Learning scale-aware relationships via Laplacian decomposition-based transformer for 3D human pose estimation
Multimedia Systems(2024)
摘要
This paper presents a parameter-free method for 3D human pose estimation via the Laplacian decomposition-based transformer. The non-local interactions between 3D mesh vertices of the whole body are effectively estimated in transformer-based approaches while the graph model also has begun to be embedded into the transformer for consideration of neighborhood interactions in the kinematic topology. Even though such combination has shown the remarkable progress in 3D human pose estimation, scale-aware relationships between body parts are not sufficiently explored in literature. To supplement this point, we propose to apply the Laplacian pyramid module to the transformer, which decomposes encoded features into Laplacian residuals of different scale spaces. Furthermore, we separately compute self-attentions according to body parts for generating more natural human poses. Experimental results on benchmark datasets show that the proposed method successfully improves the performance of 3D human pose estimation. The code and model are publicly available at: https://github.com/DCVL-3D/Laphormer_release .
更多查看译文
关键词
3D human pose estimation,Laplacian pyramid module,Scale-aware manner,Within-part relationship
AI 理解论文
溯源树
样例
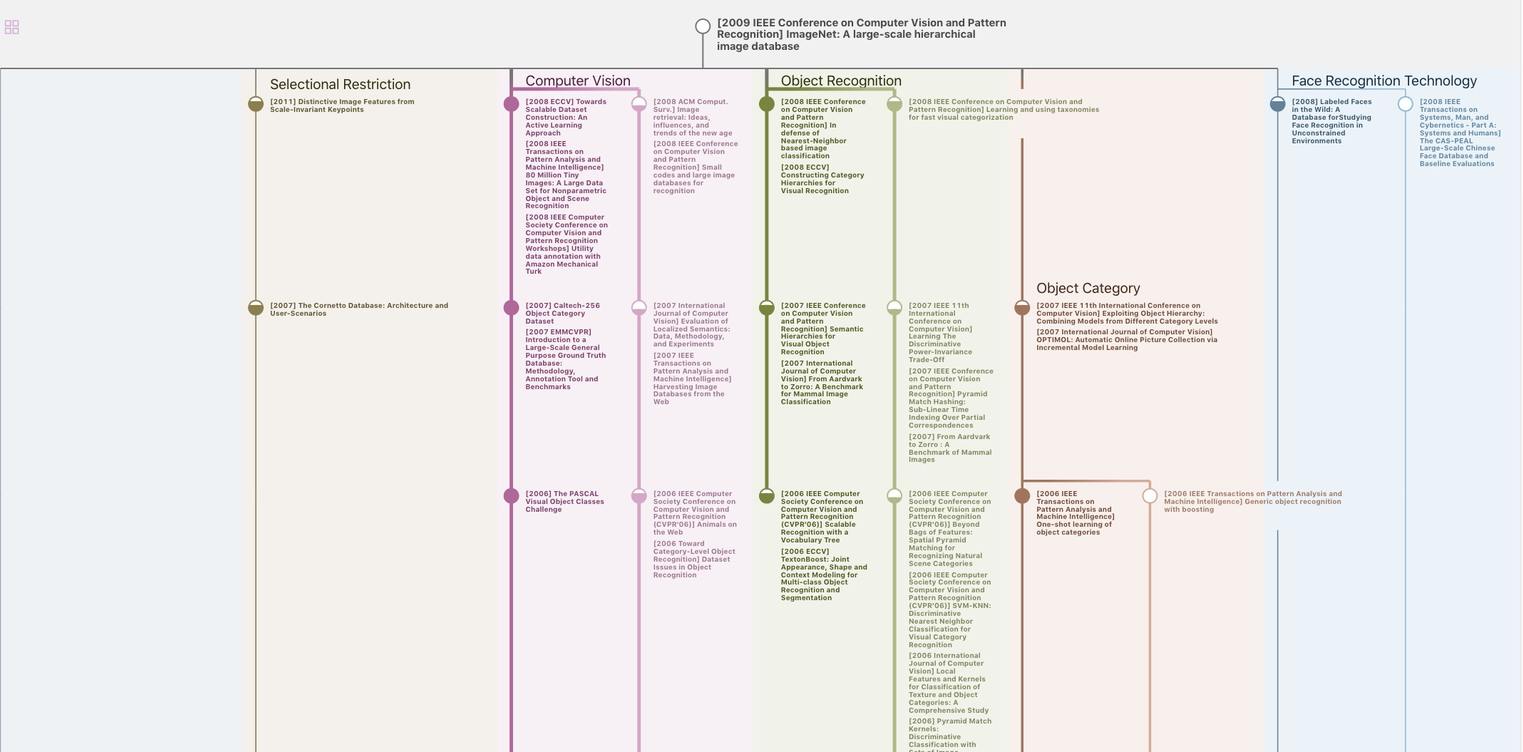
生成溯源树,研究论文发展脉络
Chat Paper
正在生成论文摘要