Mitigating Bias in Machine Learning Models for Phishing Webpage Detection
CoRR(2024)
摘要
The widespread accessibility of the Internet has led to a surge in online
fraudulent activities, underscoring the necessity of shielding users' sensitive
information from cybercriminals. Phishing, a well-known cyberattack, revolves
around the creation of phishing webpages and the dissemination of corresponding
URLs, aiming to deceive users into sharing their sensitive information, often
for identity theft or financial gain. Various techniques are available for
preemptively categorizing zero-day phishing URLs by distilling unique
attributes and constructing predictive models. However, these existing
techniques encounter unresolved issues. This proposal delves into persistent
challenges within phishing detection solutions, particularly concentrated on
the preliminary phase of assembling comprehensive datasets, and proposes a
potential solution in the form of a tool engineered to alleviate bias in ML
models. Such a tool can generate phishing webpages for any given set of
legitimate URLs, infusing randomly selected content and visual-based phishing
features. Furthermore, we contend that the tool holds the potential to assess
the efficacy of existing phishing detection solutions, especially those trained
on confined datasets.
更多查看译文
关键词
Cybersecurity,Phishing,Machine Learning,Deep Learning
AI 理解论文
溯源树
样例
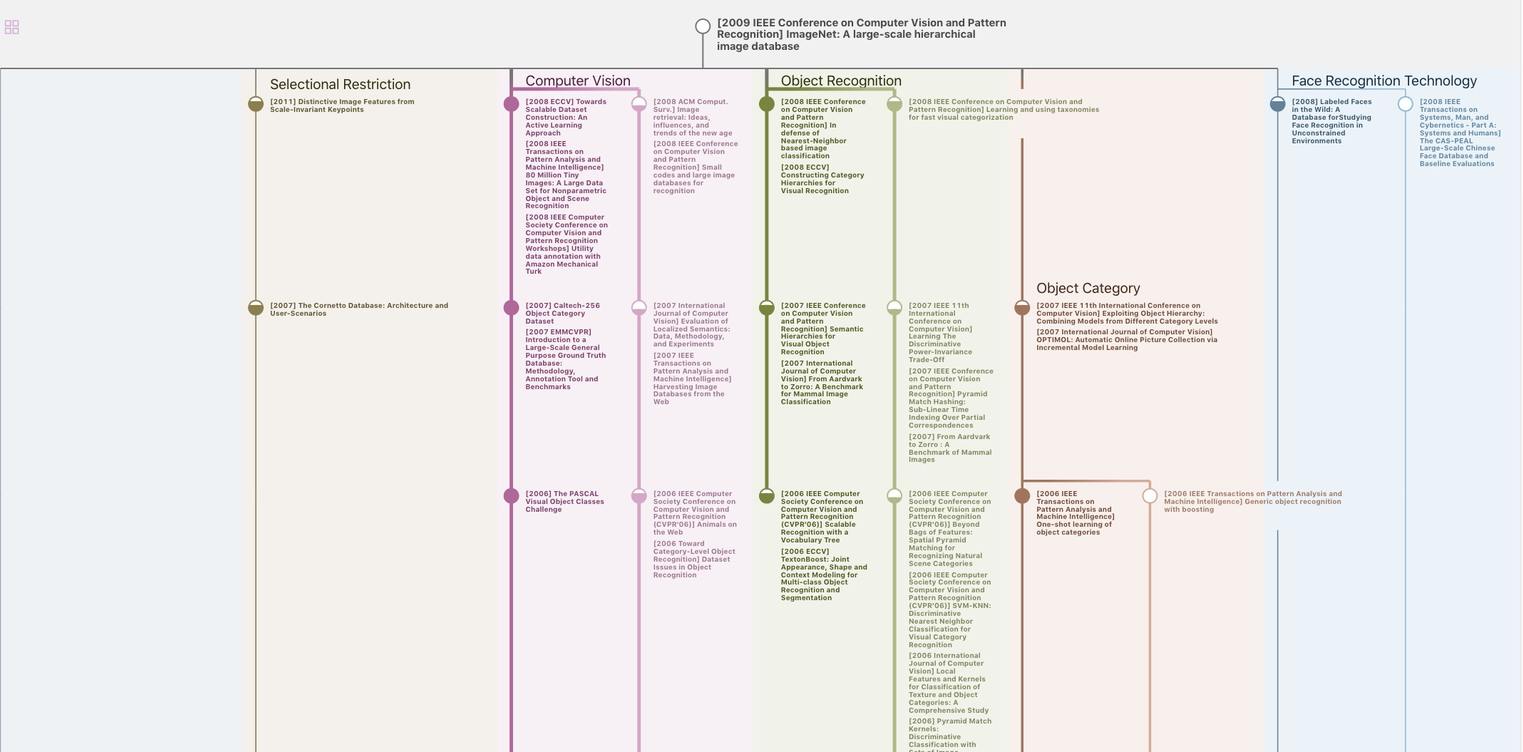
生成溯源树,研究论文发展脉络
Chat Paper
正在生成论文摘要