Semi-supervised deep learning for lithium-ion battery state-of-health estimation using dynamic discharge profiles
CELL REPORTS PHYSICAL SCIENCE(2024)
摘要
Data-driven methods for lithium-ion battery state-of-health (SoH) estimation gain attention for their ability to avoid acquiring prior battery mechanism knowledge. However, most existing methods require massive labeled data, unsuitable for dynamic conditions in the real world. In this study, extracting features from battery dynamic discharge profiles with a small amount of regularly calibrated data (1.5%-15% labeled) is used for capacity estimation. A semi -supervised deep-learning method based on bidirectional gate recurrent unit (biGRU) and structured kernel interpolation (SKI) Gaussian process regression (GPR) is proposed by employing three features: current rate, pseudo-differential voltage, and temperature. The capacity estimation error of a NASA randomized battery usage dataset is below 1.91% in root-mean-square percentage error (RMSPE). The proposed method is verified on three different random discharge datasets with RMSPE from 2.49% to 3.24%. It provides the feasibility of using dynamic data on battery SoH estimation in electric vehicle applications.
更多查看译文
AI 理解论文
溯源树
样例
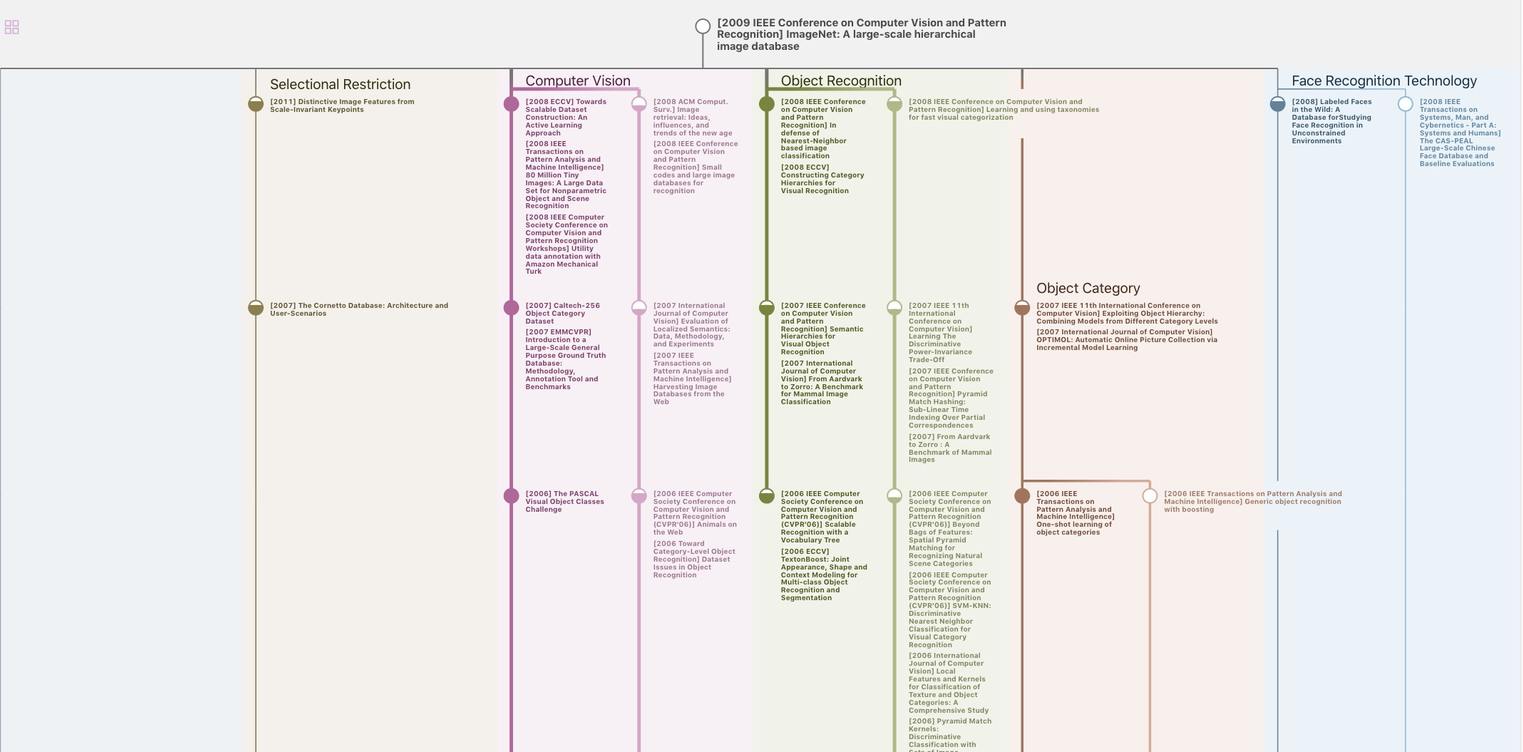
生成溯源树,研究论文发展脉络
Chat Paper
正在生成论文摘要