On the Variability of Statistical Models
2023 Fifth International Conference on Transdisciplinary AI (TransAI)(2023)
摘要
The axiom that a good model strikes a good balance between bias and variance may be regarded as a corollary of the principle of parsimony or Occam's Razor. In this context, the bias is measured in training cost, while the variance of, say, a regression model is measured by the cost associated with a validation set. If reducing bias is the goal, one will strive to fetch as complex a model as necessary. Still, complexity is invariably coupled with an increase in variance: greater complexity implies greater variance. In practice, driving training cost to near zero does not pose a fundamental problem; in fact, a sufficiently complex decision tree can drive training cost to zero; however, the problem is controlling the model's variance. We investigate various regression model frameworks, including generalized linear models, Cox proportional hazard models, ARMA, and illustrate how misspecifying a model with ‘excessive’ complexity affects the variance.
更多查看译文
关键词
Statistical Models,Regression Models,Variance-Bias Tradeoff,Model Misspecification,Cauchy Eigenvalues Interlacing Theorem,Spectral Radius of a Matrix,Principle of parsimony,Cox PH Regression,ARMA
AI 理解论文
溯源树
样例
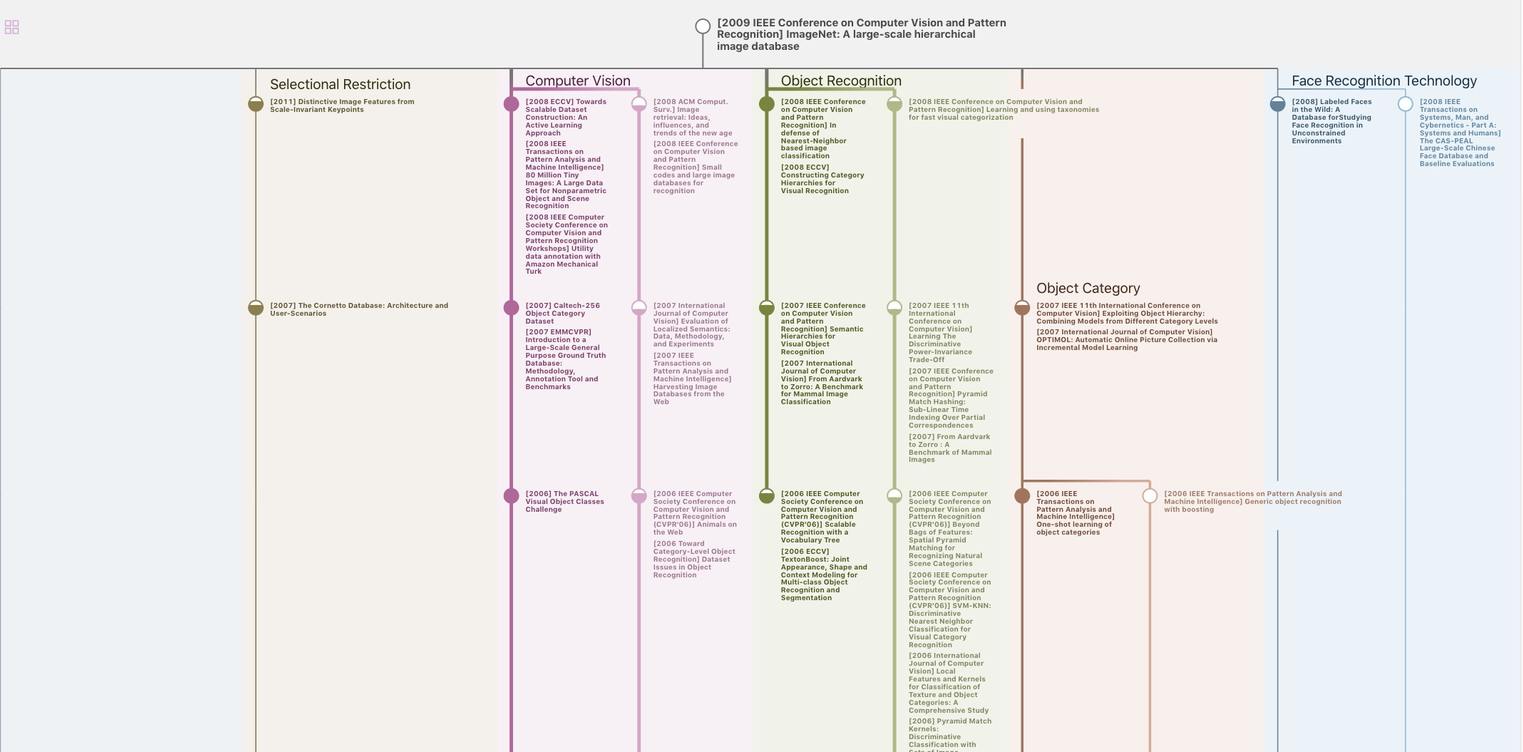
生成溯源树,研究论文发展脉络
Chat Paper
正在生成论文摘要