Flexible RAN Slicing in Open RAN With Constrained Multi-Agent Reinforcement Learning.
IEEE J. Sel. Areas Commun.(2024)
摘要
Network slicing enables the provision of customized services in next-generation mobile networks. Accordingly, the network is divided into logically isolated networks that share underlying resources but are tailored to meet the distinct service requirements of their users. However, allocating the minimum necessary resources to satisfy slices’ requirements is challenging, particularly when the number of slices is variable or too large which is envisioned in Open RAN. State-of-the-art proposals leverage reinforcement learning (RL) algorithms; however, they suffer from over-provisioning and/or frequent violations of service-level agreement (SLA) due to the large and changing state and action spaces. This paper introduces a novel cooperative multi-agent RL algorithm for RAN slicing in Open RAN, designed to adapt to variable slice numbers and effectively scale as they grow. To train this model, we exploit a novel constrained RL algorithm that explicitly considers SLA constraints to maintain a decreasing SLA violation ratio during training. Our approach is compatible with the Open RAN architecture, allowing for feasible deployment in future mobile networks. CMARS surpasses RL methods in SLA satisfaction by 50% in large-scale slicing, using only 9% more resources. It has 8% fewer SLA violations and 19% lower resource consumption for a flexible number of slices.
更多查看译文
关键词
5G and beyond mobile networks,open RAN,radio resource management,network slicing,reinforcement learning
AI 理解论文
溯源树
样例
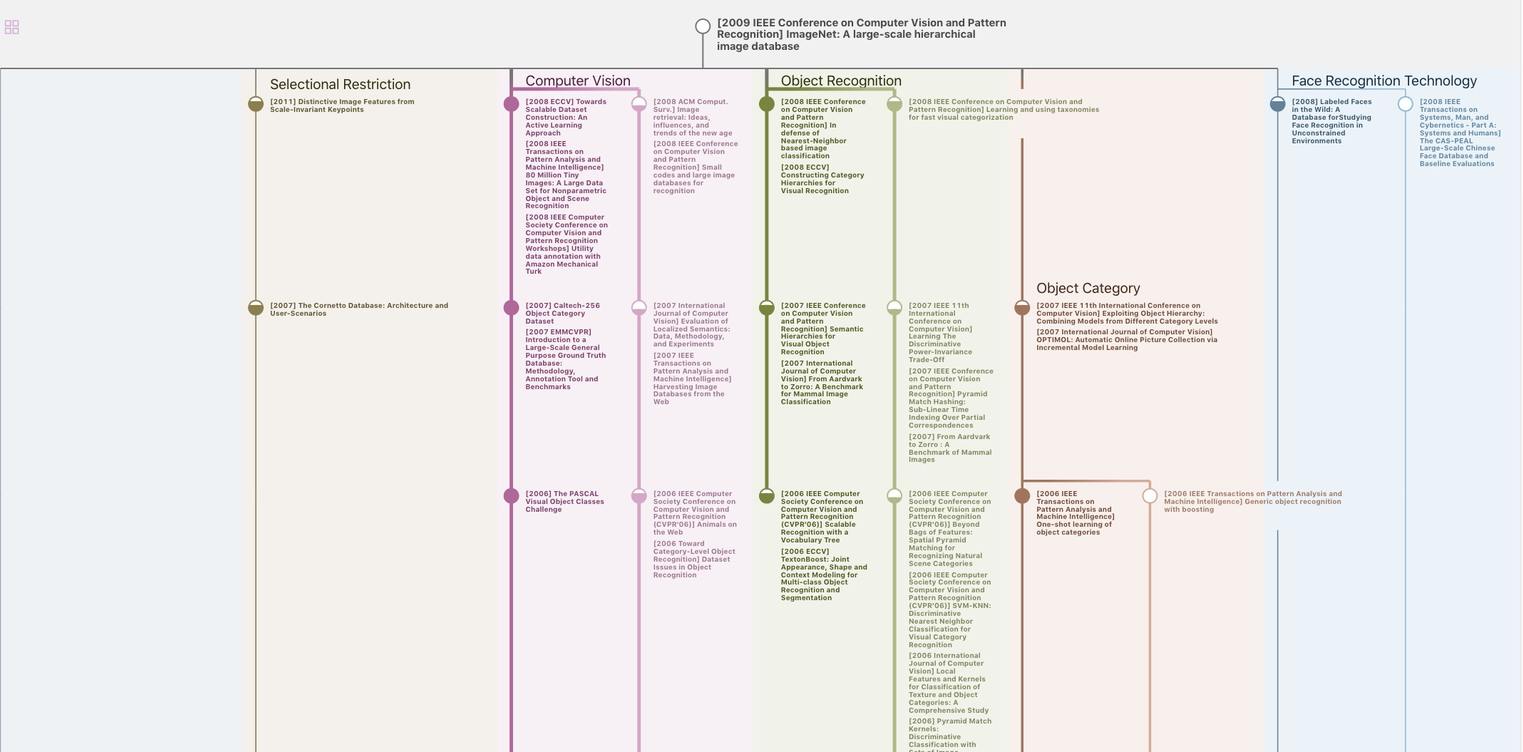
生成溯源树,研究论文发展脉络
Chat Paper
正在生成论文摘要