Machine Learning Approach to Investigating the Relative Importance of Meteorological and Aerosol-Related Parameters in Determining Cloud Microphysical Properties
Tellus: Series B, Chemical and Physical Meteorology(2024)
摘要
Aerosol effects on cloud properties are notoriously difficult to disentangle from variations driven by meteorological factors. Here, a machine learning model is trained on reanalysis data and satellite retrievals to predict cloud microphysical properties, as a way to illustrate the relative importance of meteorology and aerosol, respectively, on cloud properties. It is found that cloud droplet effective radius can be predicted with some skill from only meteorological information, including estimated air mass origin and cloud top height. For ten geographical regions the mean coefficient of determination is 0.41 and normalised root-mean square error 24%. The machine learning model thereby performs better than a reference linear regression model, and a model predicting the climatological mean. A gradient boosting regression performs on par with a neural network regression model. Adding aerosol information as input to the model improves its skill somewhat, but the difference is small and the direction of the influence of changing aerosol burden on cloud droplet effective radius is not consistent across regions, and thereby also not always consistent with what is expected from cloud brightening.
更多查看译文
关键词
aerosol-cloud interaction,cloud brightening,machine learning,gradient boosting regression,reanalysis,remote sensing
AI 理解论文
溯源树
样例
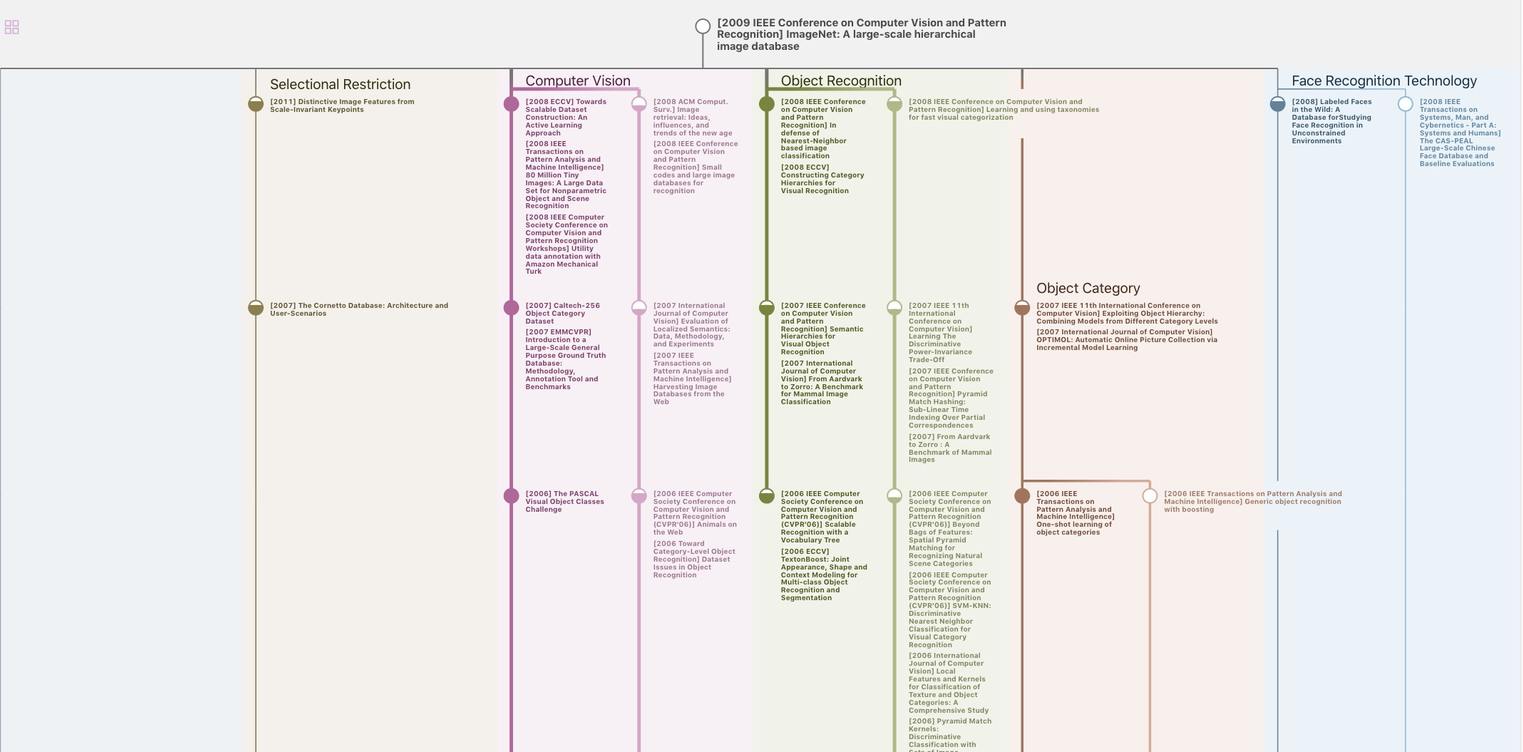
生成溯源树,研究论文发展脉络
Chat Paper
正在生成论文摘要