparison models are used for quantitatively evaluating utility and
ranking in various fields. The increasing scale of modern problems underscores
the need to understand statistical inference in these models when the number of
subjects diverges, which is currently lacking in the literature except in a few
special instances. This paper addresses this gap by establishing an asymptotic
normality result for the maximum likelihood estimator in a broad class of
pairwise comparison models. The key idea lies in identifying the Fisher
information matrix as a weighted graph Laplacian matrix which can be studied
via a meticulous spectral analysis. Our findings provide the first unified
theory for performing statistical inference in a wide range of pairwise
comparison models beyond the Bradley–Terry model, benefiting practitioners
with a solid theoretical guarantee for their use. Simulations utilizing
synthetic data are conducted to validate the asymptotic normality result,
followed by a hypothesis test using a tennis competition dataset.
更多查看译文
AI 理解论文
溯源树
样例
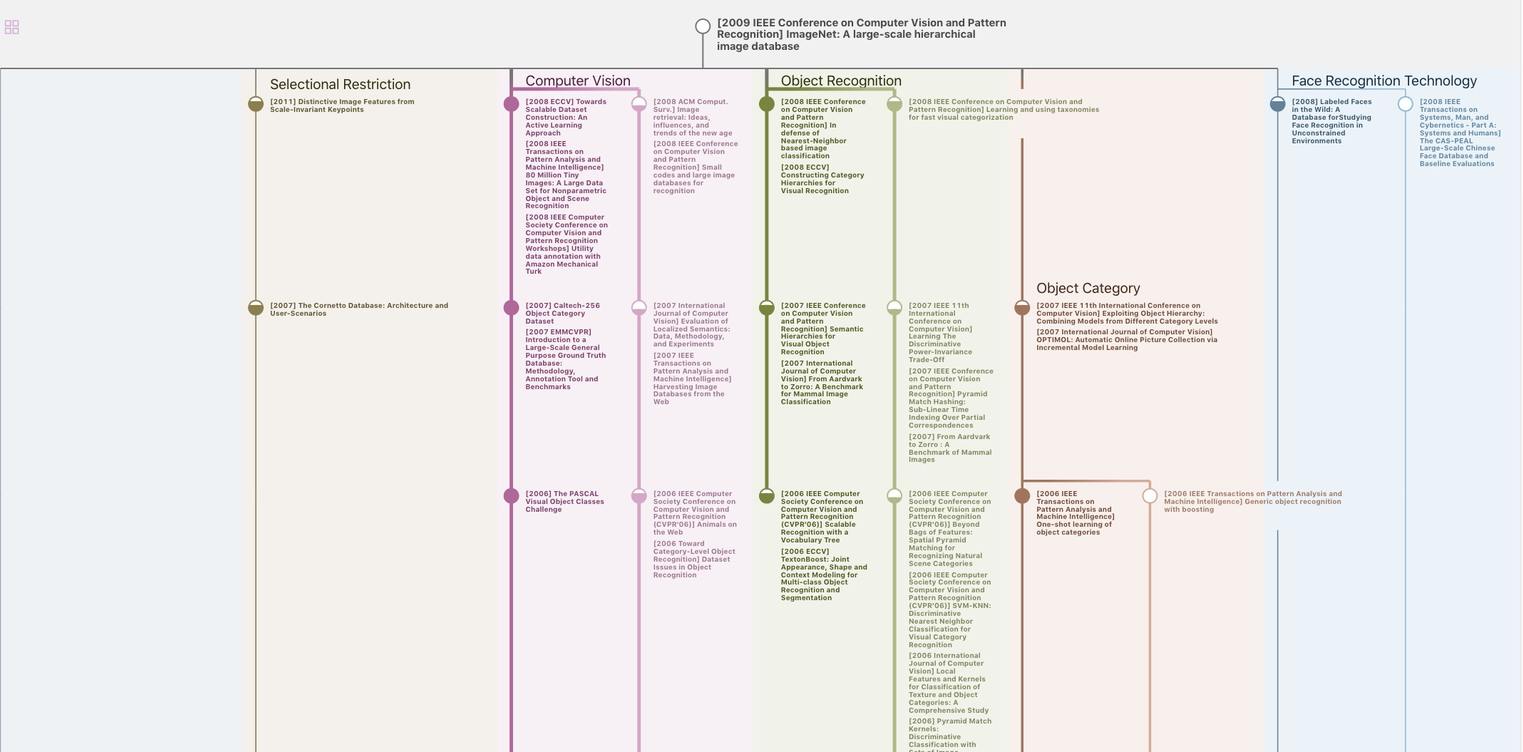
生成溯源树,研究论文发展脉络
Chat Paper
正在生成论文摘要